Deep Reinforcement Learning for Continuous Control of Material Thickness
ARTIFICIAL INTELLIGENCE XL, AI 2023(2023)
摘要
To achieve the desired quality standards of certain manufactured materials, the involved parameters are still adjusted by knowledge-based procedures according to human expertise, which can be costly and time-consuming. To optimize operational efficiency and provide decision support for human experts, we develop a general continuous control framework that utilizes deep reinforcement learning (DRL) to automatically determine the main control parameters, in situations where simulation environments are unavailable and traditional PID controllers are not viable options. In our work, we aim to automatically learn the key control parameters to achieve the desired outlet thickness of the manufactured material. We first construct a surrogate environment based on real-world expert trajectories obtained from the true underlining manufacturing process to achieve this. Subsequently, we train a DRL agent within the surrogate environment. Our results suggest a Proximal Policy Optimization (PPO) algorithm combined with a Multi-Layer Perceptron (MLP) surrogate environment to successfully learn a policy that continuously changes parameter configurations optimally, achieving the desired target material thickness within an acceptable range.
更多查看译文
关键词
Reinforcement Leaning,Deep Learning,Real World Manufacturing,Intelligent Decision Support
AI 理解论文
溯源树
样例
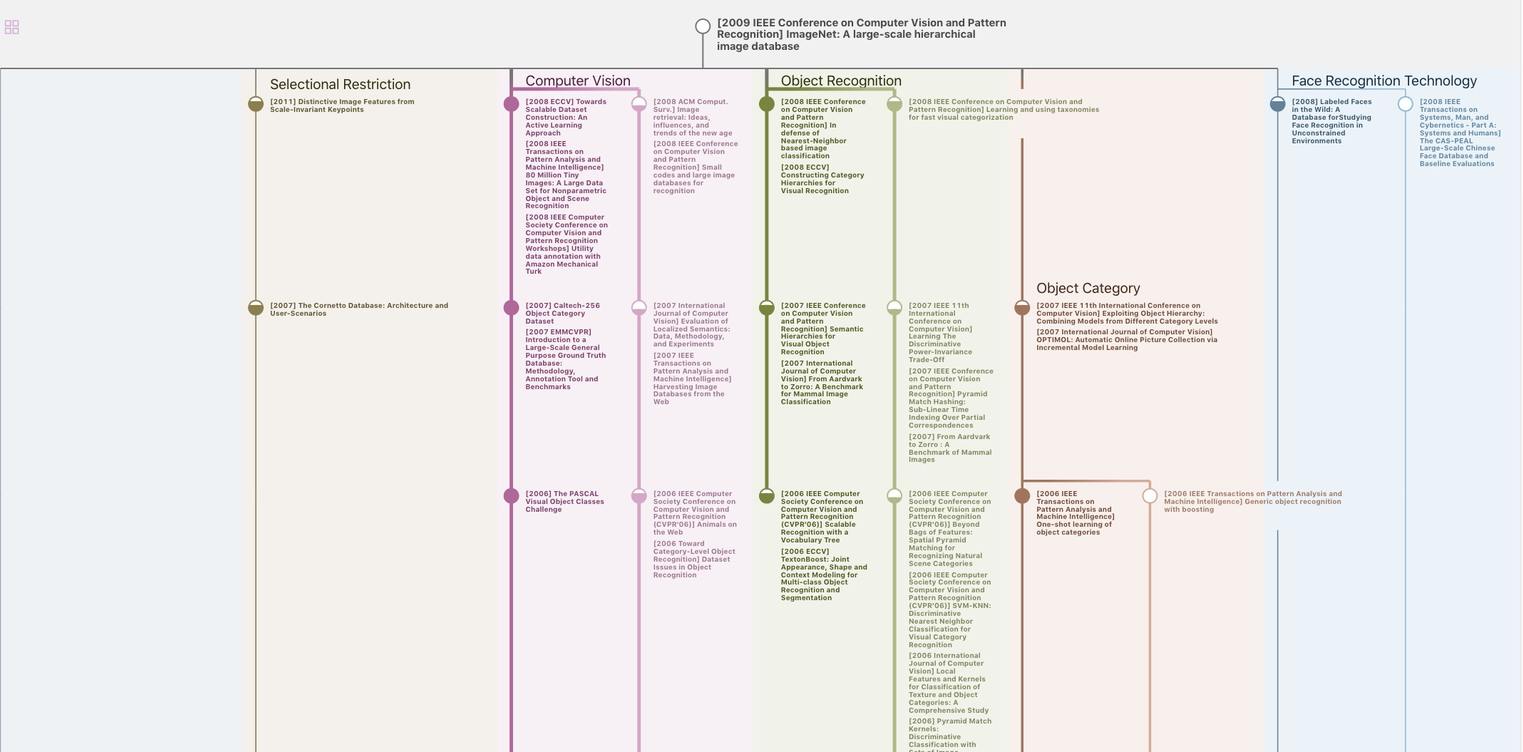
生成溯源树,研究论文发展脉络
Chat Paper
正在生成论文摘要