Development of a Handwriting Drawings Assessment System for Early Parkinson's Disease Identification with Deep Learning Methods.
FDSE(2023)
摘要
Parkinson’s disease (PD) is a prevalent neurodegenerative disorder, and early detection plays a crucial role in timely treatment to prevent further harm to patients. In recent years, researchers have primarily employed machine learning methods using clinical manifestations of PD patients for diagnosis, such as gait rigidity and distorted handwriting. Hand tremors and handwriting difficulties are typical early motor symptoms of PD, making handwriting analysis an important tool for detecting PD. However, previous approaches have limitations in capturing subtle variations in handwriting and often combine other biological signals. This study aims to develop a deep learning-based handwriting drawings assessment system that relies solely on patients’ handwriting as vital evidence for early-stage Parkinson’s diagnosis. We utilized two publicly available datasets, HandPD and NewHandPD, which contain hand-drawn spirals and meanders from PD patients and healthy participants. We employed EfficientNet-B1, ResNet-34, ResNet-101 and DenseNet-121 deep learning models for the classification task. Experimental results demonstrated that the EfficientNet-B1 network achieved the best performance on patients’ meander traced graphics, with a precision and sensitivity of 97.62% and an accuracy of 96.36%. Furthermore, we created a Python Web API based on Flask and a user-friendly Windows application for the assessment system, enabling its use in screening tests for Parkinson’s disease diagnosis. This system holds promising potential for aiding early detection and providing valuable support to healthcare professionals in diagnosing Parkinson’s disease effectively.
更多查看译文
AI 理解论文
溯源树
样例
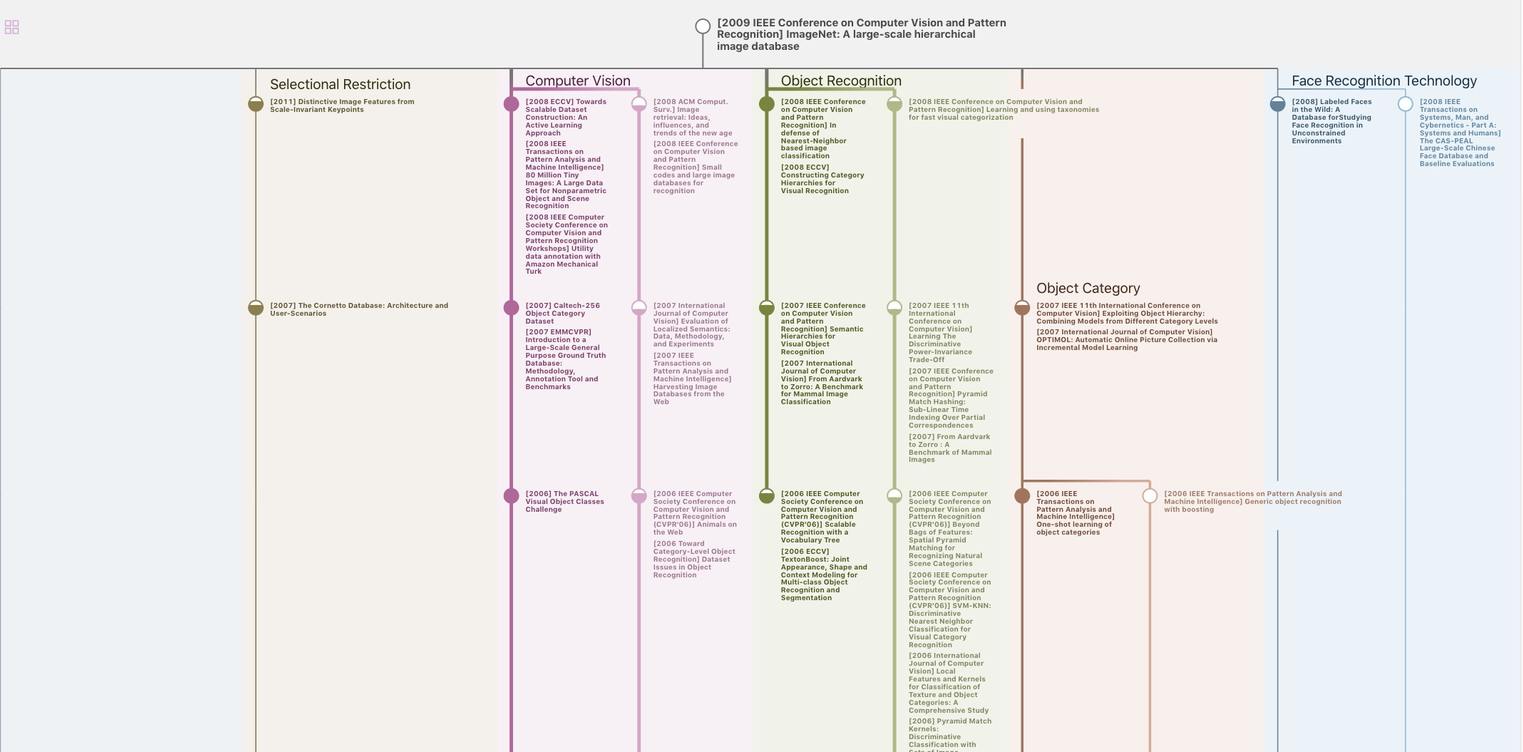
生成溯源树,研究论文发展脉络
Chat Paper
正在生成论文摘要