Differentiable visual computing for inverse problems and machine learning
Nature Machine Intelligence(2023)
摘要
Modern 3D computer graphics technologies are able to reproduce the dynamics and appearance of real-world environments and phenomena, building on theoretical models in applied mathematics, statistics and physics. These methods are applied in architectural design and visualization, biological imaging and visual effects. Differentiable methods, instead, aim to determine how graphics outputs (that is, the real-world dynamics or appearance) change when the environment changes. We survey this growing body of work and propose a holistic and unified differentiable visual computing pipeline. Differentiable visual computing can be leveraged to efficiently solve otherwise intractable problems in physical inference, optimal control, object detection and scene understanding, computational design, manufacturing, autonomous vehicles and robotics. Any application that can benefit from an understanding of the underlying dynamics of the real world stands to benefit substantially from a differentiable graphics treatment. We draw parallels between the well-established computer graphics pipeline and a unified differentiable graphics pipeline, targeting consumers, practitioners and researchers. The breadth of fields that these pipelines draw upon—and are of interest to—includes the physical sciences, data sciences, vision and graphics, machine learning, and adjacent mathematical and computing communities. Traditionally, 3D graphics involves numerical methods for physical and virtual simulations of real-world scenes. Spielberg et al. review how deep learning enables differentiable visual computing, which determines how graphics outputs change when the environment changes, with applications in areas such as computer-aided design, manufacturing and robotics.
更多查看译文
AI 理解论文
溯源树
样例
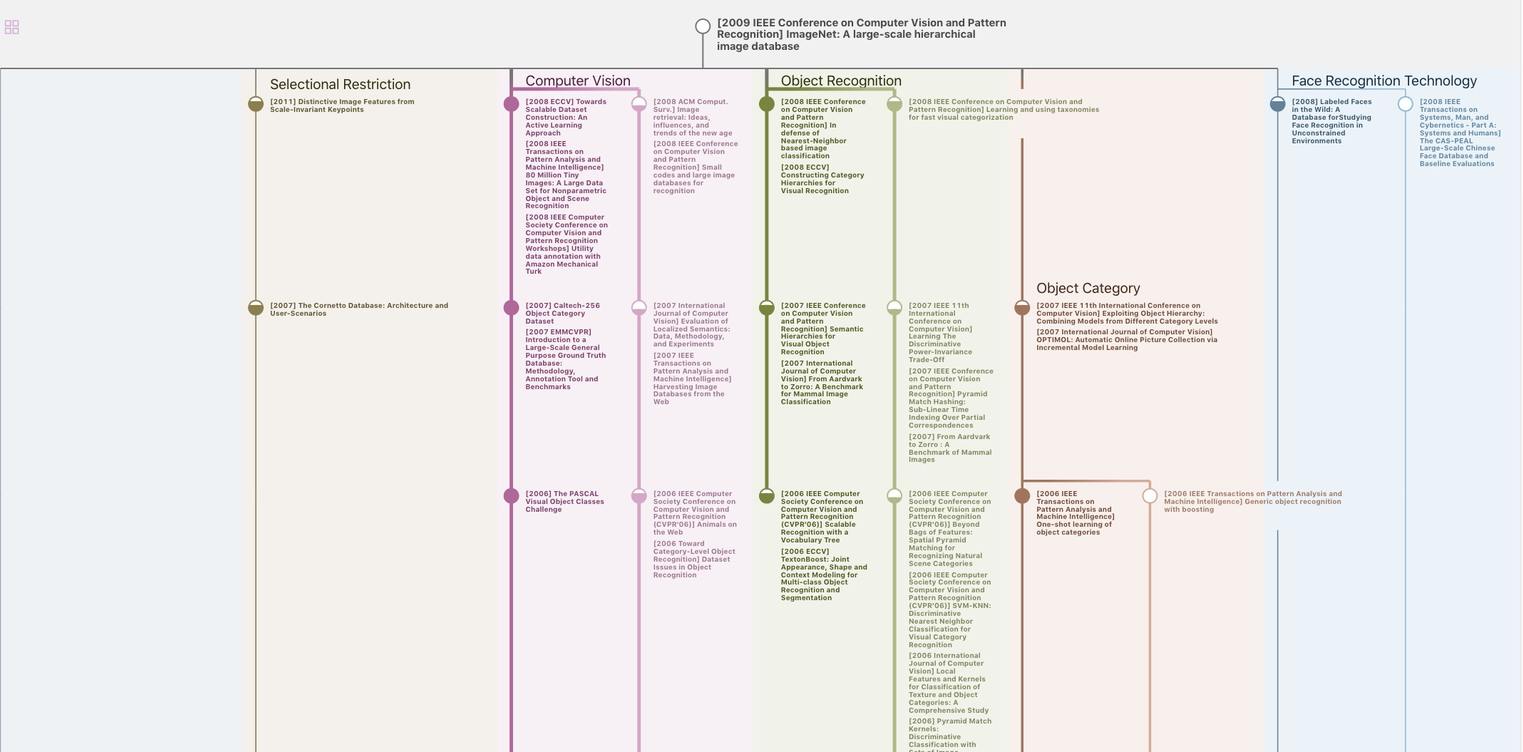
生成溯源树,研究论文发展脉络
Chat Paper
正在生成论文摘要