GCEN: Multi-Agent Deep Reinforcement Learning With Grouped Cognitive Feature Representation
IEEE Transactions on Cognitive and Developmental Systems(2023)
摘要
In recent years, cooperative Multi-Agent Deep Reinforcement Learning (MADRL) has received increasing research interest and has been widely applied to computer games and coordinated multi-robot systems, etc. However, it is still challenging to realize high solution quality and learning efficiency for MADRL under the conditions of incomplete and noisy observations. To this end, this paper proposes a multi-agent deep reinforcement learning approach with Grouped Cognitive featurE representatioN (GCEN), following the paradigm of Centralized Training and Decentralized Execution (CTDE). Different from previous works, GCEN incorporates a new grouped cognitive feature representation that combines a grouped attention mechanism and a training approach using mutual information. The grouped attention mechanism is proposed to selectively extract entity features within the observation field for each agent while avoiding the influence of irrelevant observations. The mutual information regularization term is designed to guide the agents to learn grouped cognitive features based on global information, aiming to mitigate the influence of partial observations. The proposed GCEN approach can be extended as a feature representation module to different MADRL methods. Extensive experiments on the challenging level-based foraging and StarCraft II micromanagement benchmarks were conducted to illustrate the effectiveness and advantages of the proposed approach. Compared with seven representative MADRL algorithms, our proposed approach achieves state-of-the-art performance in winning rates and training efficiency. Experiments results further demonstrate that GCEN has improved generalization ability across varying sight ranges.
更多查看译文
关键词
Cooperative multi-agent reinforcement learning,Attention mechanism,Cognitive feature extraction,Deep learning
AI 理解论文
溯源树
样例
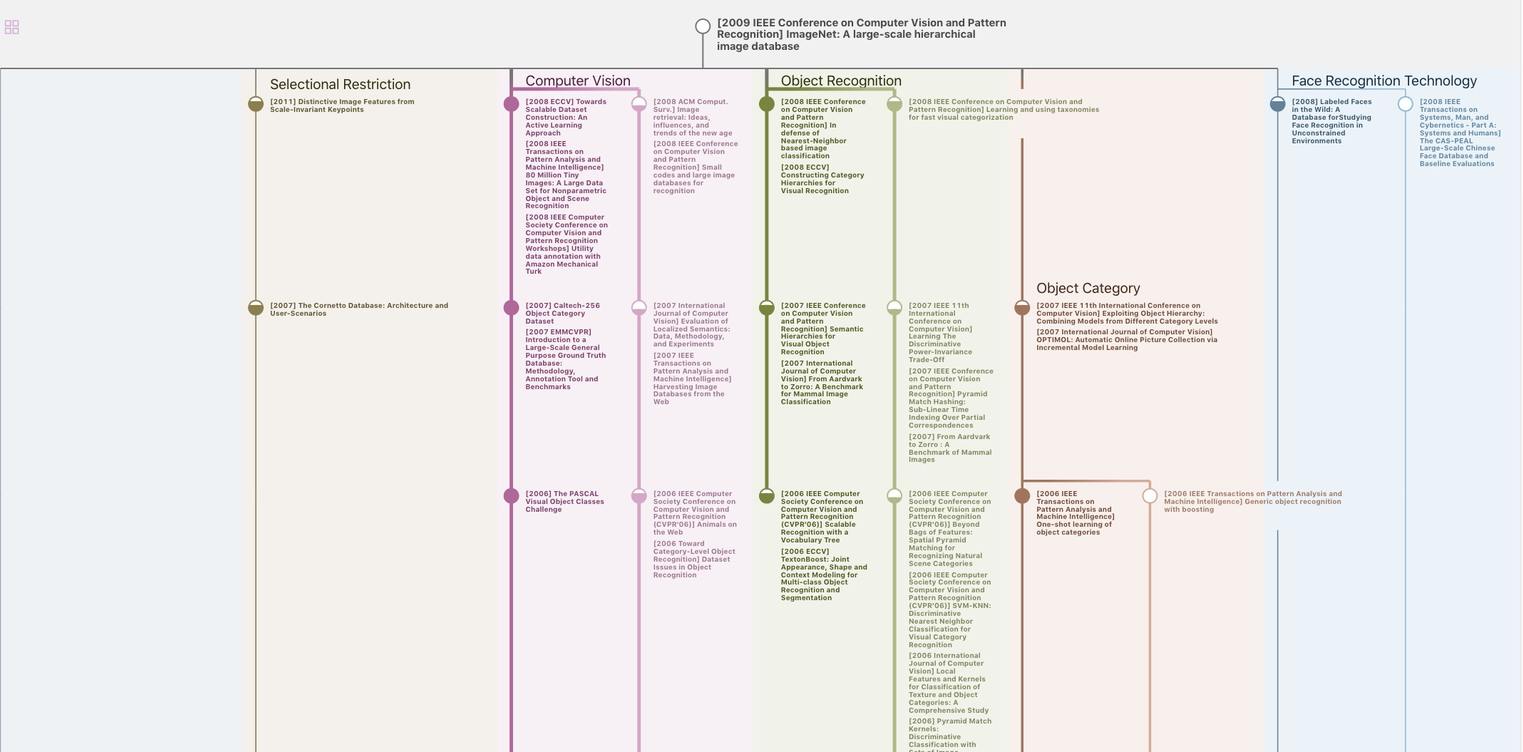
生成溯源树,研究论文发展脉络
Chat Paper
正在生成论文摘要