Extended nonnegative matrix factorization for dynamic functional connectivity analysis of fMRI data
Cognitive Neurodynamics(2023)
摘要
Dynamic functional connectivity (DFC) analysis using functional magnetic resonance imaging (fMRI) technology has attracted increasing attention in revealing brain dynamics in recent years. Although the nonnegative matrix factorization (NMF) method was applied to dynamic subgraph analysis to reveal brain dynamics, its application in DFC analysis was largely limited due to its nonnegative constraint on the input data. This study proposed the extended NMF (eNMF) method that allowed the input matrix and decomposed basis matrix to have negative values without altering the NMF algorithm. The eNMF method was applied to DFC analysis of both simulated and real resting fMRI data. The simulated data demonstrated that eNMF successfully decomposed the mixed-sign matrix into one positive matrix and one mixed-sign matrix. In contrast to K-means, eNMF extracted more accurate brain state patterns in all cases and estimated better DFC temporal properties for uneven brain state distribution. The real resting-fMRI data demonstrated that eNMF can provide more temporal measures of DFC and was more sensitive to detect intergroup differences of DFC than K-means. Results of eNMF revealed that the female group possibly showed worse relaxation and produced stronger spontaneous cognitive processes although they tended to spend more time in relaxation state and less time in states relevant to cognitive processes in contrast to the male group.
更多查看译文
关键词
NMF,fMRI,Dynamic functional connectivity,Brain states
AI 理解论文
溯源树
样例
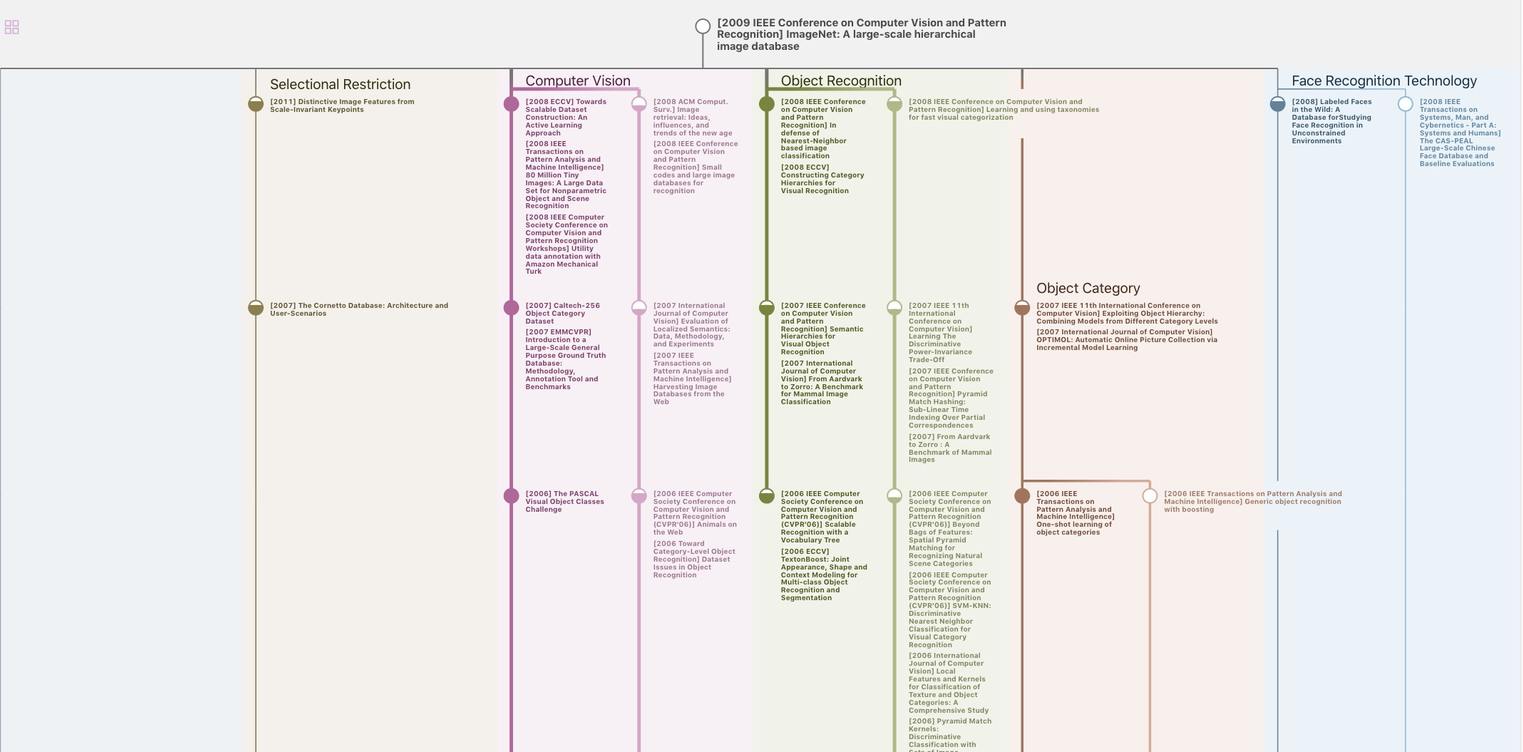
生成溯源树,研究论文发展脉络
Chat Paper
正在生成论文摘要