Multi-feature spatial distribution alignment enhanced domain adaptive method for tool condition monitoring
Eksploatacja i Niezawodność – Maintenance and Reliability(2023)
摘要
Transfer learning (TL) has been successfully implemented in tool condition monitoring (TCM) to address the lack of labeled data in real industrial scenarios. In current TL models, the domain offset in the joint distribution of input feature and output label still exists after the feature distribution of the two domains is aligned, resulting in performance degradation. A multiple feature spatial distribution alignment (MSDA) method is proposed, Including Correlation alignment for deep domain adaptation (Deep CORAL) and Joint maximum mean difference (JMMD). Deep CORAL is employed to learn nonlinear transformations, align source and target domains at the feature level through the second order statistical correlations. JMMD is applied to improve domain alignment by aligning the joint distribution of input features and output labels. ResNet18 combining with bidirectional short-term memory network and attention mechanism is developed to extract the invariant features. TCM experiments with four transfer tasks were conducted and demonstrated the effectiveness of the proposed method.
更多查看译文
关键词
condition monitoring,Transfer learning,correlation alignment,joint maximum mean difference,feature extractor
AI 理解论文
溯源树
样例
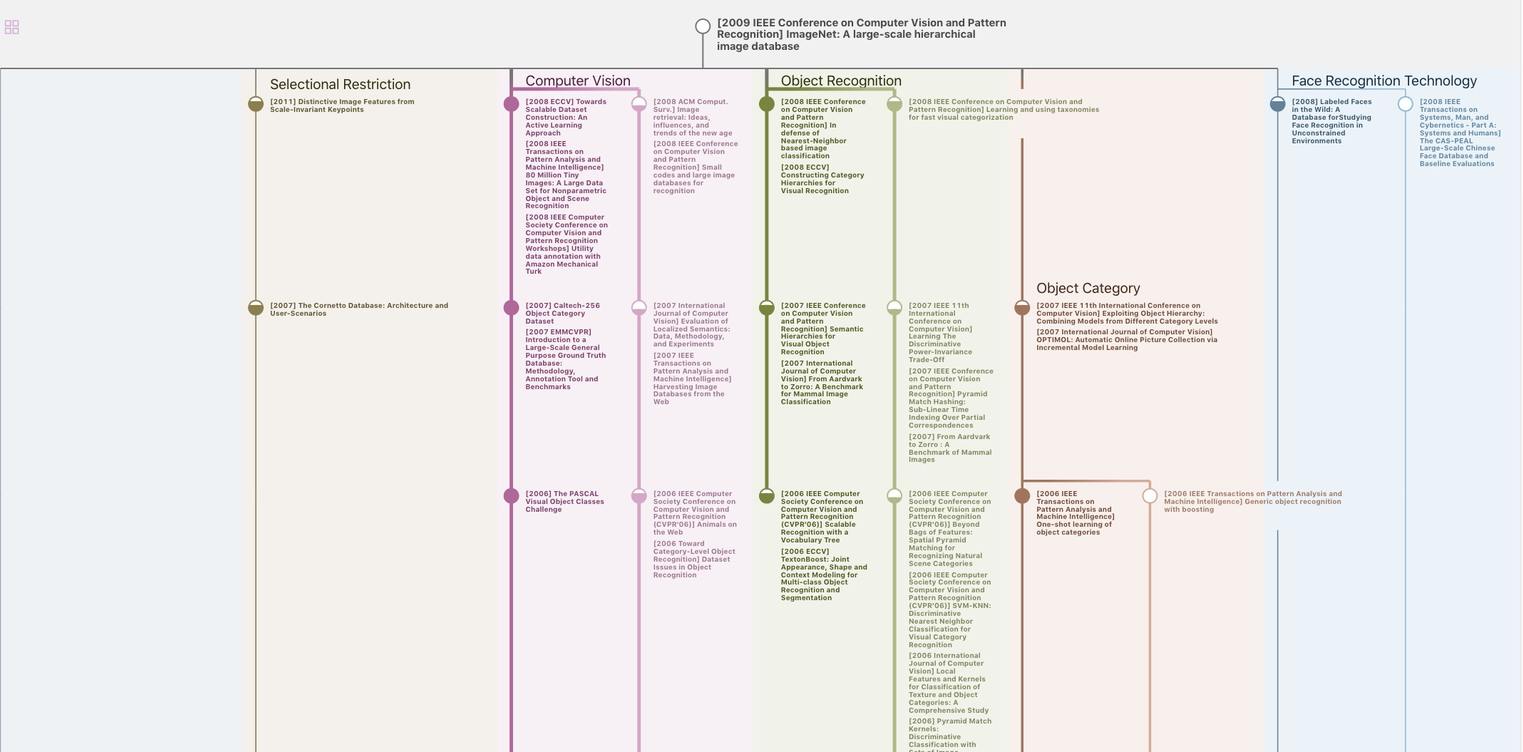
生成溯源树,研究论文发展脉络
Chat Paper
正在生成论文摘要