FM-Based Positioning via Deep Learning
crossref(2023)
Frequency modulation (FM) broadcast signals, as opportunity signals, hold significant potential for indoor and outdoor positioning applications. The existing FM-based positioning methods primarily rely on received signal strength (RSS) for positioning, the accuracy of which needs improvement. In this paper, we introduce an end-to-end FM-based positioning method that leverages deep learning, known as FM-Pnet. This method utilizes the time-frequency representation of FM signals as the network input, allowing the network to automatically learn deep features for positioning. We further propose two strategies, noise injection and enriching training samples, to enhance the model's generalization performance over long time spans. We construct datasets for both indoor and outdoor scenarios and conduct extensive experiments to validate the performance of our proposed method. Experimental results demonstrate that FM-Pnet significantly outperforms traditional RSS-based positioning methods in terms of both positioning accuracy and stability.
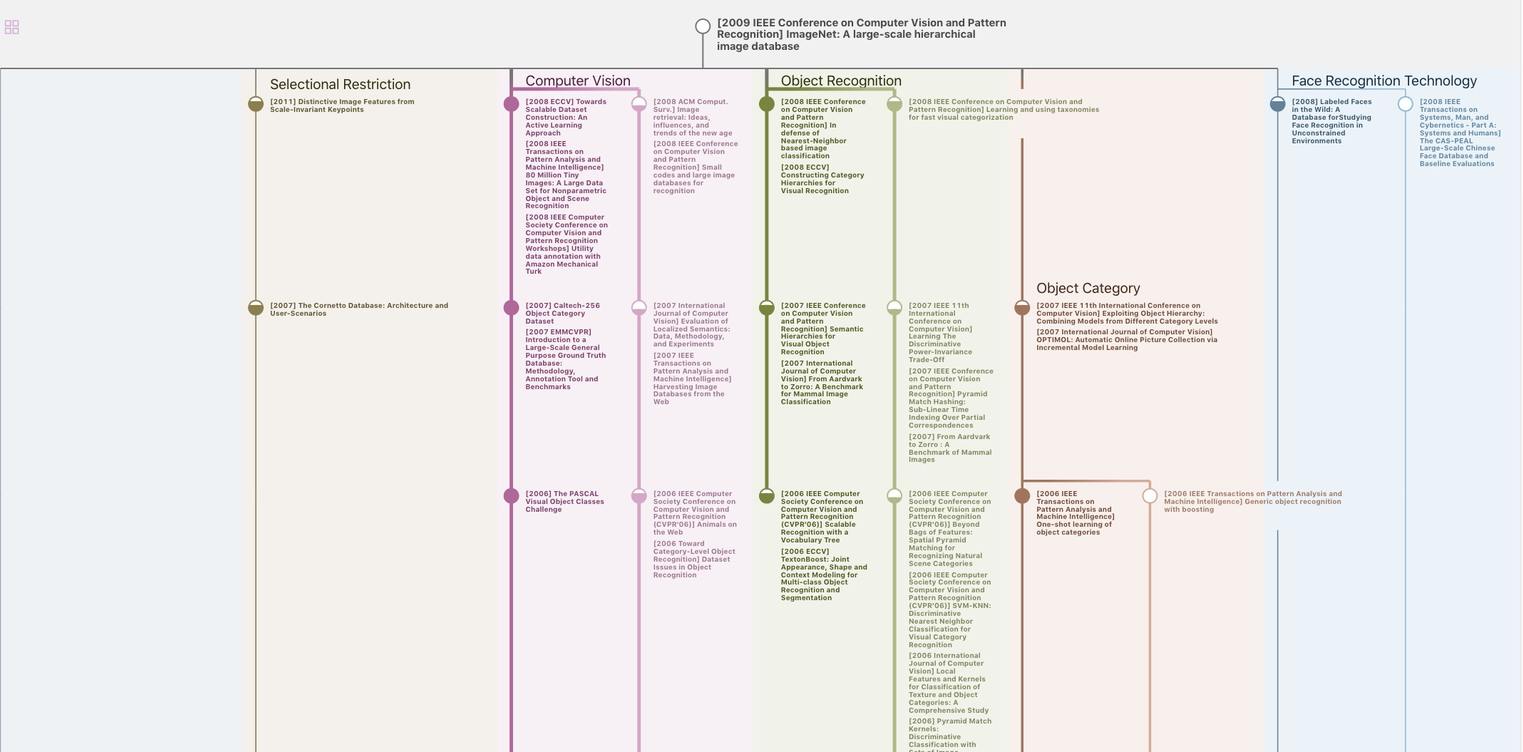