Rolling bearing fault diagnosis method based on MTF-MFACNN
MEASUREMENT SCIENCE AND TECHNOLOGY(2024)
摘要
A rolling bearing fault diagnosis method based on the Markov transition field (MTF) and multi-scale feature aggregation convolutional neural network (MFACNN) is proposed to address the problems of excessive parameter number, slow training speed, and insufficient generalization of traditional CNNs. Firstly, the original vibration signal is input into the MTF and converted into two-dimensional images with time correlation. Then, in order to effectively aggregate feature information at different scales and levels, a MFA module is presented to capture rich information from feature maps at different scales and assign different weights to these features for fusion. Secondly, while ensuring the lightweight of the model, utilizing feature information of different resolutions, a lightweight feature fusion module is put forward to fuse multiple feature maps together to improve the performance and efficiency of the model. On this basis, an MFACNN model is constructed. Finally, the two-dimensional images are input into MTF-MFACNN and experimentally validated using two different datasets. The results show that the proposed method has faster calculation speed, higher fault recognition accuracy, and stronger generalization performance compared to other methods.
更多查看译文
关键词
fault diagnosis,multi-scale feature aggregation,lightweight feature fusion,rolling bearings
AI 理解论文
溯源树
样例
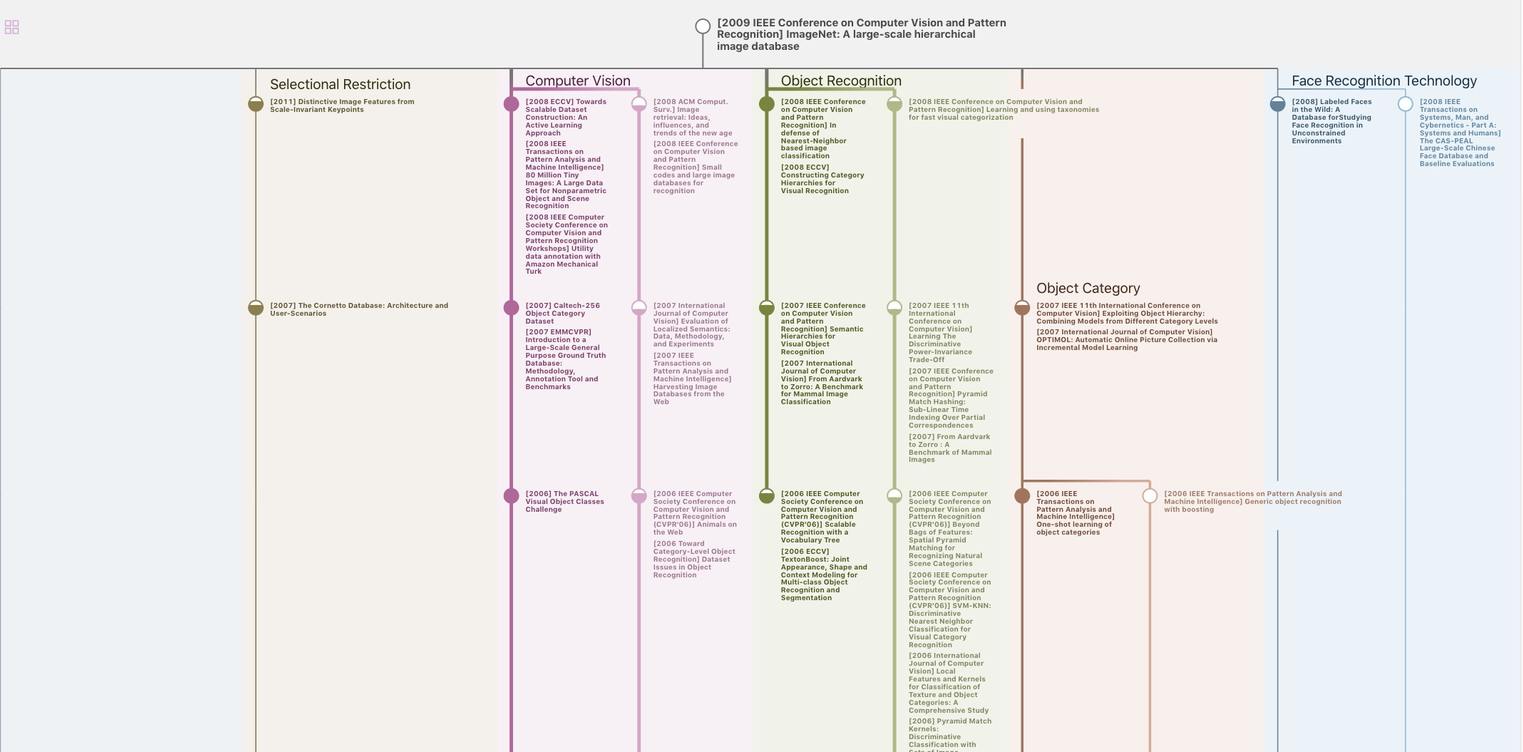
生成溯源树,研究论文发展脉络
Chat Paper
正在生成论文摘要