Life course analysis using deep learning approaches: a simulation study
crossref(2023)
摘要
Abstract Life course theory seeks to explain the cause of negative health outcomes in an individual’s life through intricate interactions between different exposure events. The methodology used in life course theory requires longitudinal cohort data, but these data are invariably sparse due to a combination of rare exposure events and high dimensionality. Traditionally these problems have been analysed using group-based trajectory approaches or structural equation models. These methods depend on assumptions about the nature of the connections between different exposures and the outcome limiting their complexity and, therefore, model accuracy. Here, we explore the utility of deep learning (DL) approaches to capture complexities both across variables and through time. We hypothesise that these reflect a more accurate mathematical description of life course theory. We conducted an extensive synthetic study where data are simulated from representative life course patterns drawn from the literature. We find that DL methods can accurately detect complex patterns in time that baseline linear models cannot. We find that while these DL models perform worse with noisy data, they are still reliable in detecting outcomes generated from complex time series patterns. Our analysis suggests that DL approaches may be a suitable null model for studying life course theory.
更多查看译文
AI 理解论文
溯源树
样例
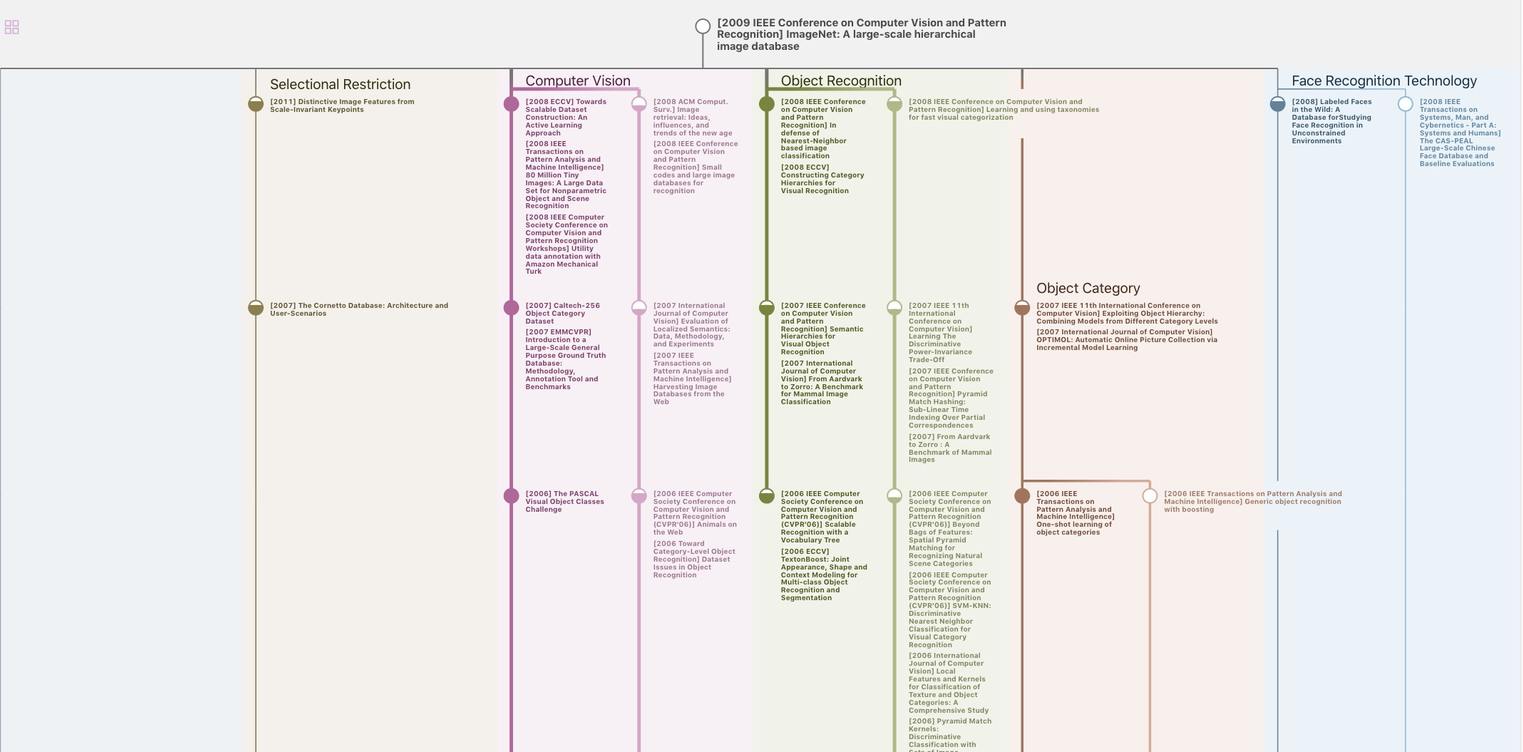
生成溯源树,研究论文发展脉络
Chat Paper
正在生成论文摘要