Deep Facial Phenotyping with Mixup Augmentation
MEDICAL IMAGE UNDERSTANDING AND ANALYSIS, MIUA 2023(2024)
摘要
The classification of genetic disorders from face images has the potential to assist with early diagnosis and effective treatment. A key objective in this field is to enhance the accuracy and robustness of deep learning models in classifying genetic disorders from face images for disorders that are not represented in the training data. In this paper, we propose the use of input mixup augmentation to improve few-shot classification performance on this task. Furthermore, we present a specialised version of mixup that warps together face images using face keypoints, and show that this improves performance further. The motivation for using keypoint guided mixup is to align face structure and produce an intermediate image between two disorders. We compare the performance of our proposed method with the baseline model and demonstrate significant improvements in accuracy, with a classification accuracy of 31.3% on the GMDB-Rare benchmark dataset. Our results show that incorporating input mixup augmentation and face keypoint-based mixup can enhance the ability of deep learning models to identify genetic disorders from face images, providing a promising approach for future research in this area.
更多查看译文
关键词
Genetic disorders,Phenotypes,Deep learning,Computer vision,Few-shot,Image classification,Representation learning
AI 理解论文
溯源树
样例
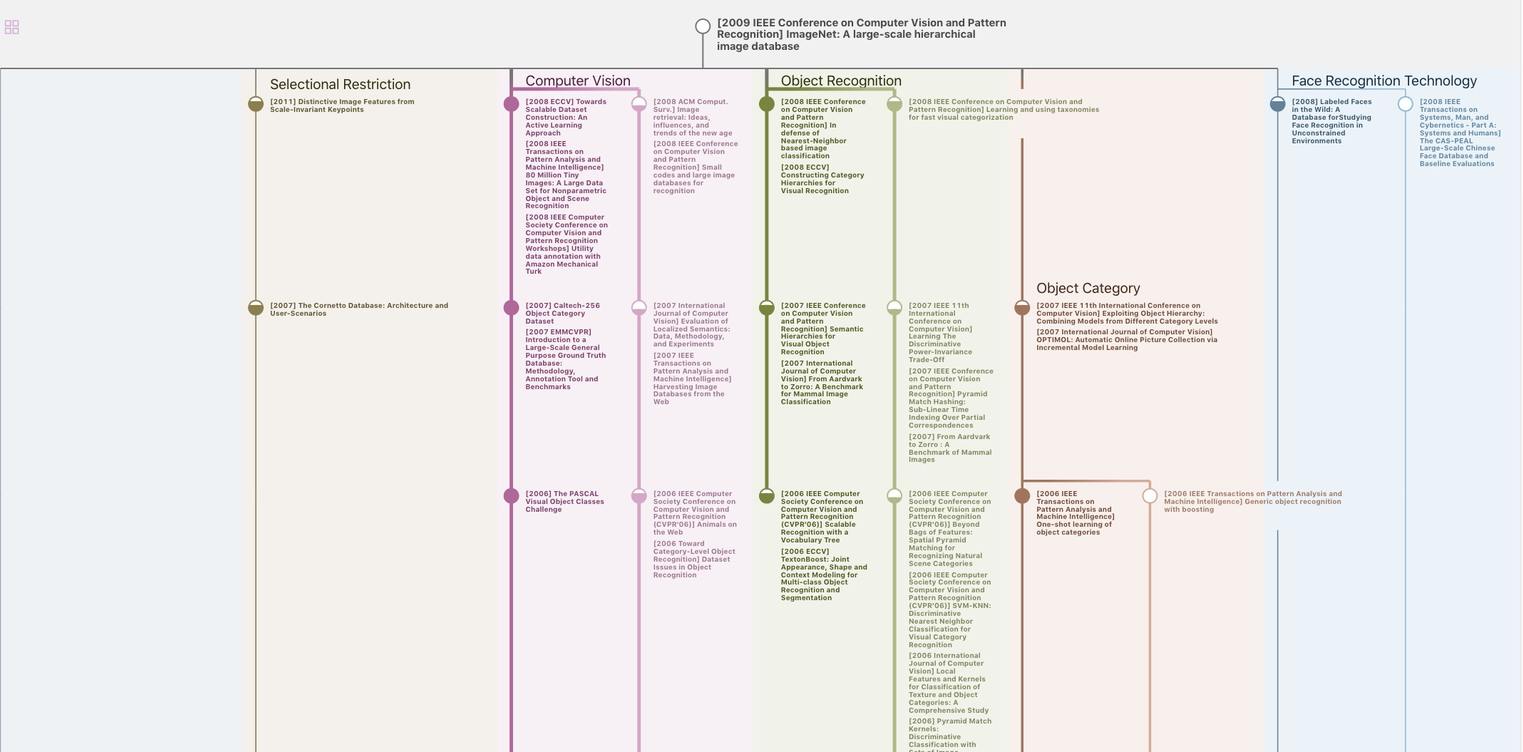
生成溯源树,研究论文发展脉络
Chat Paper
正在生成论文摘要