A novel data-driven optimization framework for unsupervised and multivariate early-warning threshold modification in risk assessment of deep excavations
EXPERT SYSTEMS WITH APPLICATIONS(2024)
摘要
Due to the great uncertainty and complexity in the underground environment, precious risk perception in underground construction is a highly challenging problem. Excessive false-positive alarms (FPA) and false-negative alarms (FNA) generated by the unreasonable setting of early-warning values are the main causes to affect the safety of engineering in underground construction. Aiming to reduce the FPA and FNA in risk assessment of an excavation procedure, this paper provides a novel data-driven optimization framework for early-warning threshold modification based on massive field monitoring data. The multi-objective expected value optimiza-tion (MEVO) approach is applied to achieve the goal of unsupervised and multivariate modification tasks. Firstly, the objective functions of the MEVO approach are established with the combination of grey relational analysis and Cosine similarity measure. Secondly, the expected values of objective functions are calculated based on the expert evaluation method and Monte Carlo simulation. Finally, the sample sequence generated from observed monitoring data satisfying the expected values is selected as the optimal solution. A case study is utilized to verify the effectiveness and applicability of the proposed approach. The result from the case study indicates that: (1) The proposed approach modifies the multivariate early-warning values to decrease the number of alarms with a reduction ratio of 12% in the training set; (2) The early-warning frequency under the modified values is lower than that under the original values during the whole excavation stages in the testing set; (3) The modified threshold values fall within the threshold intervals based on expert evaluation method, which are capable of improving risk assessment in current practice. In short, our proposed approach enables a more accurate and intelligent evaluation of the risk state by formulating more credible criteria. It contributes to serving as a reliable decision tool for ensuring construction safety during deep excavation.
更多查看译文
关键词
Underground construction,Data-driven optimization framework,Threshold modification,Monte Carlo simulation,Risk assessment
AI 理解论文
溯源树
样例
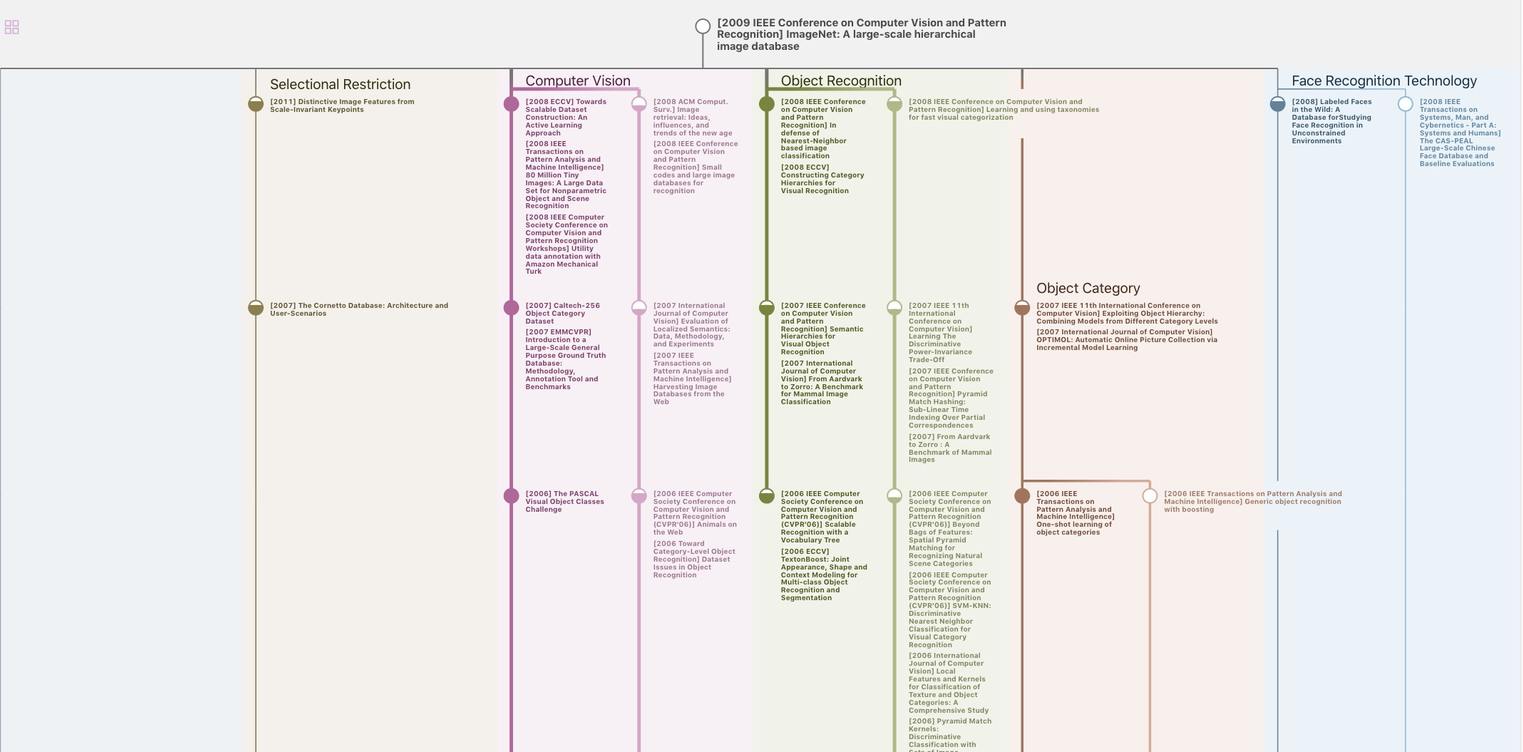
生成溯源树,研究论文发展脉络
Chat Paper
正在生成论文摘要