A DNN-Based Learning Framework for Continuous Movements Segmentation
NEURAL INFORMATION PROCESSING, ICONIP 2023, PT III(2024)
摘要
This study presents a novel experimental paradigm for collecting Electromyography (EMG) data from continuous movement sequences and a Deep Neural Network (DNN) learning framework for segmenting movements from these signals. Unlike prior research focusing on individual movements, this approach characterizes human motion as continuous sequences. The DNN framework comprises a segmentation module for time point level labeling of EMG data and a transfer module predicting movement transition time points. These outputs are integrated based on defined rules. Experimental results reveal an impressive capacity to accurately segment movements, evidenced by segmentation metrics (accuracy: 88.3%; Dice coefficient: 82.9%; mIoU: 72.7%). This innovative approach to time point level analysis of continuous movement sequences via EMG signals offers promising implications for future studies of human motor functions and the advancement of human-machine interaction systems.
更多查看译文
关键词
Human motor analysis,Electromyography,Deep neural networks
AI 理解论文
溯源树
样例
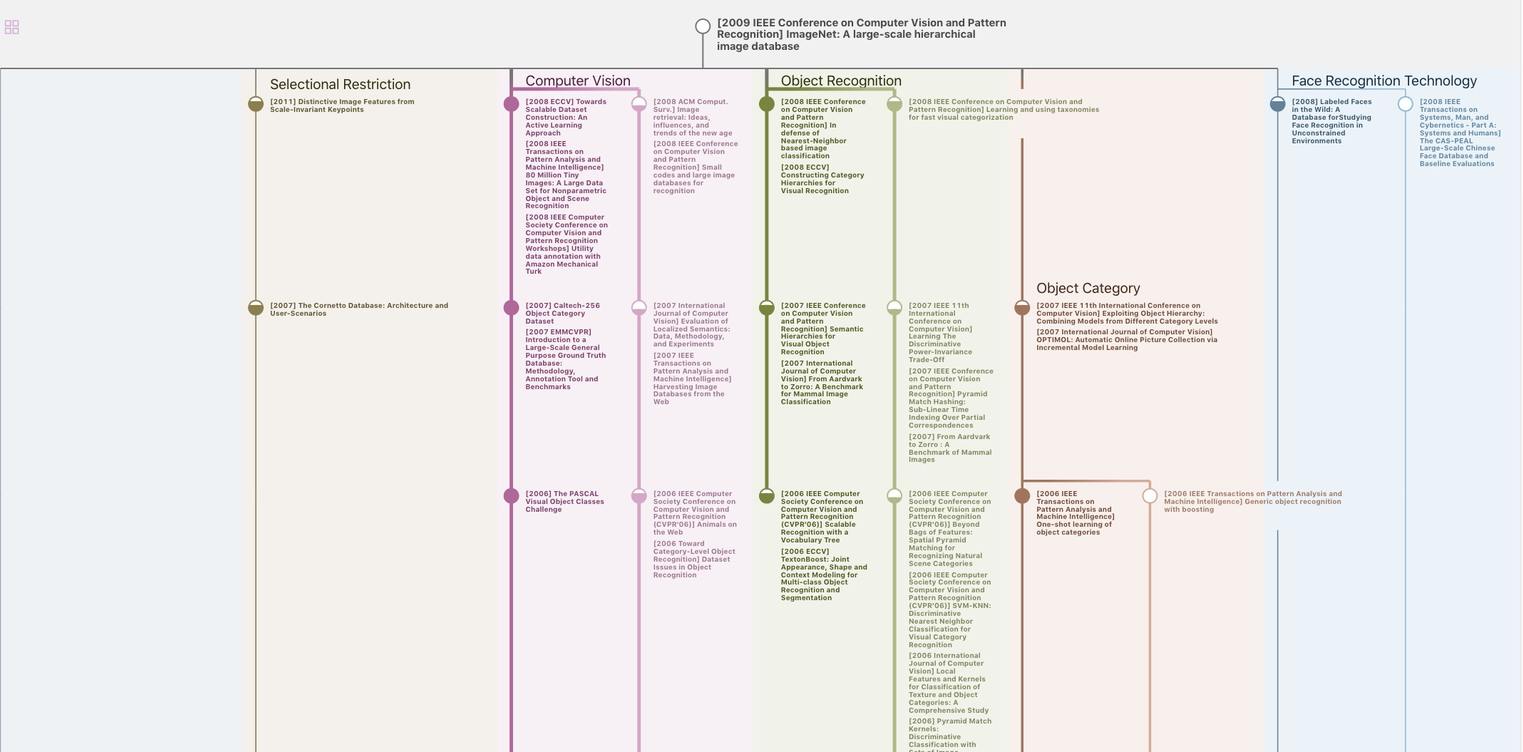
生成溯源树,研究论文发展脉络
Chat Paper
正在生成论文摘要