Learning Representations for Sparse Crowd Answers
NEURAL INFORMATION PROCESSING, ICONIP 2023, PT VI(2024)
摘要
When collecting answers from crowds, if there are many instances, each worker can only provide the answers to a small subset of the instances, and the instance-worker answer matrix is thus sparse. The solutions for improving the quality of crowd answers such as answer aggregation are usually proposed in an unsupervised fashion. In this paper, for enhancing the quality of crowd answers used for inferring true answers, we propose a solution with a self-supervised fashion to effectively learn the potential information in the sparse crowd answers. We propose a method named CROWDLR which first learns rich instance and worker representations from the crowd answers based on two types of self-supervised signals. We create a multi-task model with a Siamese structure to learn two classification tasks for two self-supervised signals in one framework. We then utilize the learned representations to complete the answers to fill the missing answers, and can utilize the answer aggregation methods to the complete answers. The experimental results based on real datasets show that our approach can effectively learn the representations from crowd answers and improve the performance of answer aggregation especially when the crowd answers are sparse.
更多查看译文
关键词
Crowdsourcing,Answer Aggregation,Representation Learning
AI 理解论文
溯源树
样例
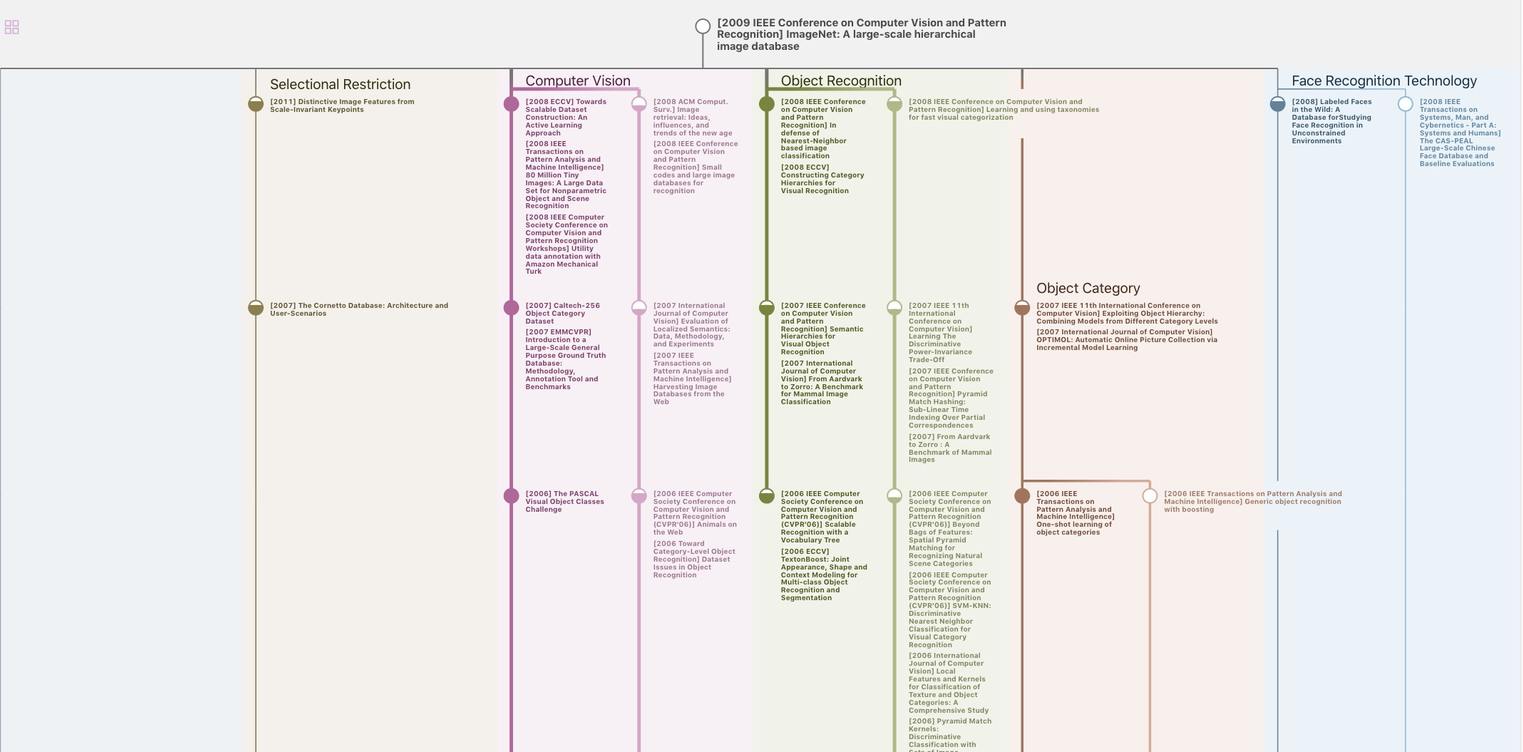
生成溯源树,研究论文发展脉络
Chat Paper
正在生成论文摘要