A novel recurrent convolutional network based on grid correlation modeling for crowd flow prediction
JOURNAL OF KING SAUD UNIVERSITY-COMPUTER AND INFORMATION SCIENCES(2023)
摘要
Due to the increasing implementation of smart cities, crowd flow prediction has become a crucial aspect in various fields such as transportation management and public risk assessment. However, accurately predicting crowd flow can be influenced by various complex factors, including local and long-range spatial dependencies, as well as long-term temporal dependencies. Many works have attempted to model long-range spatial dependence relying on stacked convolutional layers, failing to directly consider it from a global perspective. In this article, we propose a novel recurrent convolutional network based on grid correlation, named RCNGC. In this model, the correlation between different grids based on crowd flow pattern is identified first. Then, a deep network following an encoding-prediction framework is proposed. Specifically, a novel GC-ConvLSTM module based on the grid correlation is utilized to capture long-range dependence in both temporal and spatial dimensions in encoding phase while ConvLSTM is leveraged in prediction phase. We conduct extensive experiments on a dataset in Futian, China. The results demonstrate that the proposed RCNGC is superior to baseline methods in multi-step prediction, indicating that identifying the correlation between grids preliminarily can improve the precision of prediction.(c) 2023 The Author(s). Published by Elsevier B.V. on behalf of King Saud University. This is an open access article under the CC BY-NC-ND license (http://creativecommons.org/licenses/by-nc-nd/4.0/).
更多查看译文
关键词
grid correlation,convolutional network,flow,prediction
AI 理解论文
溯源树
样例
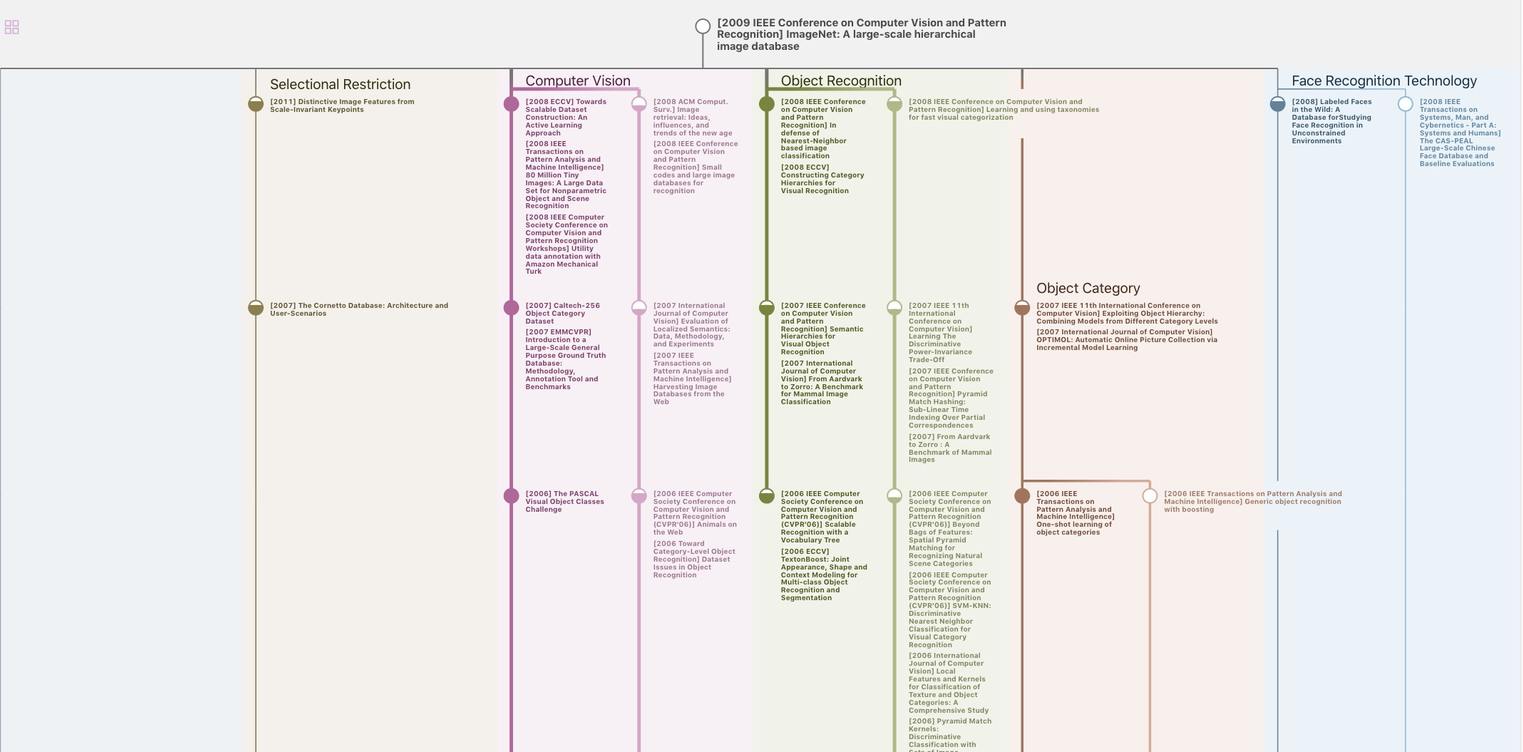
生成溯源树,研究论文发展脉络
Chat Paper
正在生成论文摘要