Comparing reinforcement learning algorithms for a trip building task: A multi-objective approach using non-local information.
Comput. Sci. Inf. Syst.(2024)
摘要
Using reinforcement learning (RL) to support agents in making decisions that consider more than one objective poses challenges. We formulate the problem of multiple agents learning how to travel from A to B as a reinforcement learning task modeled as a stochastic game, in which we take into account: (i) more than one objective, (ii) non-stationarity, (iii) communication of local and non-local information among the various actors. We use and compare RL algorithms, both for the single objective (Q-learning), as well as for multiple objectives (Pareto Q learning), with and without non-local communication. We evaluate these methods in a scenario in which hundreds of agents have to learn how to travel from their origins to their destinations, aiming at minimizing their travel times, as well as the carbon monoxide vehicles emit. Results show that the use of non-local communication reduces both travel time and emissions.
更多查看译文
关键词
reinforcement learning algorithms,reinforcement learning,trip,multi-objective,non-local
AI 理解论文
溯源树
样例
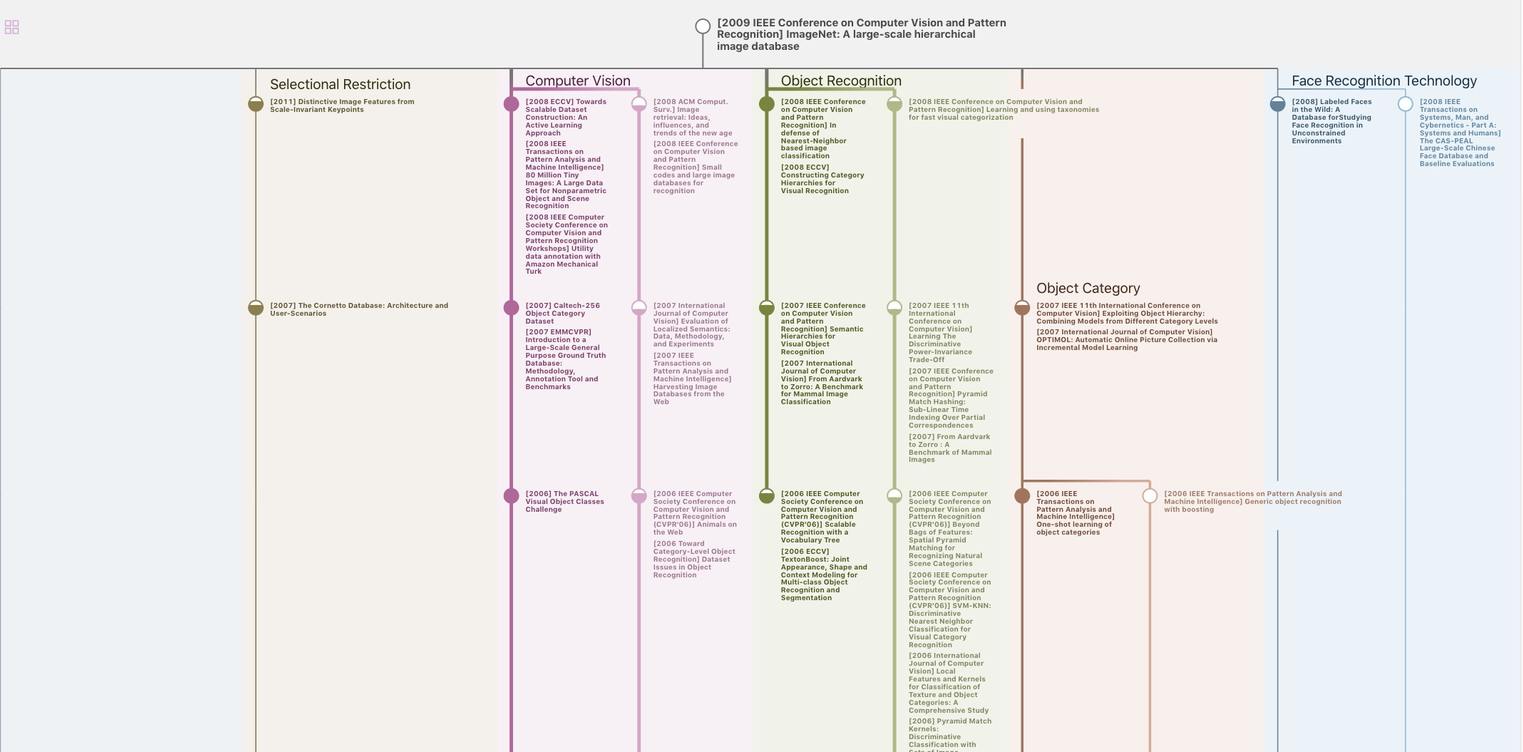
生成溯源树,研究论文发展脉络
Chat Paper
正在生成论文摘要