Quality-aware face alignment using high-resolution spatial dependencies
MULTIMEDIA TOOLS AND APPLICATIONS(2023)
摘要
Although CNN-based face alignment algorithms have got promising results. However, their alignment accuracy are still suffer from faces with severe occlusions and large poses, which mainly because (1) the inability to model long-range dependencies, construct effective face shape constraints and (2) the limitation on the size of the labeled facial datasets. To address the above problems, this study proposed a transformer-based data distillation semi-supervised face alignment algorithm. The transformer-based heatmap detection network introduces the transformer to model more efficient face shape constraint relationships, thus improving algorithm robustness under partial occlusion. Moreover, a quality-aware pseudolabeled sample distillation network is designed to help transformer obtain the CNNs inherent inductive biases by evaluating the quality of pseudolabeled data generated by transformer-based heatmap detection networks. This study also proposed intensive training strategy to use more unlabeled data without the need for manual operation to further improve the performance of transformer thermal map detection networks. Experimental results on the 300W, AFLW, and 300VW datasets demonstrate the superiority of our method over state-of-the-art face alignment methods.
更多查看译文
关键词
Face alignment,Transformer,Semi-supervised learning,Heatmap detection,Knowledge distillation
AI 理解论文
溯源树
样例
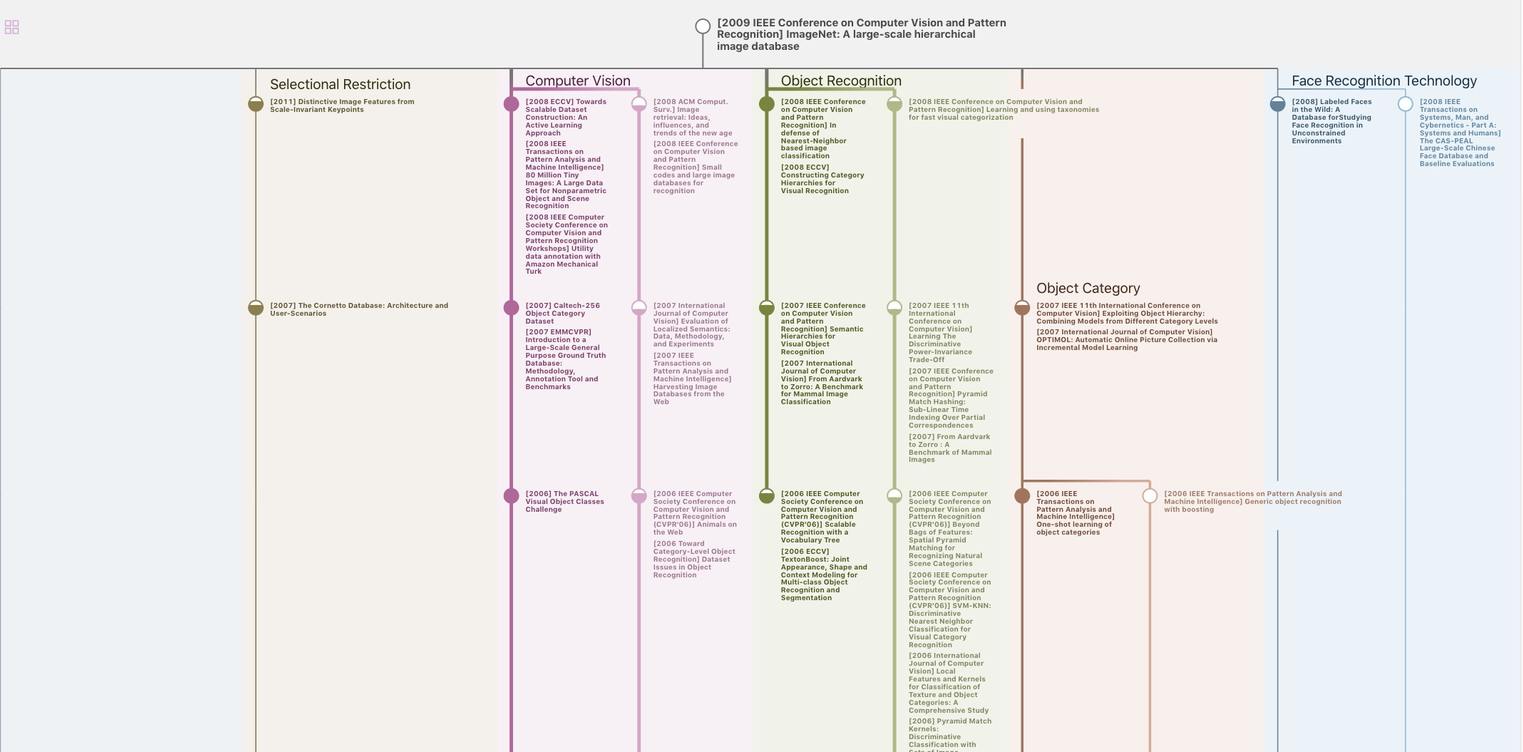
生成溯源树,研究论文发展脉络
Chat Paper
正在生成论文摘要