A new hybrid model for monthly runoff prediction using ELMAN neural network based on decomposition-integration structure with local error correction method
EXPERT SYSTEMS WITH APPLICATIONS(2024)
摘要
The important foundation for water resource management and utilization is effective monthly runoff prediction. In this study, a new coupled model for predicting monthly runoff is proposed. In order to predict the decomposed subsequences separately using an ELMAN neural network optimized by the sparrow search algorithm (SSA), this model first decomposes the original runoff series using robust local mean decomposition (RLMD) and complete ensemble empirical mode decomposition with adaptive noise (CEEMDAN) predicts the decomposed subsequences separately using an ELMAN neural network optimized by the sparrow search algorithm (SSA), and reconstructs the results to get initial prediction results. To acquire the final prediction result, local error correction (LEC) is used to perform error correction on the initial prediction model. Five evaluation indicators are used to assess the performance of the suggested coupling model on monthly runoff data from three experimental stations in China. After having analyzed the error correction capability of LEC model, it is found that CEEMDANRLMD-SSA-ELMAN-LEC decreases root mean square error (RMSE) values of Manwan Hydropower, Jiayuguan Station, and Yingluoxia Station by 18.52%, 21.78%, and 38.80%, respectively, and increases Nash-Sutcliffe efficiency coefficient (NSEC) values by 2.11%, 4.49%, and 5.43% compared to CEEMDAN-RLMD-SSA-ELMAN without error correction. Therefore, by incorporating error correction, the proposed coupled model is a trustworthy and beneficial means for forecasting monthly runoff.
更多查看译文
关键词
Monthly runoff forecast,Robust local mean decomposition,Local error correction,Complete ensemble empirical mode,decomposition with adaptive noise,Elman neural network
AI 理解论文
溯源树
样例
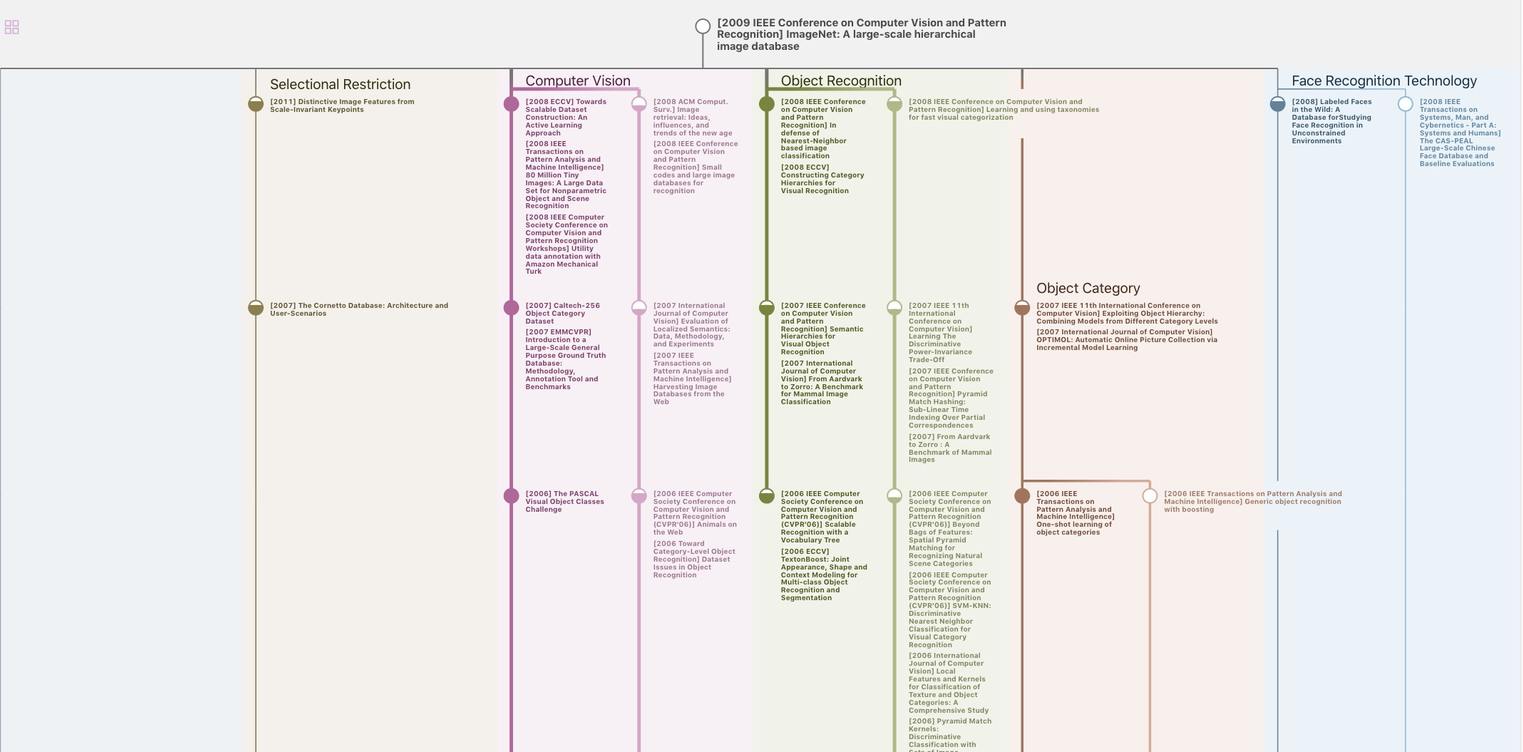
生成溯源树,研究论文发展脉络
Chat Paper
正在生成论文摘要