Key factors for species distribution modeling in benthic marine environments
FRONTIERS IN MARINE SCIENCE(2023)
摘要
Species distribution modeling is a widely used technique for estimating the potential habitats of target organisms based on their environmental preferences. These methods serve as valuable tools for resource managers and conservationists, and their utilization is increasing, particularly in marine environments where data limitations persist as a challenge. In this study, we employed the global distribution predictions of six cold-water coral species as a case study to investigate various factors influencing predictions, including modeling algorithms, background points sampling strategies and sizes, and the collinearity of environmental datasets, using both discriminative and functional performance metrics. The choice of background sampling method exhibits a stronger influence on model performance compared to the effects of modeling algorithms, background point sampling size, and the collinearity of the environmental dataset. Predictions that utilize kernel density backgrounds, maintain an equal number of presences and background points for algorithms of BRT, RF, and MARS, and employ a substantial number of background points for MAXENT, coupled with a collinearity-filtered environmental dataset in species distribution modeling, yield higher levels of discriminative and functional performance. Overall, BRT and RF outperformed MAXENT, a conclusion that is further substantiated by the analysis of smoothed residuals and the uncertainty associated with the predicted habitat suitability of Madrepora oculata. This study offers valuable insights for enhancing species distribution modeling in marine benthic environments, thereby benefiting resource management and conservation strategies for benthic species.
更多查看译文
关键词
species distribution modeling,benthic marine environments,modeling algorithms,collinearity,presence-only,background points,model comparison,model evaluation
AI 理解论文
溯源树
样例
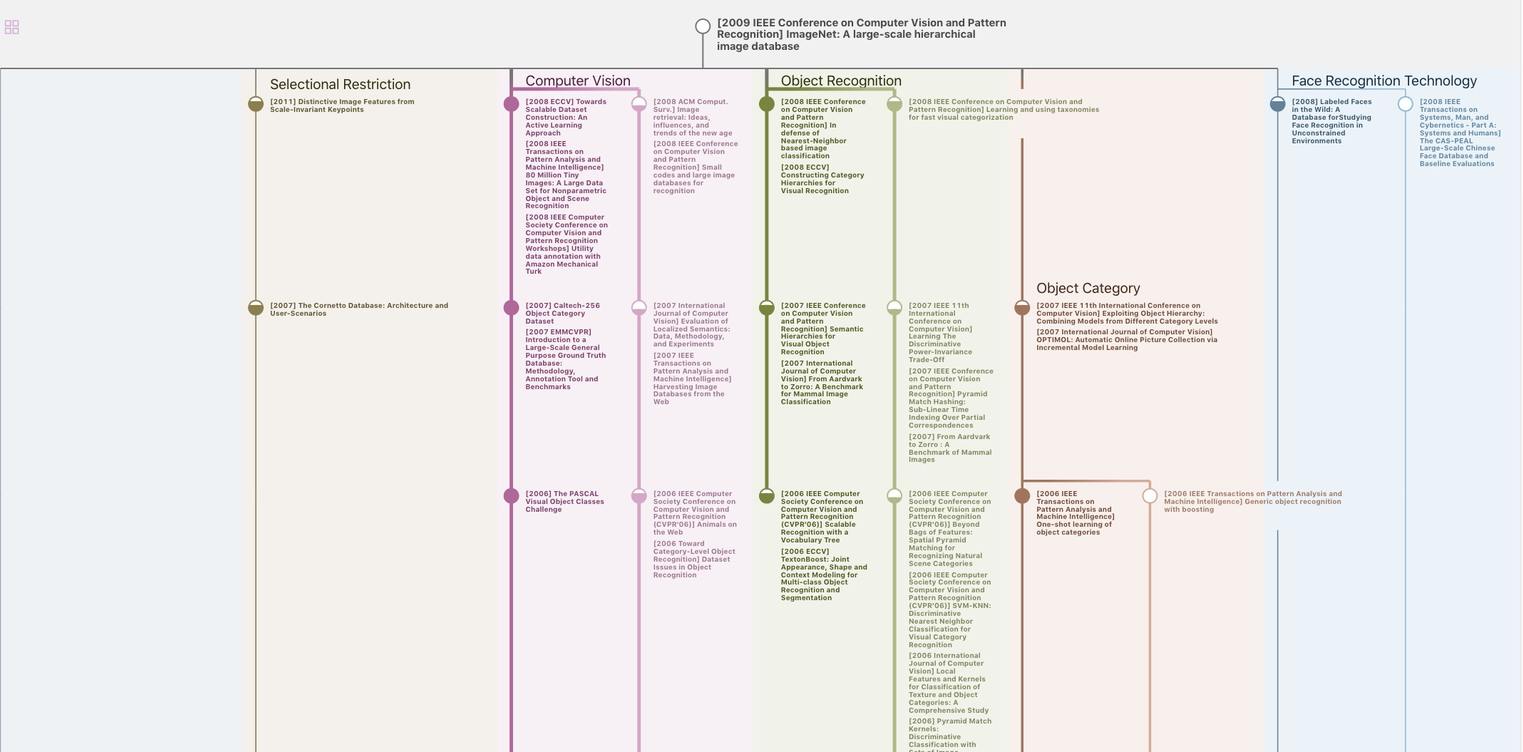
生成溯源树,研究论文发展脉络
Chat Paper
正在生成论文摘要