Machining surface roughness detection by adaptive deep fusion capsule network with low illumination and noise robustness
MEASUREMENT SCIENCE AND TECHNOLOGY(2024)
摘要
Surface roughness measurement is of great importance for monitoring machining quality. To address the performance degradation of deep models caused by low illumination and ambient noise, this study proposes a non-contact surface roughness detection method based on an adaptive deep fusion capsule network (ADFCNet) model. Firstly, principal competent analysis-based color image enhancement is employed to augment initial surface images collected from normal illumination. Then, consisting of a deep multi-model fusion for high-level feature representation and a capsule classifier for roughness recognition, the ADFCNet model is designed to detect roughness grades by using workpiece surface images. The key hyperparameters of the model are automatically determined by a genetic algorithm. Finally, laboratory and factory experiments under low illumination are carried out to validate the effectiveness and superiority of the proposed method. Experimental results demonstrate that the proposed method has strong low-illumination and noise robustness and generalization capability, indicating a better application prospect in actual scenarios.
更多查看译文
关键词
surface roughness detection,deep learning,capsule network,hyperparameter automated tuning,low illumination
AI 理解论文
溯源树
样例
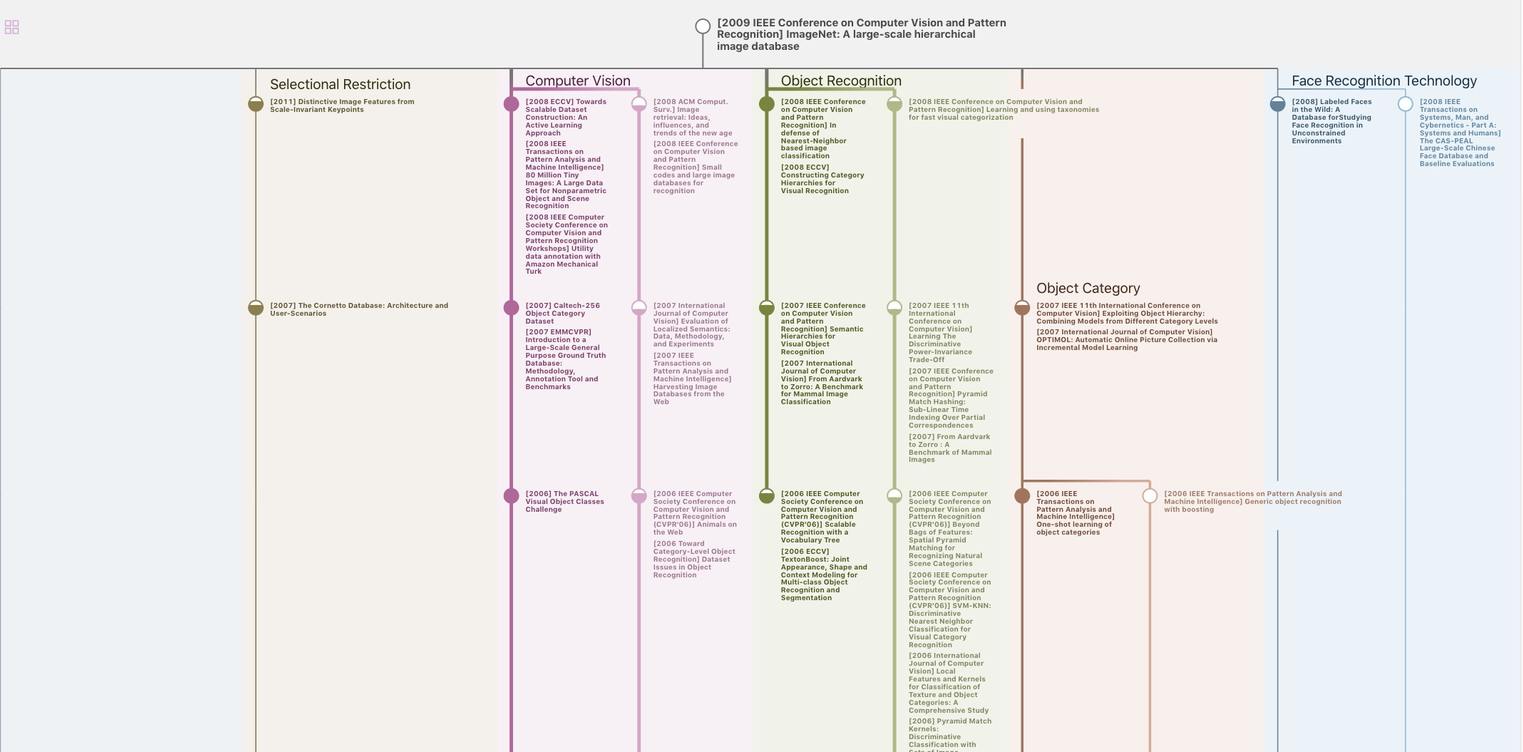
生成溯源树,研究论文发展脉络
Chat Paper
正在生成论文摘要