MSGCN-ISTL: A multi-scaled self-attention-enhanced graph convolutional network with improved STL decomposition for probabilistic load forecasting
EXPERT SYSTEMS WITH APPLICATIONS(2024)
摘要
Probabilistic load forecasting has gained widespread attention for its effectiveness in providing scientific power dispatch plans in recent years. Most existing works adopted decomposition strategies to handle the temporal features of load data, but ignored the fact that decomposition components have different levels of importance, resulting in the incomplete extraction of temporal correlation information. Moreover, previous works have primarily concentrated on the geographical correlation among neighboring load zones and overlooked the semantic correlation among load zones, resulting in insufficient extraction of spatial correlation information. In this study, a novel multi-scaled self-attention-enhanced graph convolutional network with improved Seasonal-Trend decomposition based on Loess (STL) decomposition (MSGCN-ISTL) is proposed for probabilistic load forecasting. First, an improved STL decomposition is proposed, which adopts a convolutional neural network with dual convolutional channels to separately handle the different decomposition components, thus improving the extraction of temporal correlation information. Second, a new multi-scaled self-attention-enhanced graph convolutional network is proposed to extract the spatial correlation information from geographical and semantic aspects simultaneously, which enriches the receptive fields and extracts the multi-scaled enhanced spatial features. Experimental results conducted under various conditions demonstrate the superiority and robustness of the proposed model.
更多查看译文
关键词
Probabilistic load forecasting,Graph convolutional network,Spatial correlation information,Multi-scaled self-attention
AI 理解论文
溯源树
样例
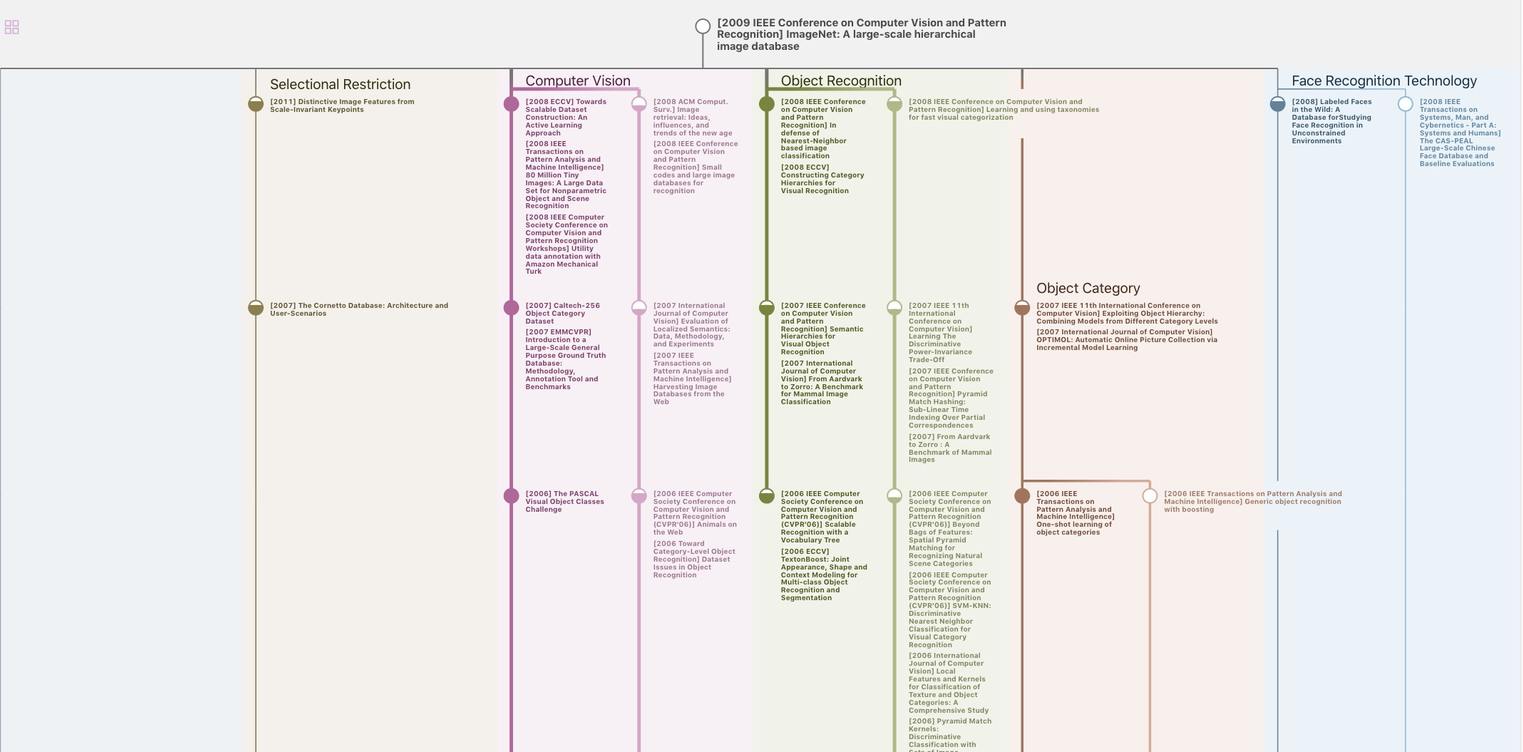
生成溯源树,研究论文发展脉络
Chat Paper
正在生成论文摘要