MetaSignal: Meta Reinforcement Learning for Traffic Signal Control via Fourier Basis Approximation
crossref(2023)
Traffic signal control plans significantly impact transportation system efficiency at intersections. Adaptive plans that adjust to real-time conditions are more effective. Reinforcement learning (RL) adapts strategies based on environmental feedback, making it proficient in handling dynamic traffic scenarios. However, current RL methods have long computational periods, hindering their adoption for new scenarios. Another approach is to optimize the RL model itself for fast learning or make it transferable with learned experience. The underlying control algorithm should ensure convergence and minimize parameter sensitivity in diverse migration scenarios. We propose MetaSignal, an efficient meta-RL method for traffic signal control. Our approach uses Fourier basis as the value function approximation in RL, offering advantages like convergence facilitation, error bound achievement, and reduced parameter dependence. The model-agnostic meta-learning framework allows for effective adaptation to target scenarios with limited training cost. Empirical evaluation shows promising and stable performance in comprehensive experiments in synthetic and real-world traffic networks.
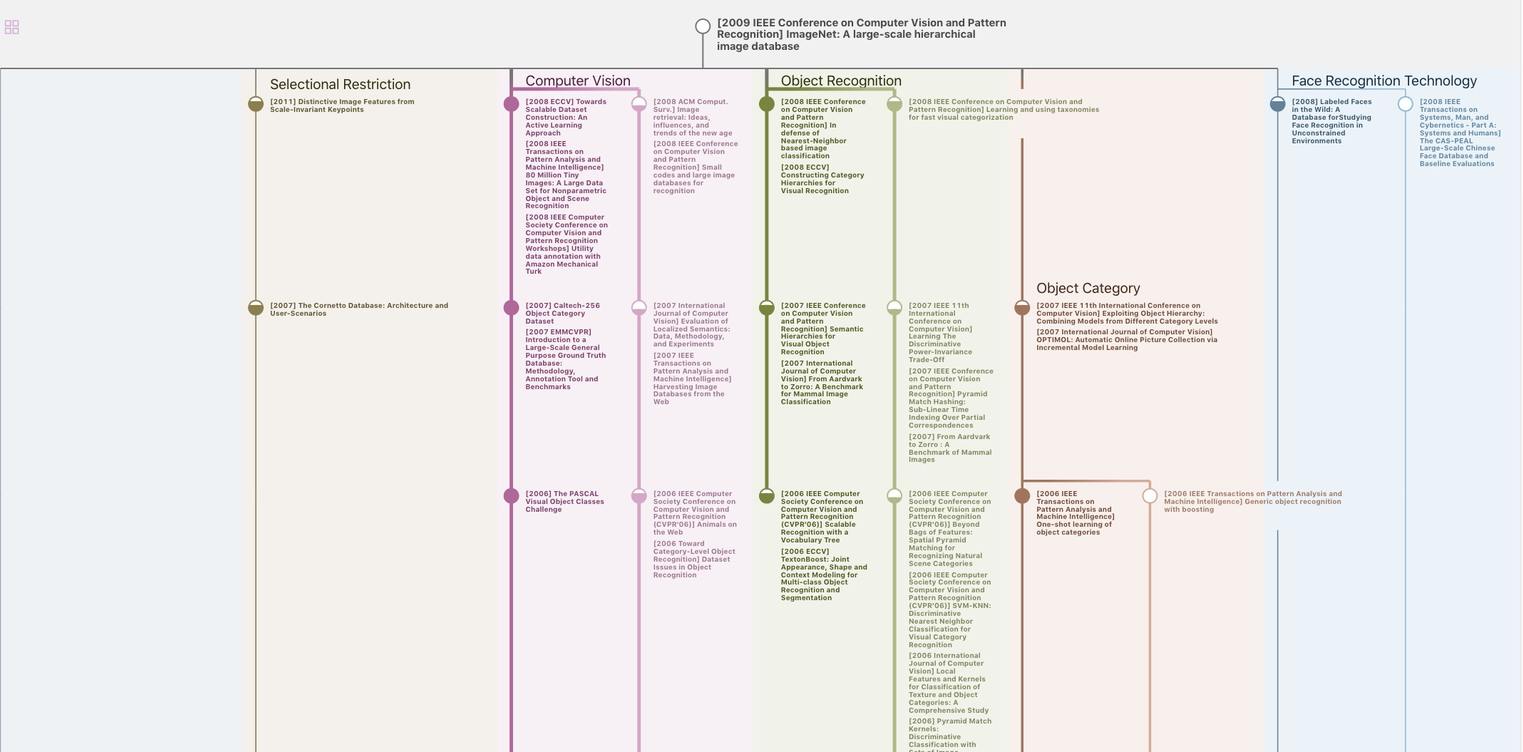