Efficient combined algorithm of Transformer and U-Net for 3D medical image segmentation
2023 35TH CHINESE CONTROL AND DECISION CONFERENCE, CCDC(2023)
摘要
In recent years, using convolutional neural network (CNN) to segment medical images has achieved good results already. The CNN model with U-shaped structure of encoder and decoder and jump connection mechanism has been widely used in various medical tasks. However, CNN is unable to learn global information and conduct remote semantic information interaction due to its inductive bias. With the application of Transformer in Computer Vision field, the global remote dependency modeling capability brought by Transformer can make up for the locality of CNN to some extent. However, in recent studies, most proposed methods only use Transformer or combinations of Transformer and CNN at both encoder and decoder. In this paper, we propose a new U-shaped network framework with incomplete symmetry, using Transformer module for image feature extraction, and using CNN for image recovery, to seek more possibilities for the combination of Transformer and CNN in the medical segmentation field. Experiments on cardiac MRI segmentation ACDC data sets show that giving up Transformer in the decoding layer will not reduce the overall segmentation performance, and half-transformer and half-CNN network structure can give a good balance between computation and segmentation performance.
更多查看译文
关键词
Transformer,CNN,Medical segmentation
AI 理解论文
溯源树
样例
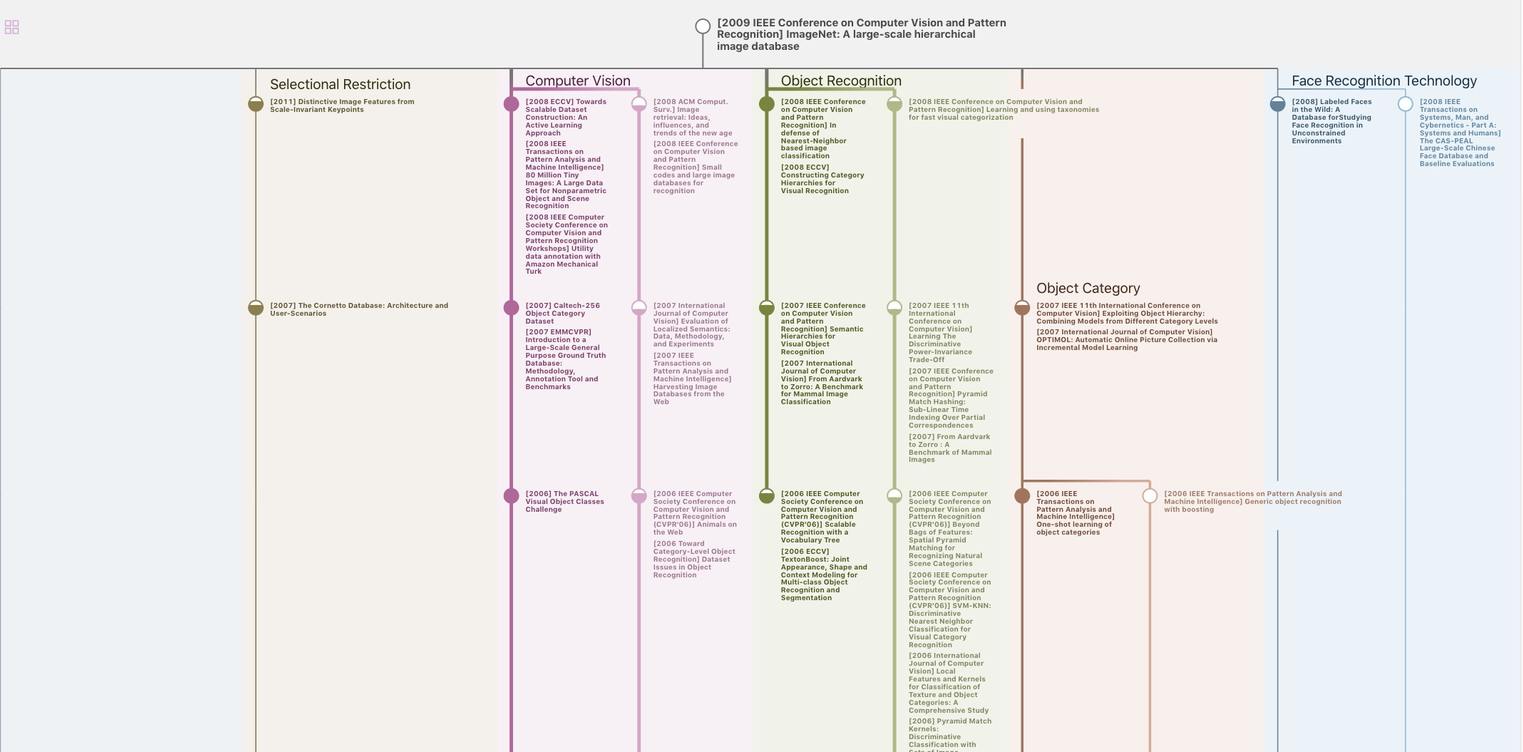
生成溯源树,研究论文发展脉络
Chat Paper
正在生成论文摘要