AMoDeBic: An adaptive Multi-objective Differential Evolution biclustering algorithm of microarray data using a biclustering binary mutation operator
EXPERT SYSTEMS WITH APPLICATIONS(2024)
摘要
In bioinformatics, biclustering is a crucial optimization task that can reveal hidden patterns and identify groups of genes that behave similarly under certain conditions. This study aims to efficiently identify high-quality and cohesive biclusters that share common characteristics across two data dimensions. To achieve this, we propose the first biclustering approach that utilizes Multi-objective Differential Evolution (DE), which is a novel technique for gene group discovery. Additionally, we introduce the Biclustering Binary Differential Evolution (BBDE), a new mutation operator that combines node addition and deletion, guided by an adaptive factor F. We thoroughly tested our method's effectiveness taking into account biological relevance, noise, overlap resistance, and statistics. We compared our results to state of the art algorithms using both synthetic and real datasets like Yeast Cell Cycle, Saccharomyces cerevisiae, and Human B Cell. Our algorithms outperformed the comparisons and effectively identified significant biclusters.
更多查看译文
关键词
Differential Evolution algorithms,Multi-objective Differential evolution,Biclustering,Evolutionary algorithms,Micro-array data,Knowledge discovery
AI 理解论文
溯源树
样例
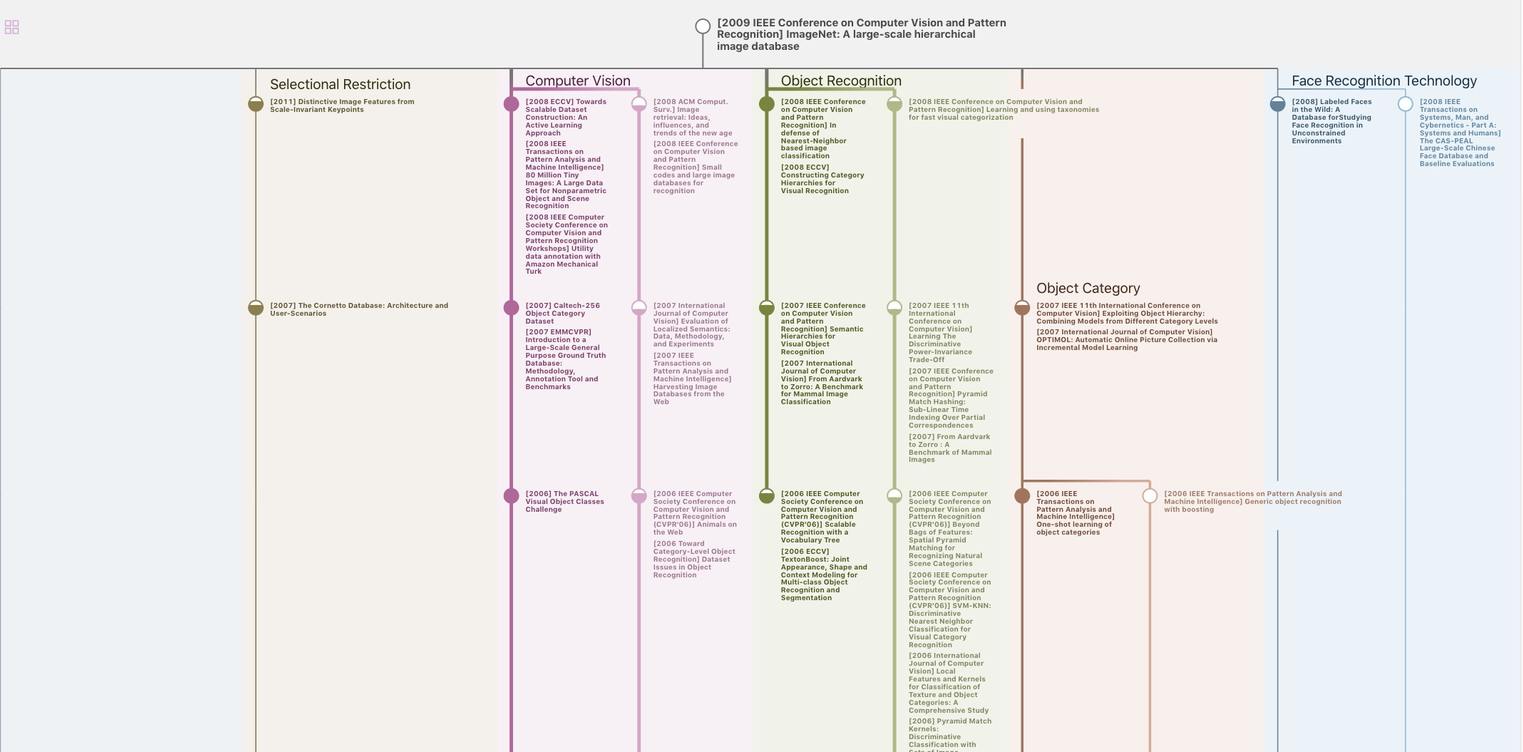
生成溯源树,研究论文发展脉络
Chat Paper
正在生成论文摘要