Domain-Invariant Task Optimization for Cross-domain Recommendation
NEURAL INFORMATION PROCESSING, ICONIP 2023, PT III(2024)
摘要
The challenge of cold start has long been a persistent issue in recommender systems. However, Cross-domain Recommendation (CDR) provides a promising solution by utilizing the abundant information available in the auxiliary source domain to facilitate cold-start recommendations for the target domain. Many existing popular CDR methods only use overlapping user data but ignore non-overlapping user data when training the model to establish a mapping function, which reduces the model's generalization ability. Furthermore, these CDR methods often directly learn the target embedding during training, because the target embedding itself may be unreasonable, resulting in an unreasonable transformed embedding, exacerbating the difficulty of model generalization. To address these issues, we propose a novel framework named Domain-Invariant Task Optimization for Cross-domain Recommendation (DITOCDR). To effectively utilize non-overlapping user information, we employ source and target domain auto-encoders to learn overlapping and non-overlapping user embeddings and extract domain-invariant factors. Additionally, we use a task-optimized strategy for target embedding learning to optimize the embedding and implicitly transform the source domain user embedding to the target feature space. We evaluate our proposed DITOCDR on three real-world datasets collected by Amazon, and the experimental results demonstrate its excellent performance and effectiveness.
更多查看译文
关键词
Cross-domain recommendation,Cold-start recommendations,Task optimization
AI 理解论文
溯源树
样例
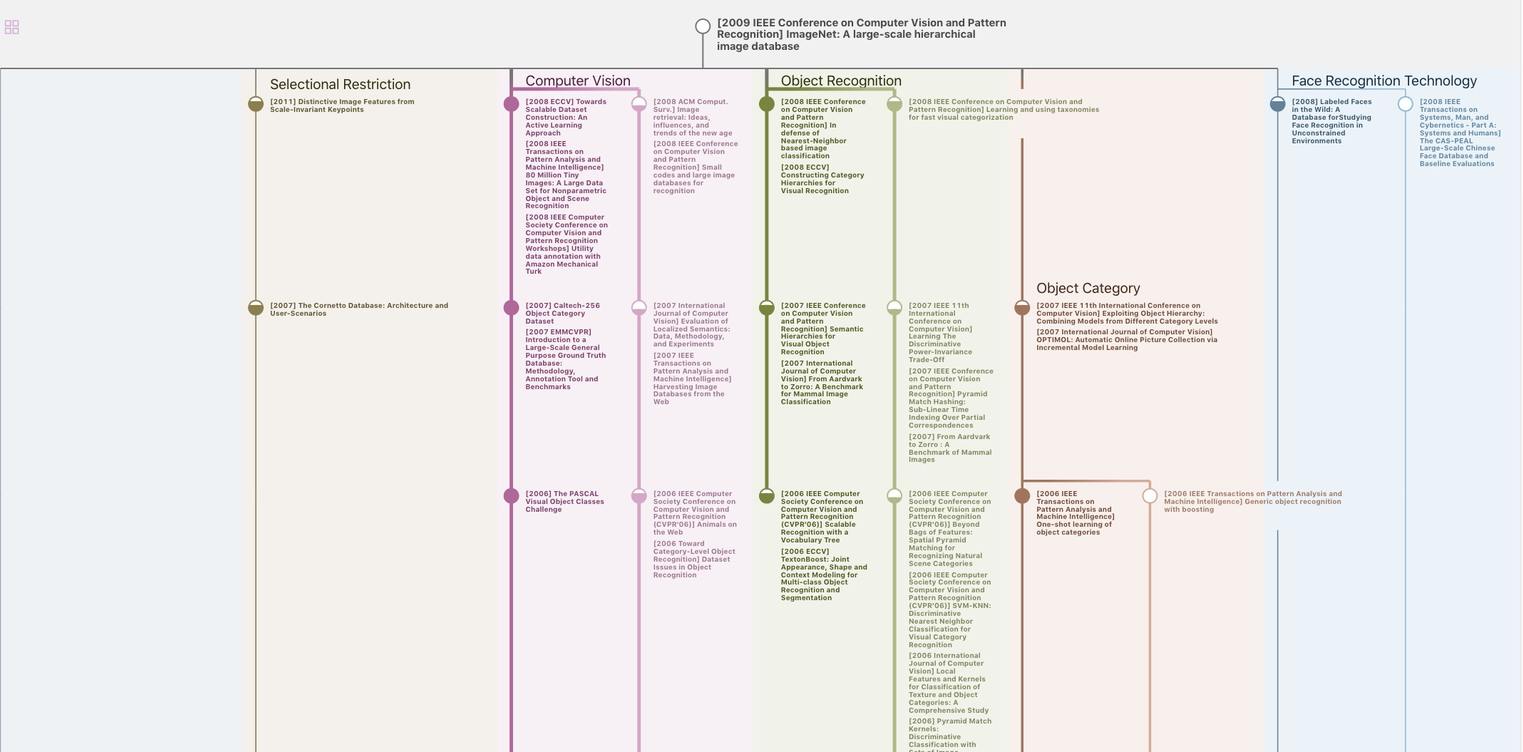
生成溯源树,研究论文发展脉络
Chat Paper
正在生成论文摘要