A novel adaptive class weight adjustment‐based multiclass segmentation error minimization technique for COVID ‐19 X‐ray image analysis
International Journal of Imaging Systems and Technology(2023)
摘要
AbstractCoronavirus outbreaks during the last couple of years created a huge health disaster for human lives. Diagnosis of COVID‐19 infections is, thus, very important for the medical practitioners. For a quick detection, analysis of the COVID‐19 chest X‐ray images is inevitable. Therefore, there is a strong need for the development of a multiclass segmentation method for the purpose. Earlier techniques used for multiclass segmentation of images are mostly based on entropy measurements. Nonetheless, entropy methods are not efficient when the gray‐level distribution of the image is nonuniform. To address this problem, a novel adaptive class weight adjustment‐based multiclass segmentation error minimization technique for COVID‐19 chest X‐ray image analysis is investigated. Theoretical investigations on the first‐hand objective functions are presented. The results on both the biclass and multiclass segmentation of medical images are enlightened. The key to our success is the adjustment of the pixel counts of different classes adaptively to reduce the error of segmentation. The COVID‐19 chest X‐ray images are taken from the Kaggle Radiography database for the experiments. The proposed method is compared with the state‐of‐the‐art methods based on Tsallis, Kapur's, Masi, and Rényi entropy. The well‐known segmentation metrics are used for an empirical analysis. Our method achieved a performance increase of around 8.03% in the case of PSNR values, 3.01% for FSIM, and 4.16% for SSIM. The proposed technique would be useful for extracting dots from micro‐array images of DNA sequences and multiclass segmentation of the biomedical images such as MRI, CT, and PET.
更多查看译文
关键词
novel adaptive class weight,segmentation,image analysis
AI 理解论文
溯源树
样例
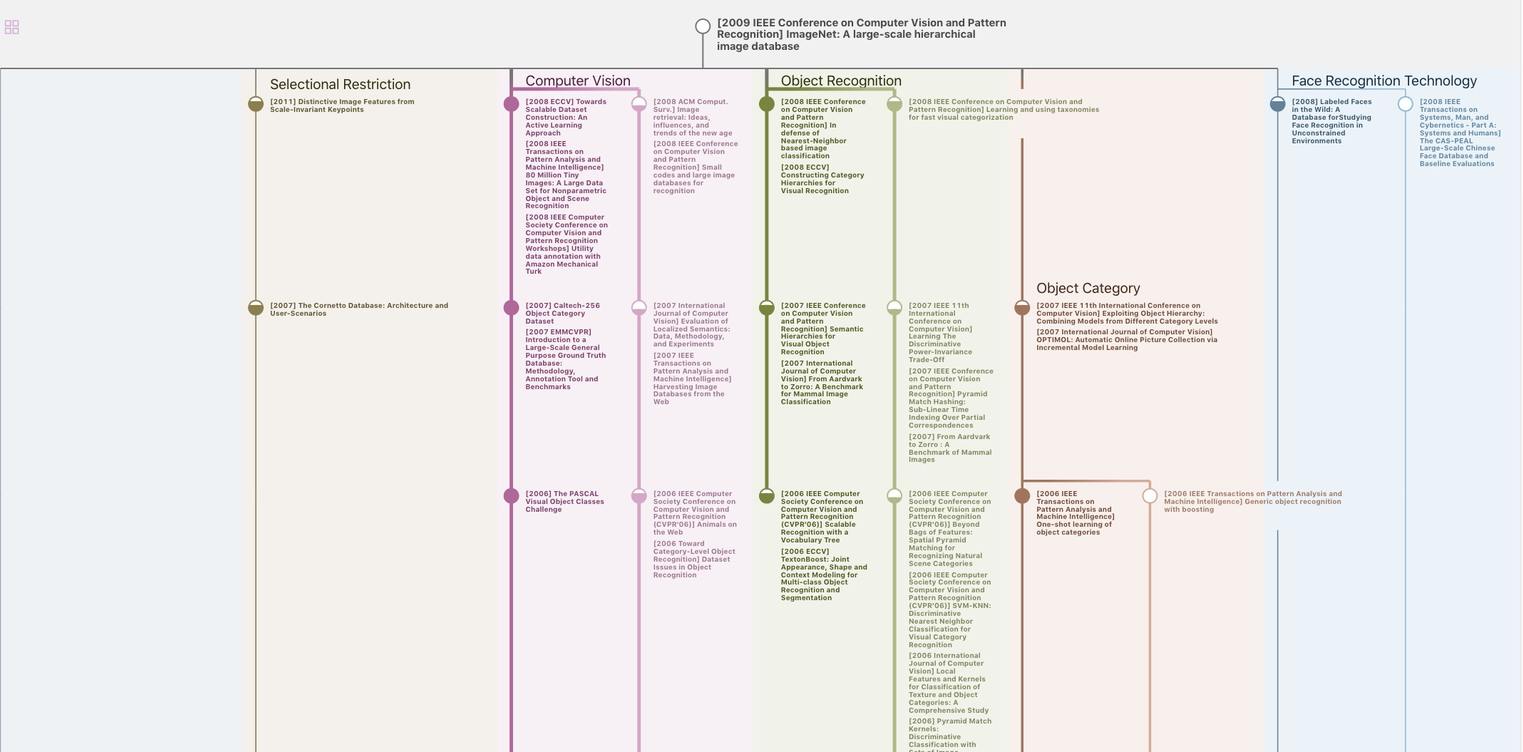
生成溯源树,研究论文发展脉络
Chat Paper
正在生成论文摘要