Predictability of Post-Earnings Announcement Drift with Textual and Contextual Factors of Earnings Calls
PROCEEDINGS OF THE 4TH ACM INTERNATIONAL CONFERENCE ON AI IN FINANCE, ICAIF 2023(2023)
摘要
Post-Earnings Announcement Drift (PEAD), a well-known anomaly in financial markets, describes the tendency of cumulative stock returns to drift in the direction of an earnings surprise for a prolonged period following an earnings announcement. Numerous studies have used a supervised learning approach to predict PEAD, using earnings, fundamental and technical factors. However, there is a lack of study on how the context of the earnings call can be used for the PEAD prediction task. This paper uses computational linguistics techniques and large language models to examine the effectiveness of incorporating textual and contextual features from earnings calls for the PEAD prediction task. Our proposed supervised model includes four categories of features: 1) textual features, 2) contextual features, 3) earnings features, and 4) fundamental and technical features. We study the proposed model using earnings from 2010/01/01 to 2022/12/31 of all point-in-time S&P500 constituents in the US stock market. Our results show that contextual features provide information unexplained by earnings, fundamental and technical features, improving the average returns per trade of a hypothetical long-short portfolio against baseline solution in out-of-sample across all four different abnormal return calculations, ranging from 53 to 354 basis points and 16.9% to 108.5% improvement from baseline model, which uses only earnings, fundamental and technical features.
更多查看译文
关键词
Post-earnings announcement drift,earnings call,computational linguistics,large language models,machine learning
AI 理解论文
溯源树
样例
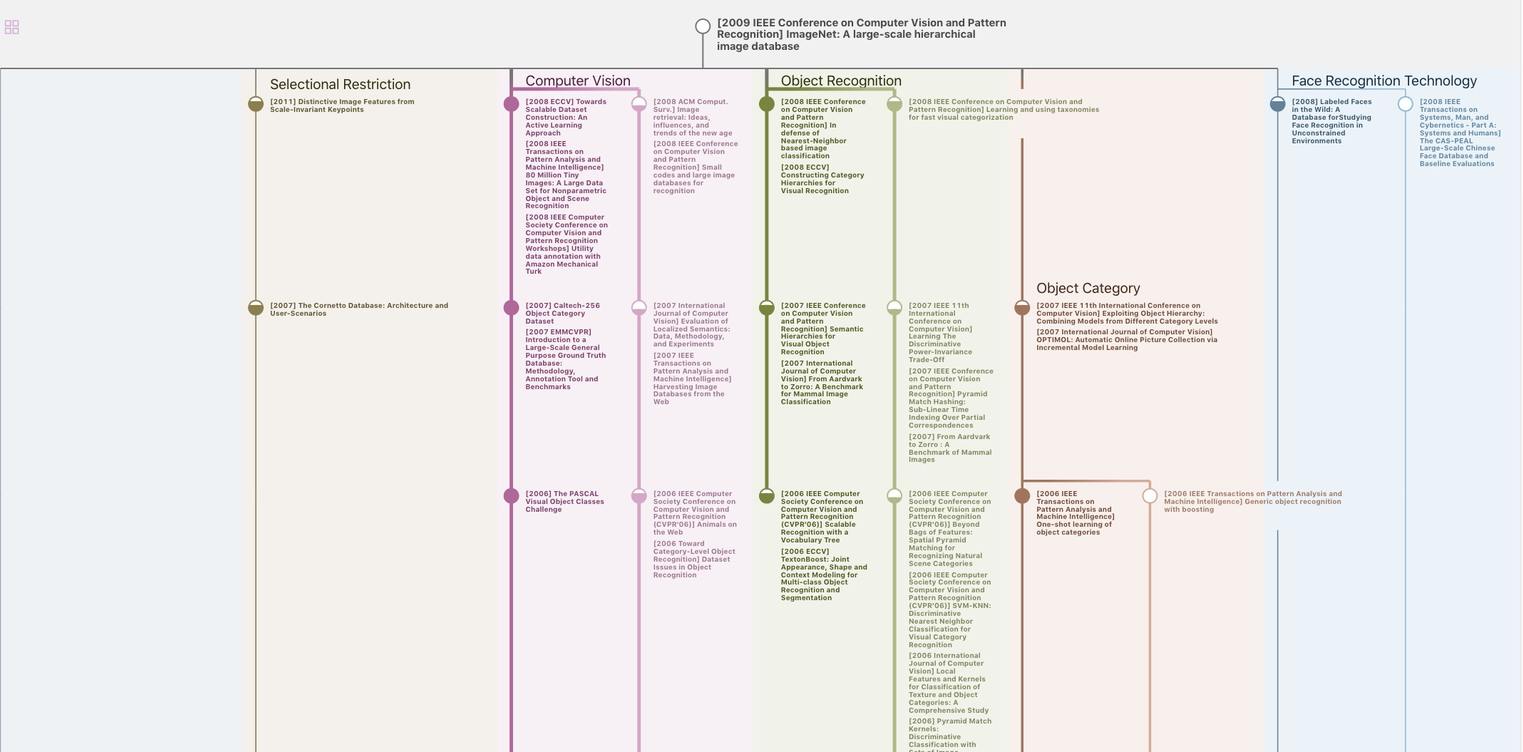
生成溯源树,研究论文发展脉络
Chat Paper
正在生成论文摘要