CAS-NN: A Robust Cascade Neural Network Without Compromising Clean Accuracy
NEURAL INFORMATION PROCESSING, ICONIP 2023, PT II(2024)
摘要
Adversarial training has emerged as a prominent approach for training robust classifiers. However, recent researches indicate that adversarial training inevitably results in a decline in a classifier's accuracy on clean (natural) data. Robustness is at odds with clean accuracy due to the inherent tension between the objectives of adversarial robustness and standard generalization. Training a single classifier that combines high adversarial robustness and high clean accuracy appears to be an insurmountable challenge. This paper proposes a straightforward strategy to bridge the gap between robustness and clean accuracy. Inspired by the idea underlying dynamic neural networks, i.e., adaptive inference, we propose a robust cascade framework that integrates a standard classifier and a robust classifier. The cascade neural network dynamically classifies clean and adversarial samples using distinct classifiers based on the confidence score of each input sample. As deep neural networks suffer from serious overconfident problems on adversarial samples, we propose an effective confidence calibration algorithm for the standard classifier, enabling accurate confidence scores for adversarial samples. The experiments demonstrate that the proposed cascade neural network increases the clean accuracies by 10.1%, 14.67%, and 9.11% compared to the advanced adversarial training (HAT) on CIFAR10, CIFAR100, and Tiny ImageNet while keeping similar robust accuracies.
更多查看译文
关键词
Adversarial training,Adversarial learning
AI 理解论文
溯源树
样例
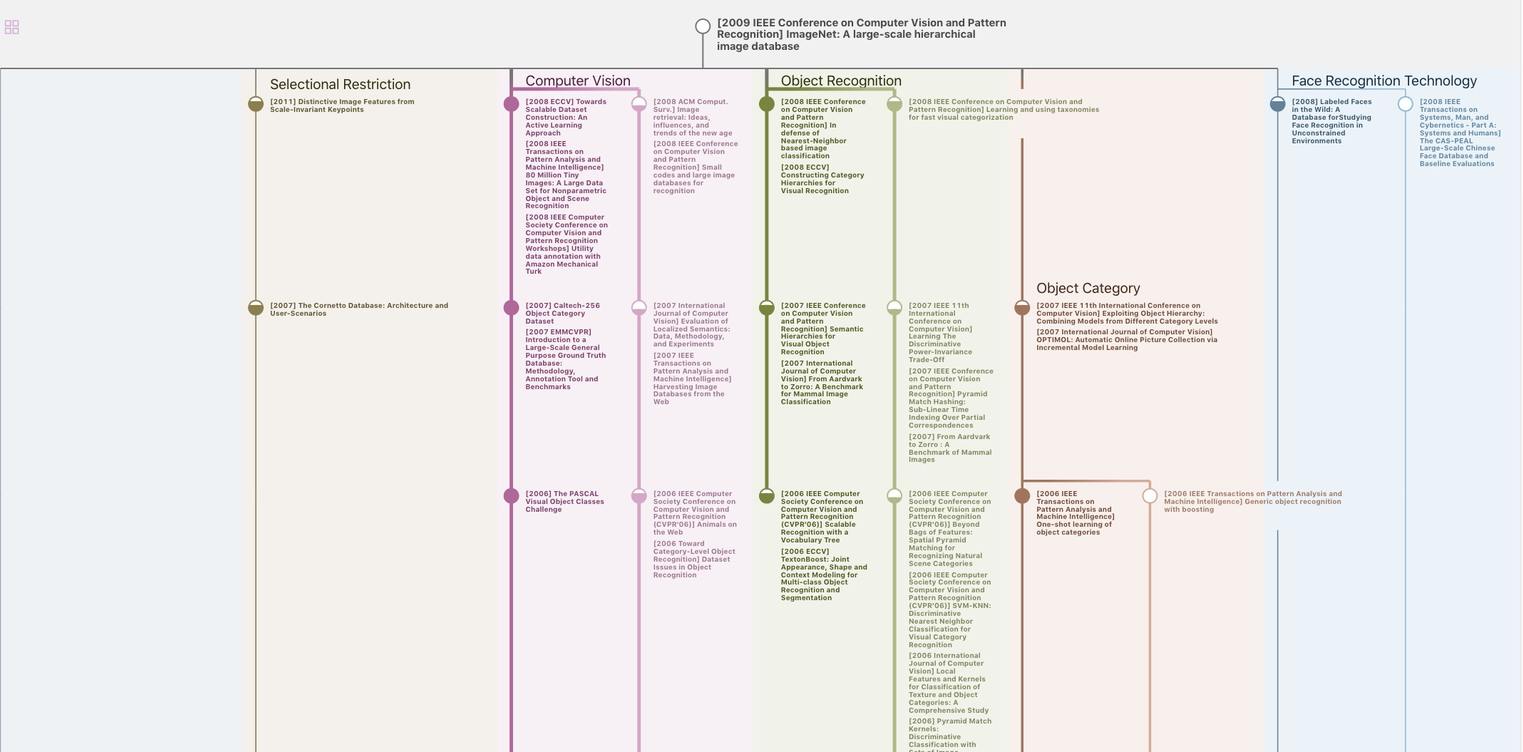
生成溯源树,研究论文发展脉络
Chat Paper
正在生成论文摘要