A Fabric Defect Segmentation Model Based on Improved Swin-Unet with Gabor Filter
Applied Sciences(2023)
摘要
Fabric inspection is critical in fabric manufacturing. Automatic detection of fabric defects in the textile industry has always been an important research field. Previously, manual visual inspection was commonly used; however, there were drawbacks such as high labor costs, slow detection speed, and high error rates. Recently, many defect detection methods based on deep learning have been proposed. However, problems need to be solved in the existing methods, such as detection accuracy and interference of complex background textures. In this paper, we propose an efficient segmentation algorithm that combines traditional operators with deep learning networks to alleviate the existing problems. Specifically, we introduce a Gabor filter into the model, which provides the unique advantage of extracting low-level texture features to solve the problem of texture interference and enable the algorithm to converge quickly in the early stages of training. Furthermore, we design a U-shaped architecture that is not completely symmetrical, making model training easier. Meanwhile, multi-stage result fusion is proposed for precise location of defects. The design of this framework significantly improves the detection accuracy and effectively breaks through the limitations of transformer-based models. Experimental results show that on a dataset with one class, a small amount of data, and complex sample background texture, our method achieved 90.03% and 33.70% in ACC and IoU, respectively, which is almost 10% higher than other previous state of the art models. Experimental results based on three different fabric datasets consistently show that the proposed model has excellent performance and great application potential in the industrial field.
更多查看译文
关键词
defect detection, fabric defect segmentation, Swin UNet, neural network
AI 理解论文
溯源树
样例
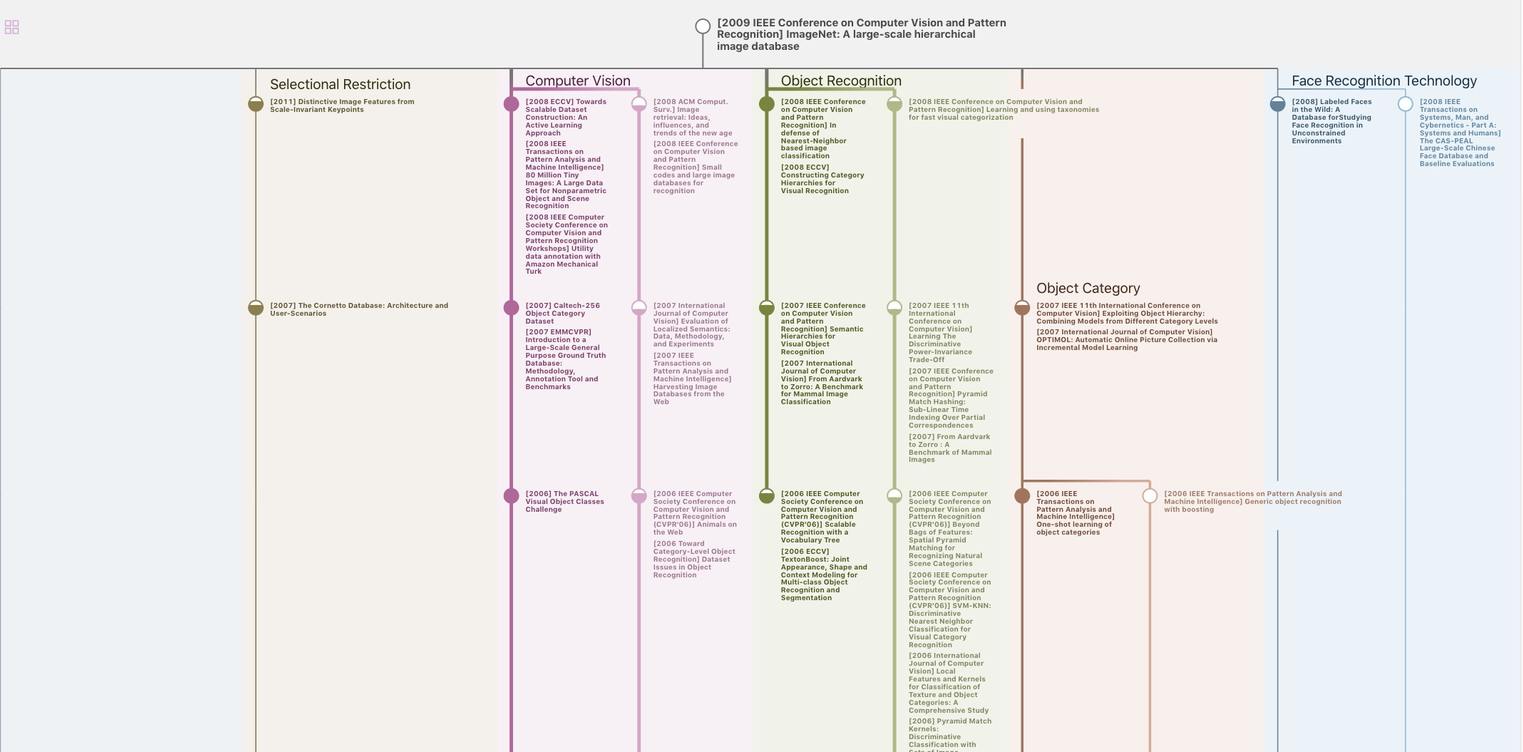
生成溯源树,研究论文发展脉络
Chat Paper
正在生成论文摘要