Large Scale Financial Time Series Forecasting with Multi-faceted Model
PROCEEDINGS OF THE 4TH ACM INTERNATIONAL CONFERENCE ON AI IN FINANCE, ICAIF 2023(2023)
摘要
Data-driven approaches using deep neural networks have been successful in modeling complex financial time series and generating accurate predictions without requiring extensive domain knowledge. However, most of the existing models that assume independent and identically distributed (i.i.d) data may not generalize well to novel situations or distributional shifts across or inside financial scenarios. To address this challenge, we introduce an invariant learning-based regularizer with relaxed bounds that expands the range of feasible solutions and mitigates over-convergence issues in Invariant Risk Minimization (IRM). In practice, the regularizer can be incorporated into both linear and nonlinear financial time series forecasting models. Experimental results on real-world large-scale financial datasets show that our proposed method enables more robust and adaptable financial forecasting models, enhancing the overall performance and generalizability of financial forecasting on both in-distribution and out-of-distribution (OOD) samples.
更多查看译文
关键词
Financial time series,Forecasting algorithm,Distributional shifts
AI 理解论文
溯源树
样例
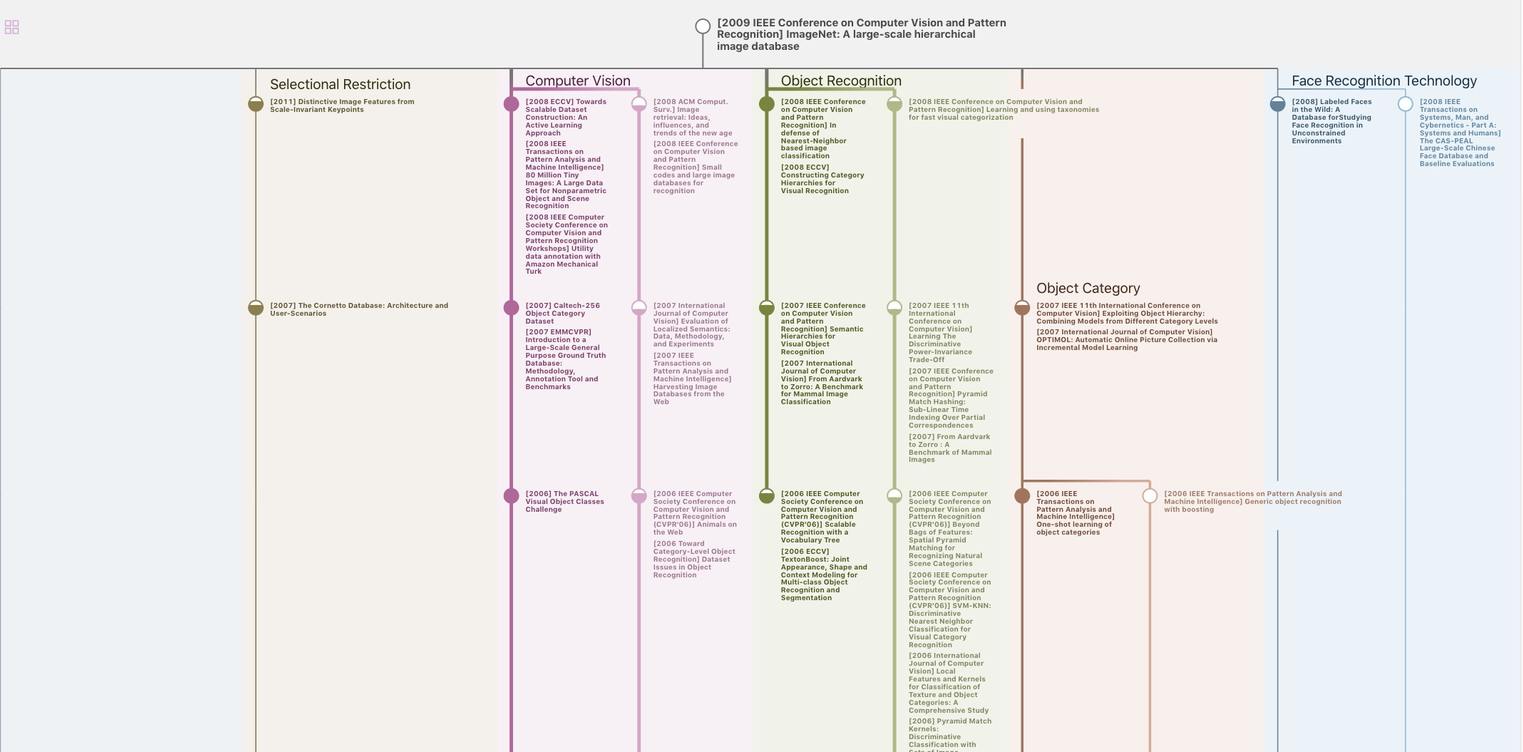
生成溯源树,研究论文发展脉络
Chat Paper
正在生成论文摘要