A fast local citation recommendation algorithm scalable to multi-topics *
EXPERT SYSTEMS WITH APPLICATIONS(2024)
摘要
In the era of rapid paper publications in various venues, automatic citation recommendations would be highly useful to researchers when they write papers. Local citation recommendation aims to recommend possible papers to cite given local citation contexts. Previous work mainly computes the similarity score between citation contexts and cited papers on a one-to-one basis, which is quite time-consuming. We train a pair of neural network encoders that map citation contexts and all possible cited papers to the same vector space, respectively. After that, we index the positions of all cited papers in the vector space. This makes our process for searching recommended papers considerably faster. On the other hand, existing methods tend to recommend papers that are highly similar to each other, which makes recommendations lack diversity. Therefore, we extend our algorithm to perform multi-topic recommendations. We generate multi-topic training examples based on the index we mentioned earlier. Furthermore, we specially design a multi-group contrastive learning method to train our model so that it can distinguish different topics. Empirical experiments show that our model outperforms previous methods by a wide margin. Our model is also light weighted and has been deployed online so that researchers can use it to obtain recommended citations for their own paper in real-time.
更多查看译文
关键词
Machine learning,Information retrieval,Natural language processing,Citation recommendation
AI 理解论文
溯源树
样例
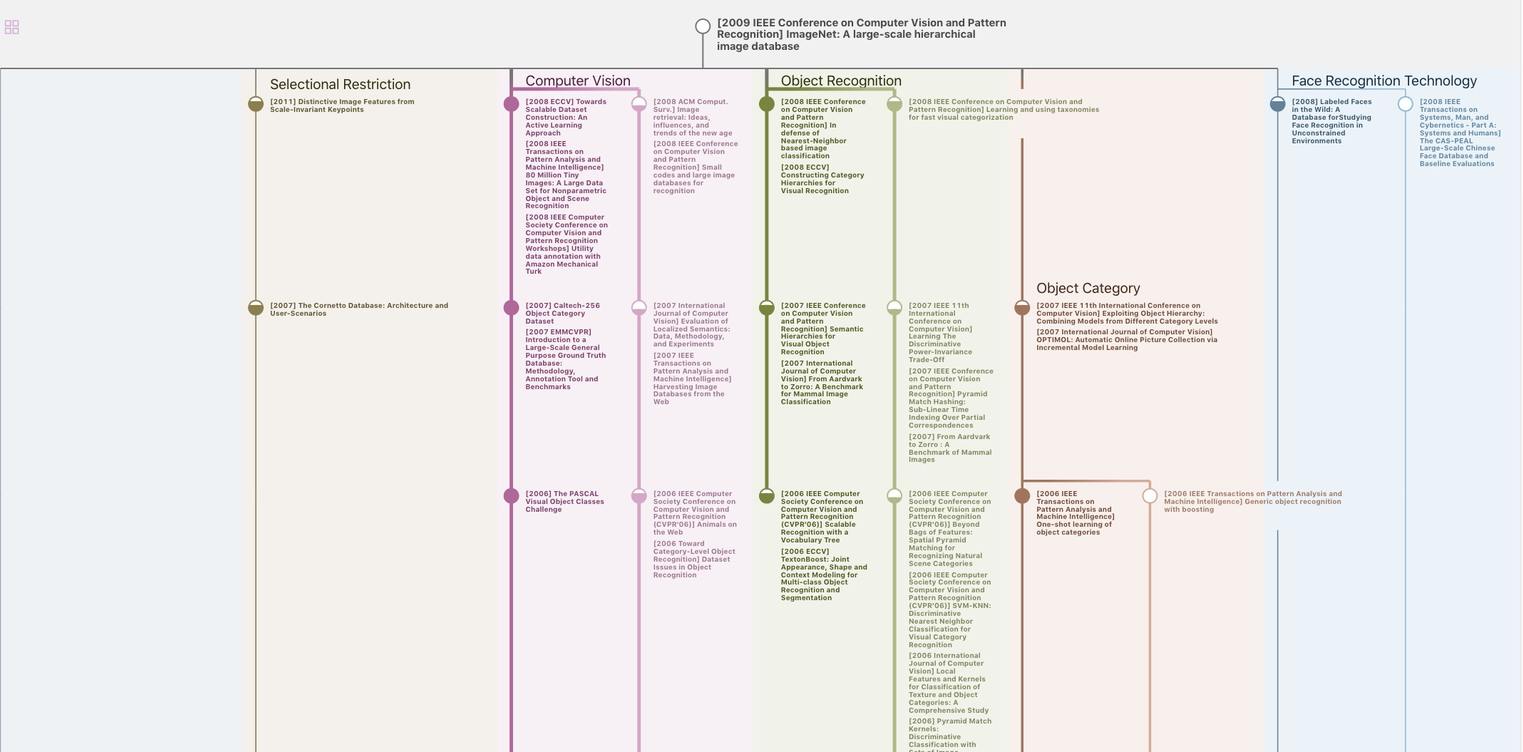
生成溯源树,研究论文发展脉络
Chat Paper
正在生成论文摘要