Deep Knowledge Tracing with Concept Trees.
ADMA (2)(2023)
摘要
Knowledge tracing aims to diagnose the student’s knowledge status and predict the responses to the next questions, which is a critical task in personalized learning. The existing studies consider more academic features, while this paper introduces DKCT, a deep knowledge tracing model with concept trees, to integrate the hierarchical concept tree that describes the structure of concepts in a question. DKCT casts the knowledge concept tree (KCT) in a question from the views of feature, breadth, and difficulty into a KCT representation at first. Then, DKCT is composed of an encoder network with multi-head attention on the question representations and a decoder network with multi-head attention on the interaction embeddings. Finally, DKCT integrates the student embeddings by using fully connected networks to predict the responses to the next questions. Extensive experiments conducted on two real-world educational datasets show that DKCT has a higher prediction accuracy than the currently popular KT models. This work paves the way to consider KCT for knowledge tracing.
更多查看译文
关键词
knowledge,trees,concept
AI 理解论文
溯源树
样例
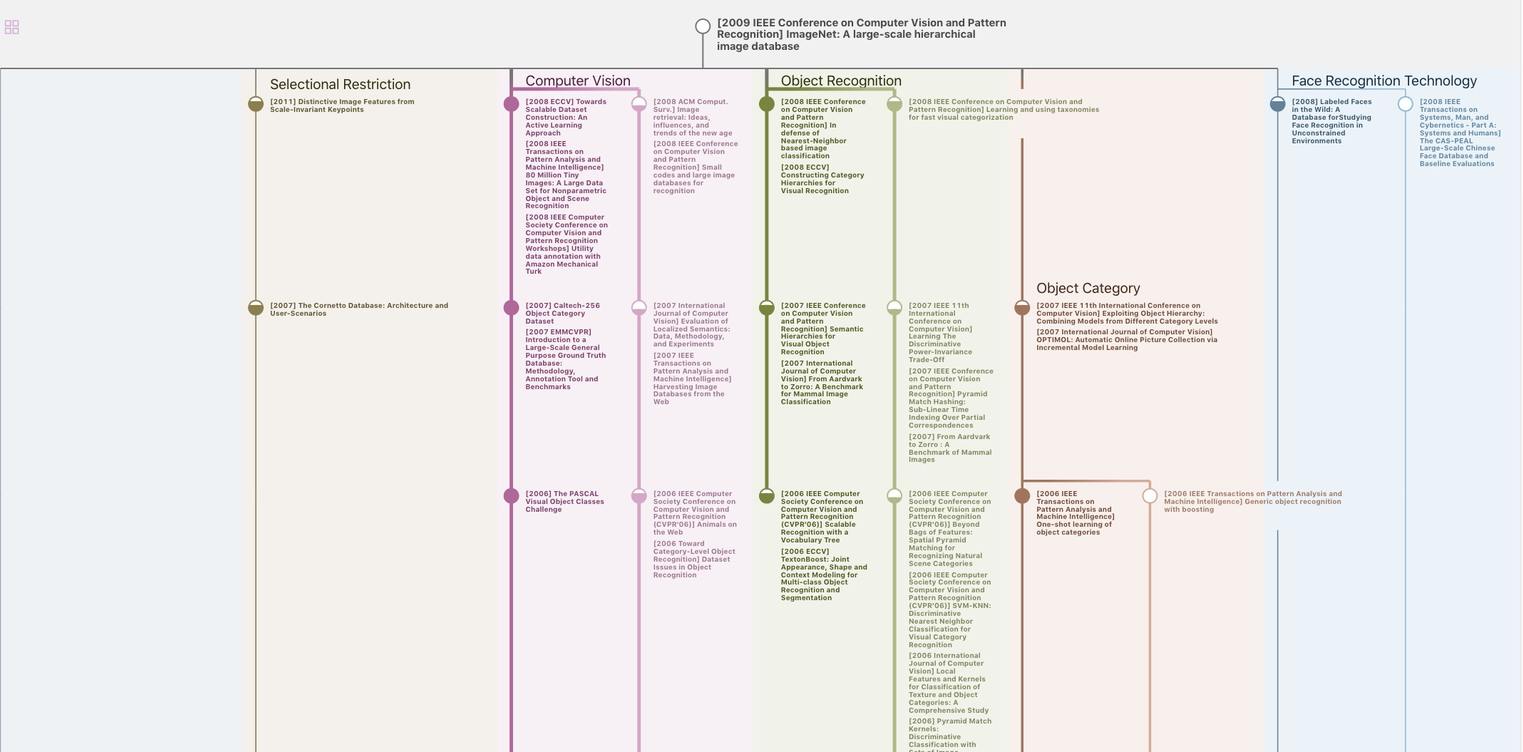
生成溯源树,研究论文发展脉络
Chat Paper
正在生成论文摘要