Deep-learning statistical downscaling of precipitation in the middle reaches of the Yellow River: A Residual in Residual Dense Block based network
crossref(2023)
摘要
Abstract. The middle reaches of the Yellow River (MRYR), located in northern China, are the transition zone between semi-arid and semi-humid climates. As one of the climate-sensitive regions in China, MRYR has fragile ecological environment and serious soil loss, which leads to geological disasters such as landslides, collapses and mudslides caused by extreme precipitation to occur. However, scarceness of the high-resolution precipitation data over MRYR limits the assessment of the environmental impacts caused by climate change, especially for extreme precipitation. In this paper, we design a Residual in Residual Dense Block based network (RRDBNet) model for the statistical downscaling of precipitation in MRYR, and compare the proposed RRDBNet with the generalized linear regression model and two popular deep learning-based models. The results show that the proposed RRDBNet model has a good performance on precipitation simulations, which can well reproduce the spatial-temporal characteristics of high-resolution precipitation. Especially, RRDBNet has substantial improvements in extreme precipitation compared with other models. On the probability density function of daily precipitation, it is further demonstrated that RRDBNet performs better on extreme precipitation frequency. Our results suggest that the statistical downscaling based on RRDBNet may be an effective tool for historical and future climate simulations from global climate models.
更多查看译文
AI 理解论文
溯源树
样例
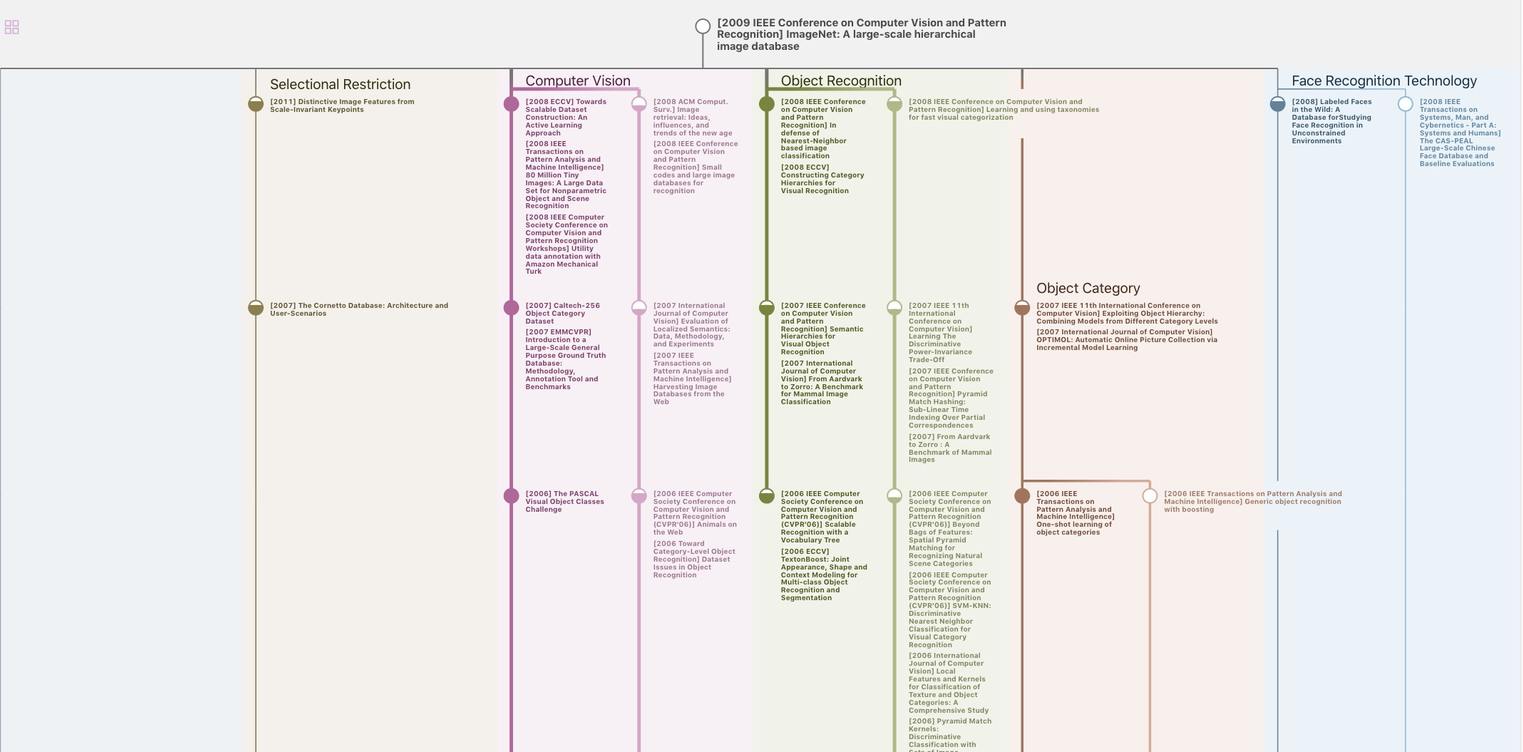
生成溯源树,研究论文发展脉络
Chat Paper
正在生成论文摘要