MVRA-UNet: Multi-View Residual Attention U-Net for Precise Defect Segmentation on Magnetic Tile Surface
IEEE ACCESS(2023)
摘要
Magnetic tile defect segmentation plays a vital role in magnet motor production. However, it is difficult to detect defect areas from the magnetic tile because of these issues: 1) There is low contrast between the defects regions and the normal ones on the magnetic tile surface; 2) The defects on the magnetic tile vary greatly, and the small defects are always masked by complex backgrounds. To tackle these problems, a Multi-View Residual Attention U-Net (MVRA-UNet) is proposed for defect segmentation from magnetic tile surface, where the Gaussian Residual Attention Convolution (GRAC) module is designed to distinguish similar feature defects in low contrast environments by modeling discriminative feature representation; then, the Multi-View Cycle Convolution (MVCC) module is designed to segment the tiny defects from background noise by learning multi-view (i.e., x, y, and z-axis) feature maps. Experiments conducted on the benchmark demonstrate the superiority of the MVRA-UNet, achieving an excellent performance of 87.7% on the Intersection over Union (IoU), which is better than the state-of-the-art approaches.
更多查看译文
关键词
Magnetic resonance imaging,Convolutional neural networks,Image segmentation,Feature extraction,Semantics,Object detection,Magnetic analysis,Gaussian processes,Surface cracks,Surface defect segmentation,multi-view learning,residual attention,Gaussian normalization
AI 理解论文
溯源树
样例
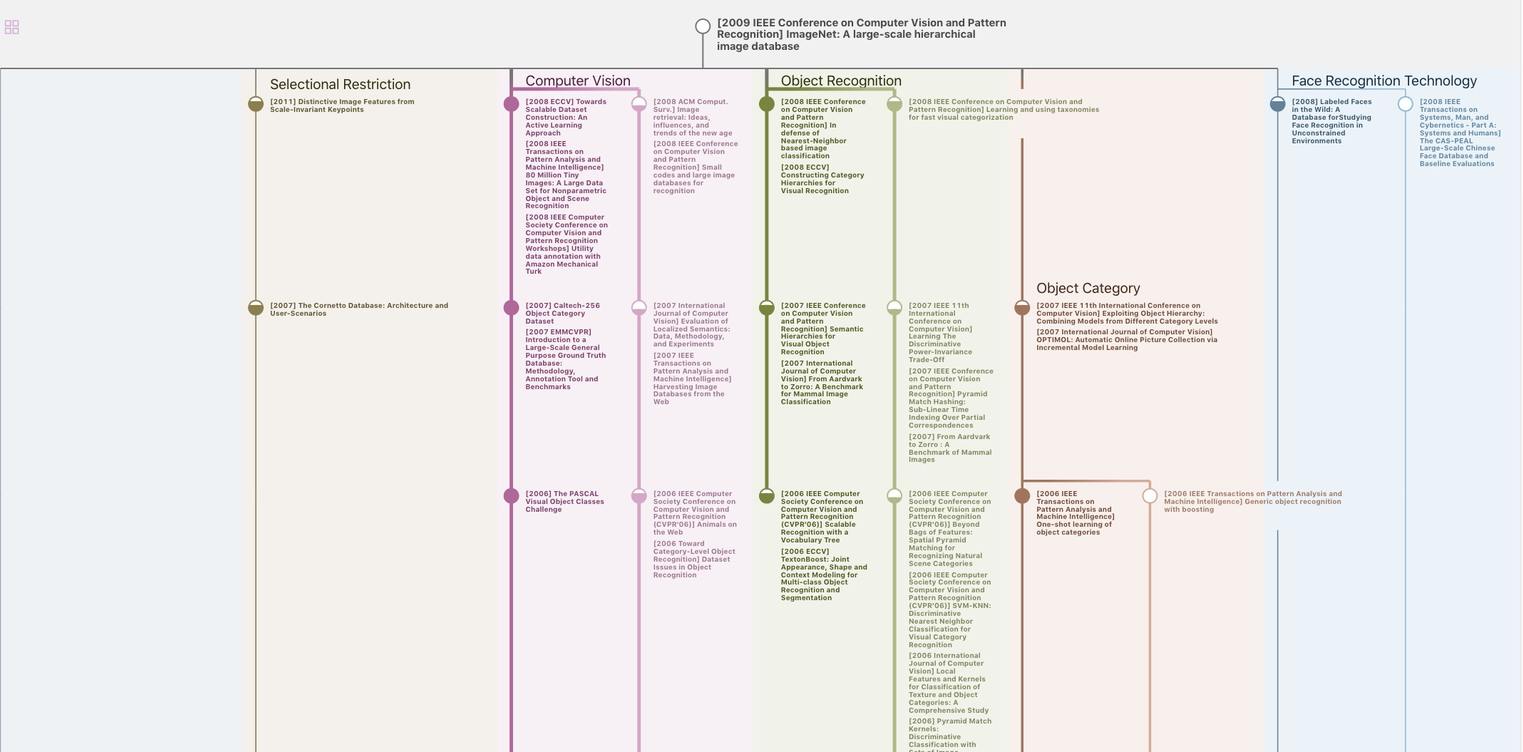
生成溯源树,研究论文发展脉络
Chat Paper
正在生成论文摘要