Invited Paper: Hyperdimensional Computing for Resilient Edge Learning
2023 IEEE/ACM INTERNATIONAL CONFERENCE ON COMPUTER AIDED DESIGN, ICCAD(2023)
摘要
Recent strides in deep learning have yielded impressive practical applications such as autonomous driving, natural language processing, and graph reasoning. However, the susceptibility of deep learning models to subtle input variations, which stems from device imperfections and non-idealities, or adversarial attacks on edge devices, presents a critical challenge. These vulnerabilities hold dual significance-security concerns in critical applications and insights into human-machine sensory alignment. Efforts to enhance model robustness encounter resource constraints in the edge and the black box nature of neural networks, hindering their deployment on edge devices. This paper focuses on algorithmic adaptations inspired by the human brain to address these challenges. Hyper Dimensional Computing (HDC), rooted in neural principles, replicates brain functions while enabling efficient, noise-tolerant computation. HDC leverages high-dimensional vectors to encode information, seamlessly blending learning and memory functions. Its transparency empowers practitioners, enhancing both robustness and understanding of deployed models. In this paper, we introduce the first comprehensive study that compares the robustness of HDC to white-box malicious attacks to that of deep neural network (DNN) models and the first HDC gradient-based attack in the literature. We develop a framework that enables HDC models to generate gradient-based adversarial examples using state-of-the-art techniques applied to DNNs. Our evaluation shows that our HDC model provides, on average, 19.9% higher robustness than DNNs to adversarial samples and up to 90% robustness improvement against random noise on the weights of the model compared to the DNN.
更多查看译文
AI 理解论文
溯源树
样例
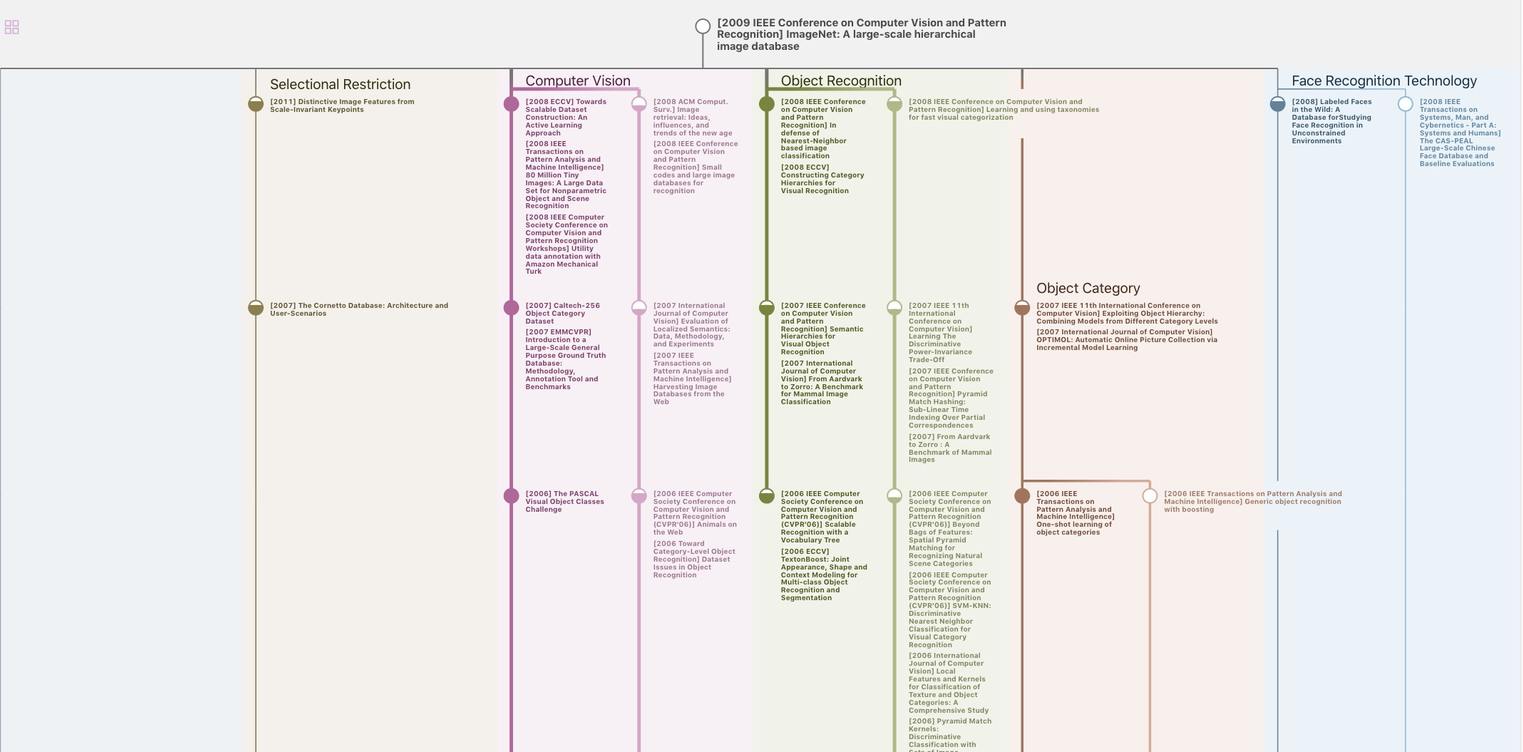
生成溯源树,研究论文发展脉络
Chat Paper
正在生成论文摘要