FeaBI: A Feature Selection-Based Framework for Interpreting KG Embeddings
SEMANTIC WEB, ISWC 2023, PART I(2023)
摘要
Knowledge Graph (KG) embedding methods represent KG entities as vectors in an embedding space, and they have been successfully used for a variety of tasks, including link prediction and entity classification. While some of the recent embedding methods outperform traditional approaches on these tasks, their main drawback is the lack of interpretability. Several methods for explaining predictions made by KG embeddings have been proposed in the literature. However, none of them targeted the problem of constructing model explanations for embeddings, i.e., interpretable KG representations that behave similarly to embeddings on certain tasks. We address this problem and propose a novel method for generating interpretable vectors for entity embeddings. To achieve this, we employ embedded feature selection techniques to extract from the KG, on which the embedding model was trained, propositional features that are important for a given KG embedding model. Our approach sheds light on the information in the KG captured by embeddings and provides valuable insights that can be used to further enhance the embedding models. Additionally, we demonstrate the usefulness of our method for explaining embedding-based entity similarity.
更多查看译文
AI 理解论文
溯源树
样例
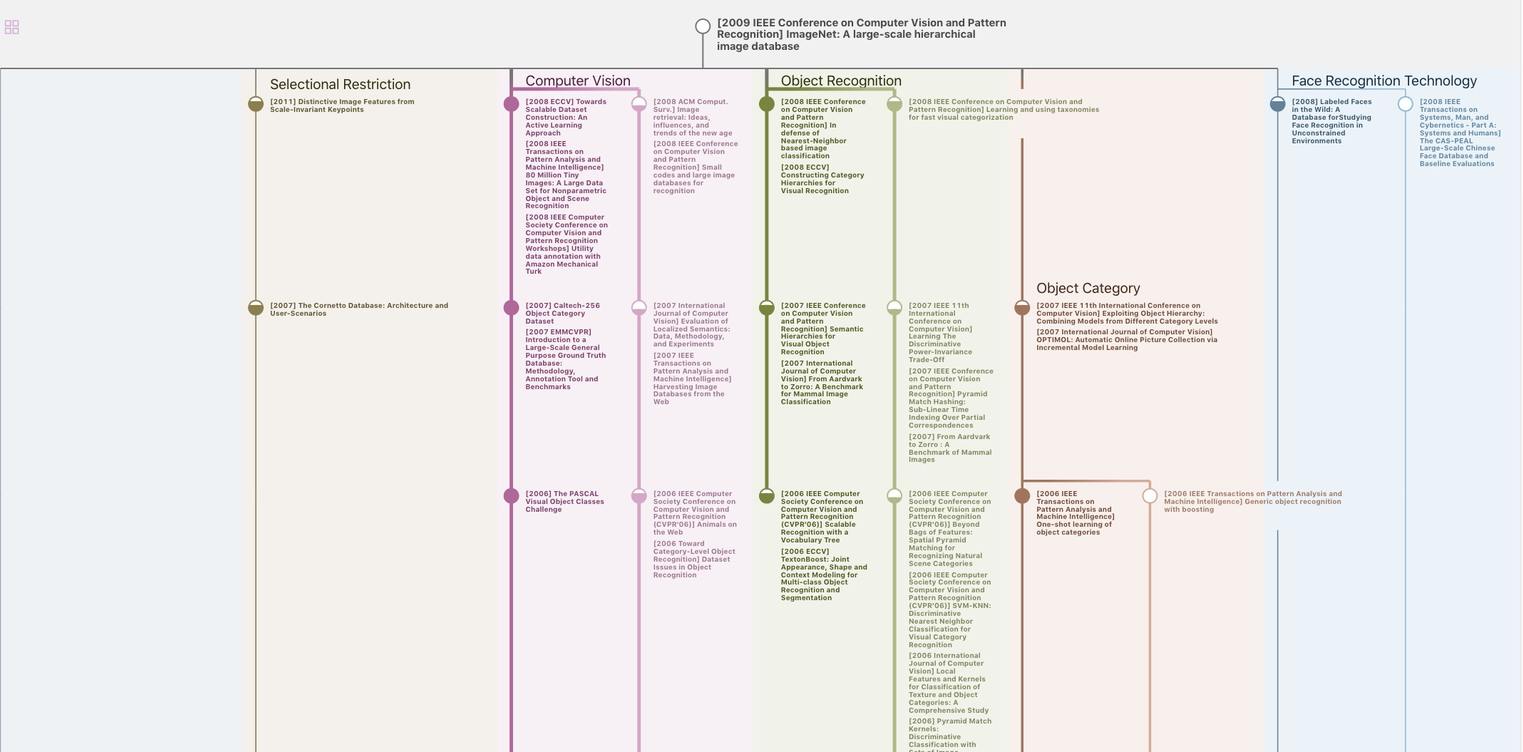
生成溯源树,研究论文发展脉络
Chat Paper
正在生成论文摘要