A method for data imbalance in defective solar cell detection in Electroluminescence images based on experimental evaluation
2023 35TH CHINESE CONTROL AND DECISION CONFERENCE, CCDC(2023)
摘要
\The large number of PV(PV) modules put into practical use make automatic defect detection increasingly important in the PV field. Since the number of solar cells in PV module containing defects is relatively small, there is a big problem that the positive and negative samples are imbalanced during the collection of solar cell image data. There have been few works considering class imbalance problem on solar cell defect detection. The purpose of this paper is to explore the practical effectiveness of methods for the class imbalance problem on a dataset of EL images. A series of methods from both data-level and algorithm-level are carried out on the EL image dataset. By comparing and analyzing the practical performances of different methods, we hope to help researchers to better solve the data imbalance problem on defective solar cell detection. We find that resampling and image enhancement in data-level methods, pre-training, appropriate model size, and thresholding operations in algorithm-level methods can improve the performance of defect detection in class imbalanced situations. The parameter alpha of Focal loss can improve the performance of the model, while gamma makes the performance of the model worse. We also reviewed the evaluation metrics which are suitable for the class imbalance problem. Finally, we synthesized these methods to give a solution for defective solar cell detection under dataset imbalance.
更多查看译文
关键词
Photovoltaic (PV) modules,Defect classification,Electroluminescence,Deep learning,Convolutional neural network
AI 理解论文
溯源树
样例
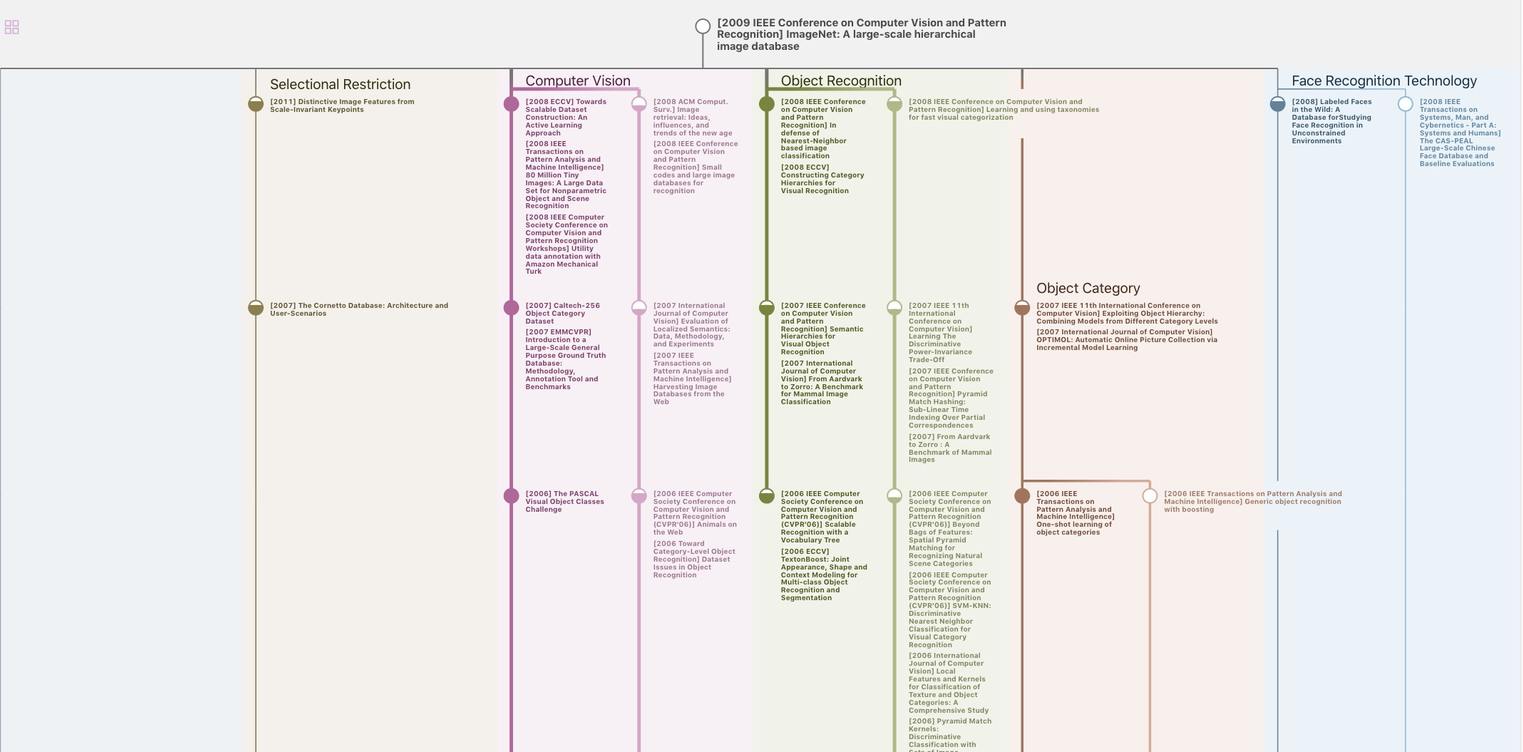
生成溯源树,研究论文发展脉络
Chat Paper
正在生成论文摘要