Temporal Attention for Robust Multiple Object Pose Tracking
NEURAL INFORMATION PROCESSING, ICONIP 2023, PT IV(2024)
摘要
Estimating the pose of multiple objects has improved substantially since deep learning became widely used. However, the performance deteriorates when the objects are highly similar in appearance or when occlusions are present. This issue is usually addressed by leveraging temporal information that takes previous frames as priors to improve the robustness of estimation. Existing methods are either computationally expensive by using multiple frames, or are inefficiently integrated with ad hoc procedures. In this paper, we perform computationally efficient object association between two consecutive frames via attention through a video sequence. Furthermore, instead of heatmap-based approaches, we adopt a coordinate classification strategy that excludes post-processing, where the network is built in an end-to-end fashion. Experiments on real data show that our approach achieves state-of-the-art results on Pose-Track datasets.
更多查看译文
关键词
Pose Estimation,Vision Transformer,Temporal Information
AI 理解论文
溯源树
样例
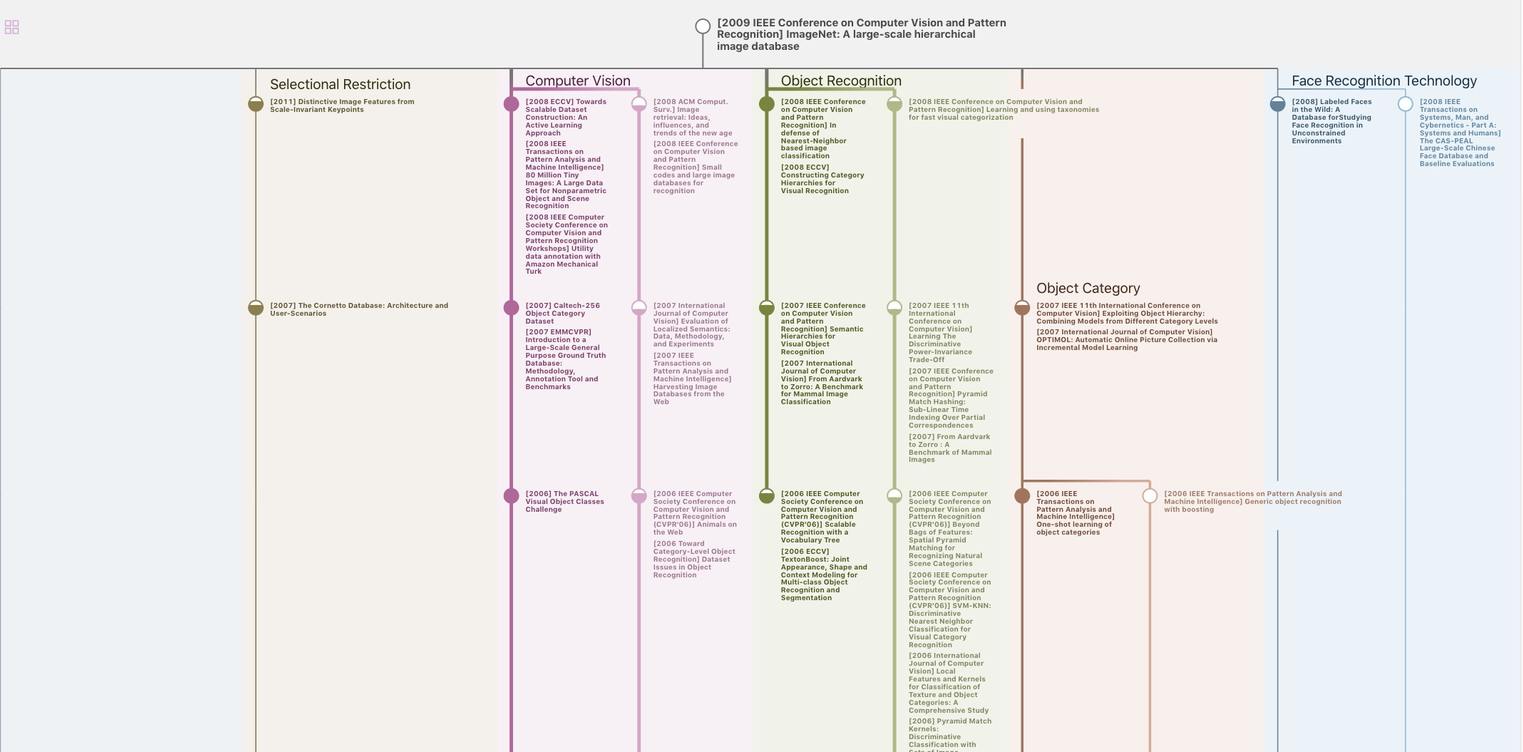
生成溯源树,研究论文发展脉络
Chat Paper
正在生成论文摘要