Efficient Spiking Neural Architecture Search with Mixed Neuron Models and Variable Thresholds
NEURAL INFORMATION PROCESSING, ICONIP 2023, PT II(2024)
摘要
Spiking Neural Networks (SNNs) are emerging as energyefficient alternatives to artificial neural networks (ANNs) due to their event-driven computation and effective processing of temporal information. While Neural Architecture Search (NAS) has been extensively used to optimize neural network structures, its application to SNNs remains limited. Existing studies often overlook the temporal differences in information propagation between ANNs and SNNs. Instead, they focus on shared structures such as convolutional, recurrent, or pooling modules. This work introduces a novel neural architecture search framework, MixedSNN, explicitly designed for SNNs. Inspired by the human brain, MixedSNN incorporates a novel search space called SSP, which explores the impact of utilizing Mixed spiking neurons and Variable thresholds on SNN performance. Additionally, we propose a training-free evaluation strategy called Period-Based Spike Evaluation (PBSE), which leverages spike activation patterns to incorporate temporal features in SNNs. The performance of SNN architectures obtained through MixedSNN is evaluated on three datasets, including CIFAR-10, CIFAR-100, and CIFAR-10DVS. Results demonstrate that MixedSNN can achieve state-of-the-art performance with significantly lower timesteps.
更多查看译文
关键词
Spiking Neural Network,Neural Architecture Search,Brain-inspired Computing,Mixed Neuron Models
AI 理解论文
溯源树
样例
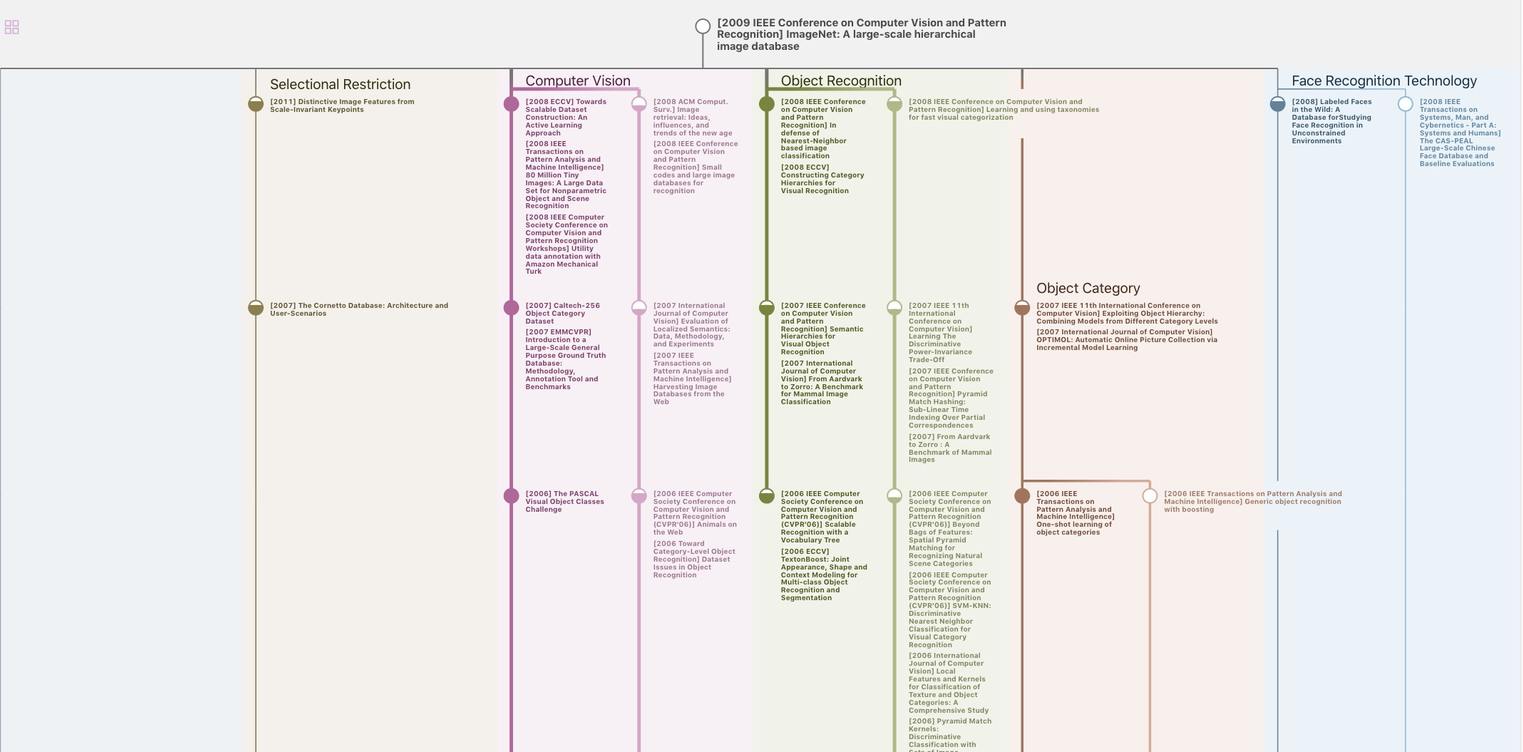
生成溯源树,研究论文发展脉络
Chat Paper
正在生成论文摘要