TNSAR: Temporal Evolution Network Embedding Based on Structural and Attribute Retention
IEEE TRANSACTIONS ON SERVICES COMPUTING(2023)
摘要
Network embedding (NE) focuses on mapping each node within a network into a condensed vector representation of lower dimensional, while simultaneously retaining the original network structure and attribute features as faithfully as possible. It applies to a wide range of tasks of network mining, including link prediction, node classification, user recommendation, etc. Most existing network embedding models primarily aim at static networks, which require node knowledge in the full-time span. However, various real-world network is dynamic, and the nodes in the network change frequently. Although dynamic network embedding has garnered attention from a limited number of researchers, their approach has been restricted to learning node representations in isolation, without considering the integration of higher-order topological structure and attribute features of the nodes. To better address the above problems, a Temporal Evolution Network Embedding Model based on Structural and Attributes Retention (TNSAR) is proposed. In summary, our contributions include Introducing the graph decomposition technique for capturing global higher-order topological features, including higher-order structure, a fusion of attribute and topological information in the Graph Convolutional Network (GCN) network for node representation, and the temporal preservation component. The experimental results validate the effectiveness of TNSAR in network embedding tasks, showcasing its superiority over baseline methods.
更多查看译文
关键词
Dynamic network embedding,graph network,link prediction,network mining
AI 理解论文
溯源树
样例
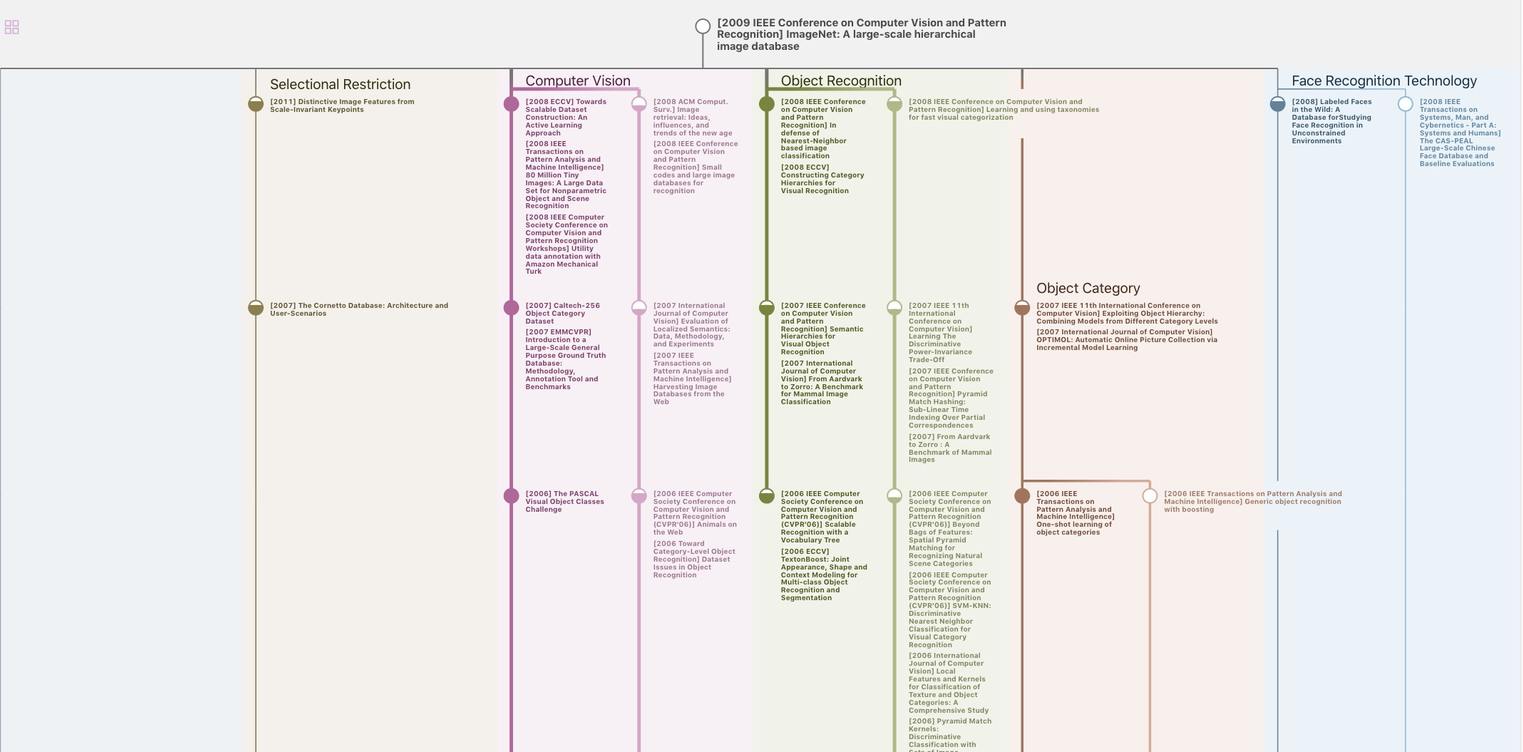
生成溯源树,研究论文发展脉络
Chat Paper
正在生成论文摘要