Optimal Sample Complexity of Contrastive Learning
ICLR 2024(2023)
摘要
Contrastive learning is a highly successful technique for learning representations of data from labeled tuples, specifying the distance relations within the tuple. We study the sample complexity of contrastive learning, i.e. the minimum number of labeled tuples sufficient for getting high generalization accuracy. We give tight bounds on the sample complexity in a variety of settings, focusing on arbitrary distance functions, both general $\ell_p$-distances, and tree metrics. Our main result is an (almost) optimal bound on the sample complexity of learning $\ell_p$-distances for integer $p$. For any $p \ge 1$ we show that $\tilde \Theta(\min(nd,n^2))$ labeled tuples are necessary and sufficient for learning $d$-dimensional representations of $n$-point datasets. Our results hold for an arbitrary distribution of the input samples and are based on giving the corresponding bounds on the Vapnik-Chervonenkis/Natarajan dimension of the associated problems. We further show that the theoretical bounds on sample complexity obtained via VC/Natarajan dimension can have strong predictive power for experimental results, in contrast with the folklore belief about a substantial gap between the statistical learning theory and the practice of deep learning.
更多查看译文
关键词
learning theory,sample complexity,vc dimension,contrastive learning,metric learning
AI 理解论文
溯源树
样例
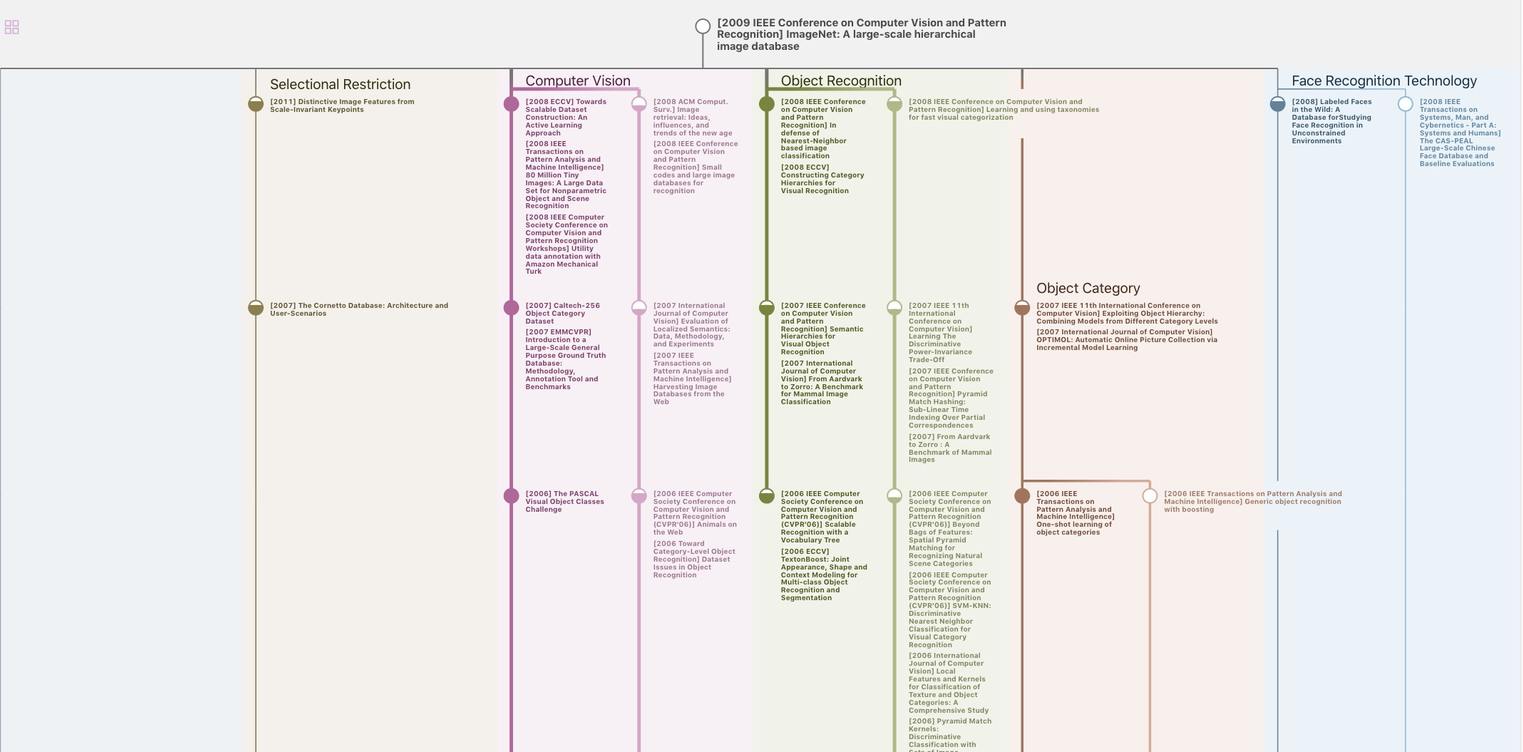
生成溯源树,研究论文发展脉络
Chat Paper
正在生成论文摘要