An End-to-End Dense Connected Heterogeneous Graph Convolutional Neural Network
NEURAL INFORMATION PROCESSING, ICONIP 2023, PT I(2024)
摘要
Graph convolutional networks (GCNs) are powerful models for graph-structured data learning task. However, most existing GCNs may confront with two major challenges when dealing with heterogeneous graph: (1) Predefined meta-paths are required to capture the semantic relations between nodes from different types, which may not exploit all the useful information in the graph; (2) Performance degradation and semantic confusion may happen with the growth of the network depth, which limits their ability to capture long-range dependencies. To meet these challenges, we propose Dense-HGCN, an end-to-end dense connected heterogeneous convolutional neural network to learn node representation. Dense-HGCN computes the attention weights between different nodes and incorporates the information of previous layers into each layer's aggregation process via a specific fuse function. Moreover, Dense-HGCN leverages multi-scale information for node classification or other downstream tasks. Experimental results on real-world datasets demonstrate the superior performance of Dense-HGCN in enhancing the representational power compared with several state-of-the-art methods.
更多查看译文
关键词
Graph neural network,Heterogeneous graph,Multi-scale
AI 理解论文
溯源树
样例
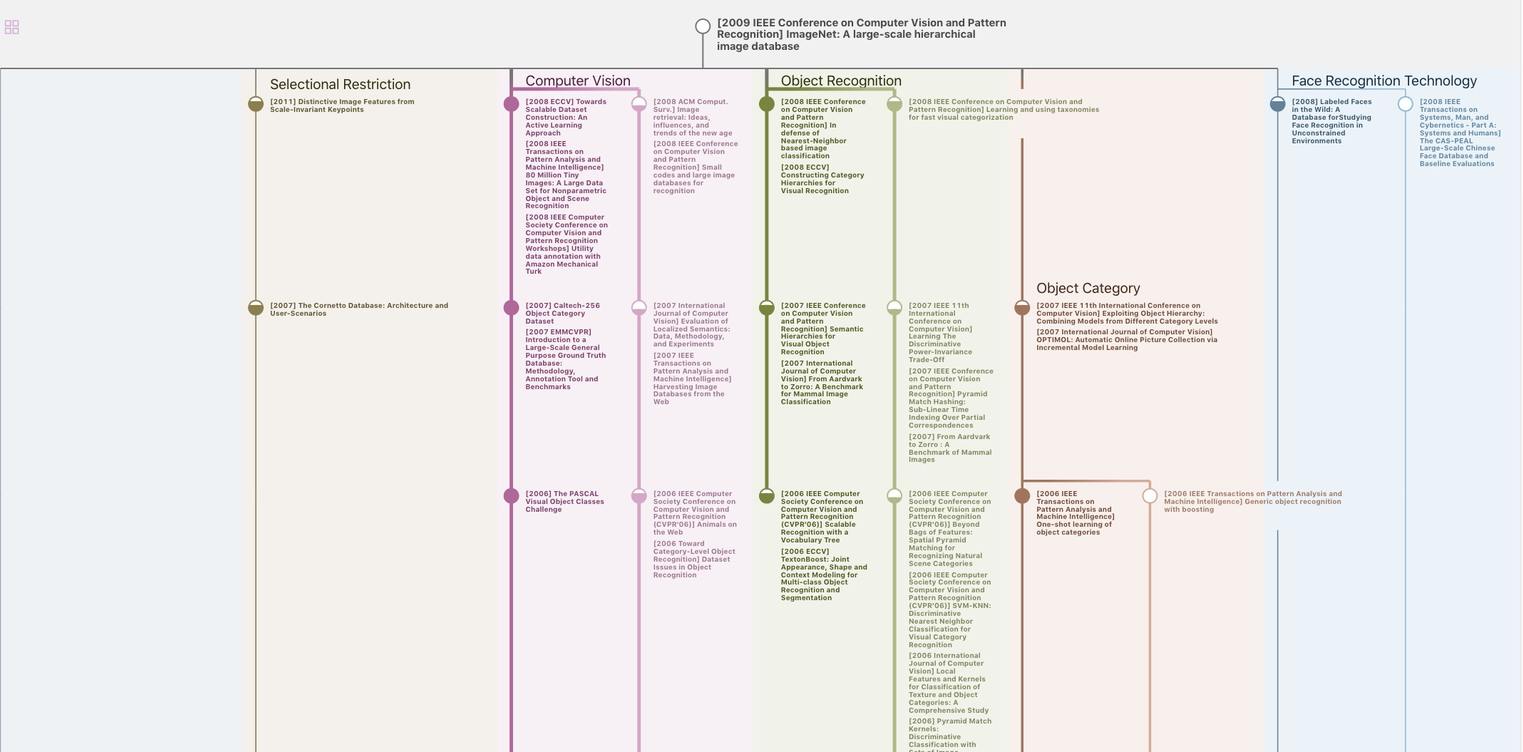
生成溯源树,研究论文发展脉络
Chat Paper
正在生成论文摘要