Joint Optimization Design of RIS-Assisted Hybrid FSO SAGINs Using Deep Reinforcement Learning
IEEE TRANSACTIONS ON VEHICULAR TECHNOLOGY(2024)
摘要
Satellite-aerial-ground integrated networks (SAGINs) have emerged as a promising infrastructure for next-generation wireless networks. Considering the fading effects of obstacles over longer transmission distances, in this article, we equip reconfigurable intelligent surfaces (RIS) on the unmanned aerial vehicle (UAV) to reflect the uplink signals from the ground vehicle transmitter (VT) to high altitude platform (HAP). The HAP then acts as a relay to forward the signals to satellites through hybrid free space optics (FSO) links. This article focuses on optimizing the system ergodic sum rate in SAGINs. To address the high-dimensional non-convex optimization problems, an asymmetric long short-term memory (LSTM)-deep deterministic policy gradient (DDPG) algorithm, called AL-DDPG, is proposed. The AL-DDPG algorithm leverages hidden states in partially observable Markov states using a memory inference method to address the training convergence problem. Numerical results demonstrate the superiority of the AL-DDPG algorithm, with a 16% improvement in the system sum rate compared to the traditional optimization algorithm. Besides, we provide insights into the impacts of various factors on system performance.
更多查看译文
关键词
Satellite-aerial-ground integrated networks (SAGINs),hybrid FSO/RF links,reconfigurable intelligent surface (RIS),long short-term memory-deep deterministic policy gradient (LSTM-DDPG),deep reinforcement learning (DRL)
AI 理解论文
溯源树
样例
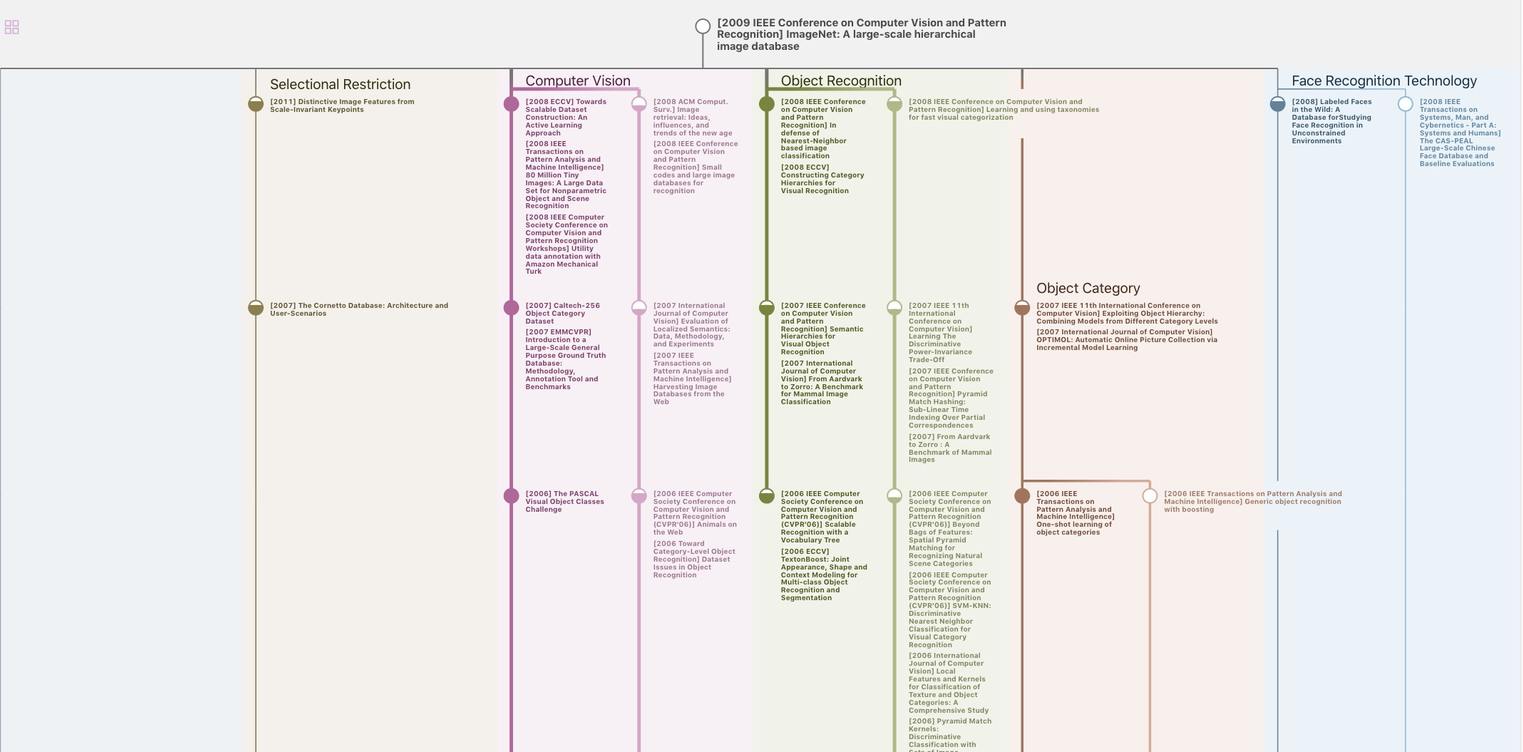
生成溯源树,研究论文发展脉络
Chat Paper
正在生成论文摘要