Classifying Emergency Patients into Fast-Track and Complex Cases Using Machine Learning
crossref(2023)
摘要
Abstract Background: Emergency medicine is a lifeline specialty at hospitals that patients head to for various reasons, including serious health problems, traumas, and adventitious conditions. Emergency departments are restricted to limited resources and personnel, which complicates handling all received cases optimally. Therefore, crowded waiting areas and long waiting durations result, which prompts some patients to leave before being examined. Methods: We utilized the databases (MIMIC-IV-ED and MIMIC-IV) to obtain records of patients who visited the Beth Israel Deaconess Medical Center in the USA. Triage and demographic data, dispositions, and length of stay of these individuals were extracted accordingly. Subsequently, the urgency of these cases was inferred based on standards stated in the literature and followed in developed countries, which are less than four-hour lengths of stay besides being discharged at the end of the emergency visit. Five classifying models were established by utilizing logistic regression, random forests, stochastic gradient boosting, classification and regression trees besides multi-layer perceptron algorithms. Results: A comparative framework using the five different machine learning algorithms was developed to classify these patients into two categories where the multi-layer perceptron model outperformed the others. Moreover, the relative importance of the outcome predictors was determined. Conclusions: This study suggests an approach to deal with non-urgent visits and lower overall waiting times at the emergency by utilizing the powers of machine learning to identify fast-track patients and discern them from critical cases.
更多查看译文
AI 理解论文
溯源树
样例
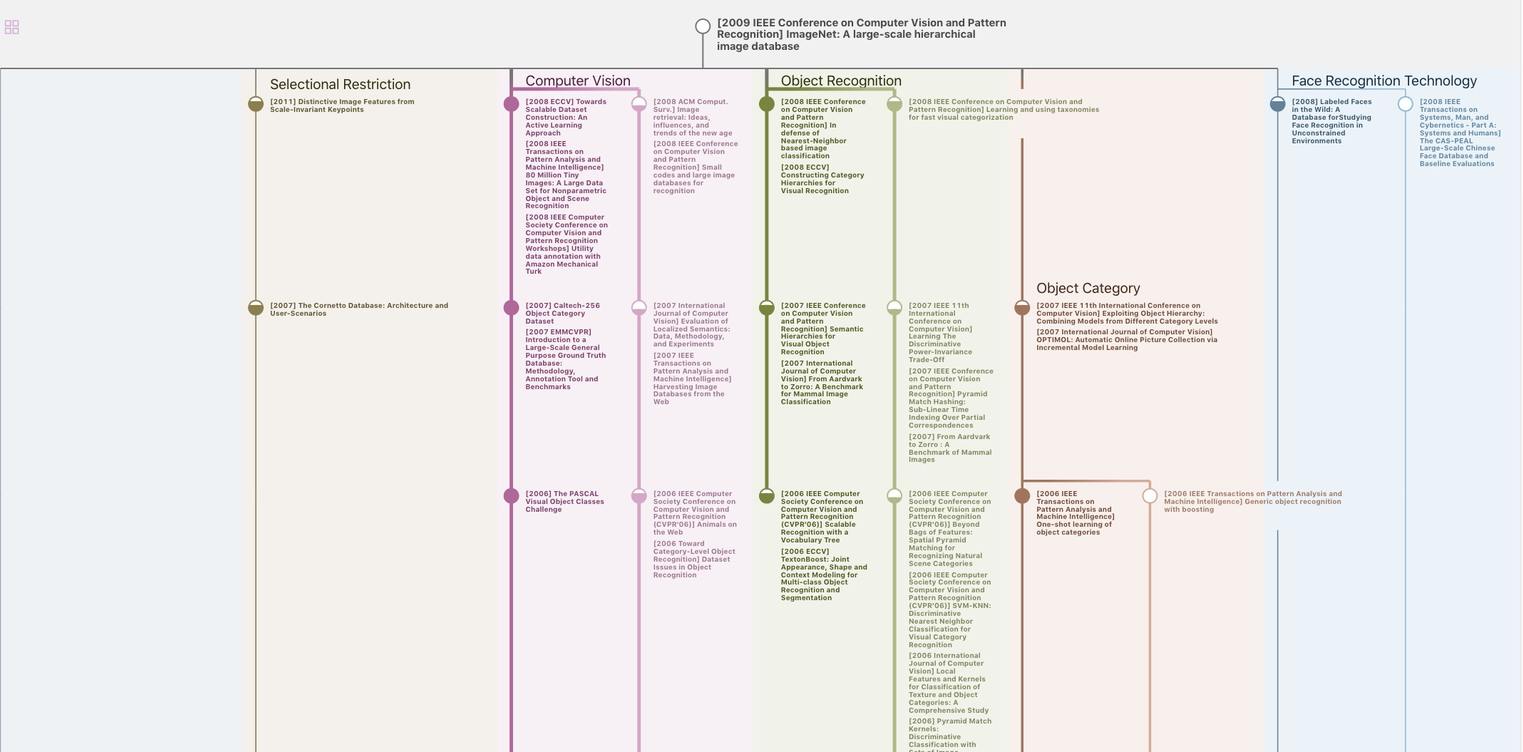
生成溯源树,研究论文发展脉络
Chat Paper
正在生成论文摘要