Feature-wise scaling and shifting: Improving the generalization capability of neural networks through capturing independent information of features
NEURAL NETWORKS(2024)
摘要
From the perspective of input features, information can be divided into independent information and correlation information. Current neural networks mainly concentrate on the capturing of correlation information through connection weight parameters supplemented by bias parameters. This paper introduces feature-wise scaling and shifting (FwSS) into neural networks for capturing independent information of features, and proposes a new neural network FwSSNet. In the network, a pair of scale and shift parameters is added before each input of each network layer, and bias is removed. The parameters are initialized as 1 and 0, respectively, and trained at separate learning rates, to guarantee the fully capturing of independence and correlation information. The learning rates of FwSS parameters depend on input data and the training speed ratios of adjacent FwSS and connection sublayers, meanwhile those of weight parameters remain unchanged as plain networks. Further, FwSS unifies the scaling and shifting operations in batch normalization (BN), and FwSSNet with BN is established through introducing a preprocessing layer. FwSS parameters except those in the last layer of the network can be simply trained at the same learning rate as weight parameters. Experiments show that FwSS is generally helpful in improving the generalization capability of both fully connected neural networks and deep convolutional neural networks, and FWSSNets achieve higher accuracies on UCI repository and CIFAR-10.
更多查看译文
关键词
Neural networks,Feature-wise scaling and shifting,Independent information,Correlation information,Generalization capability
AI 理解论文
溯源树
样例
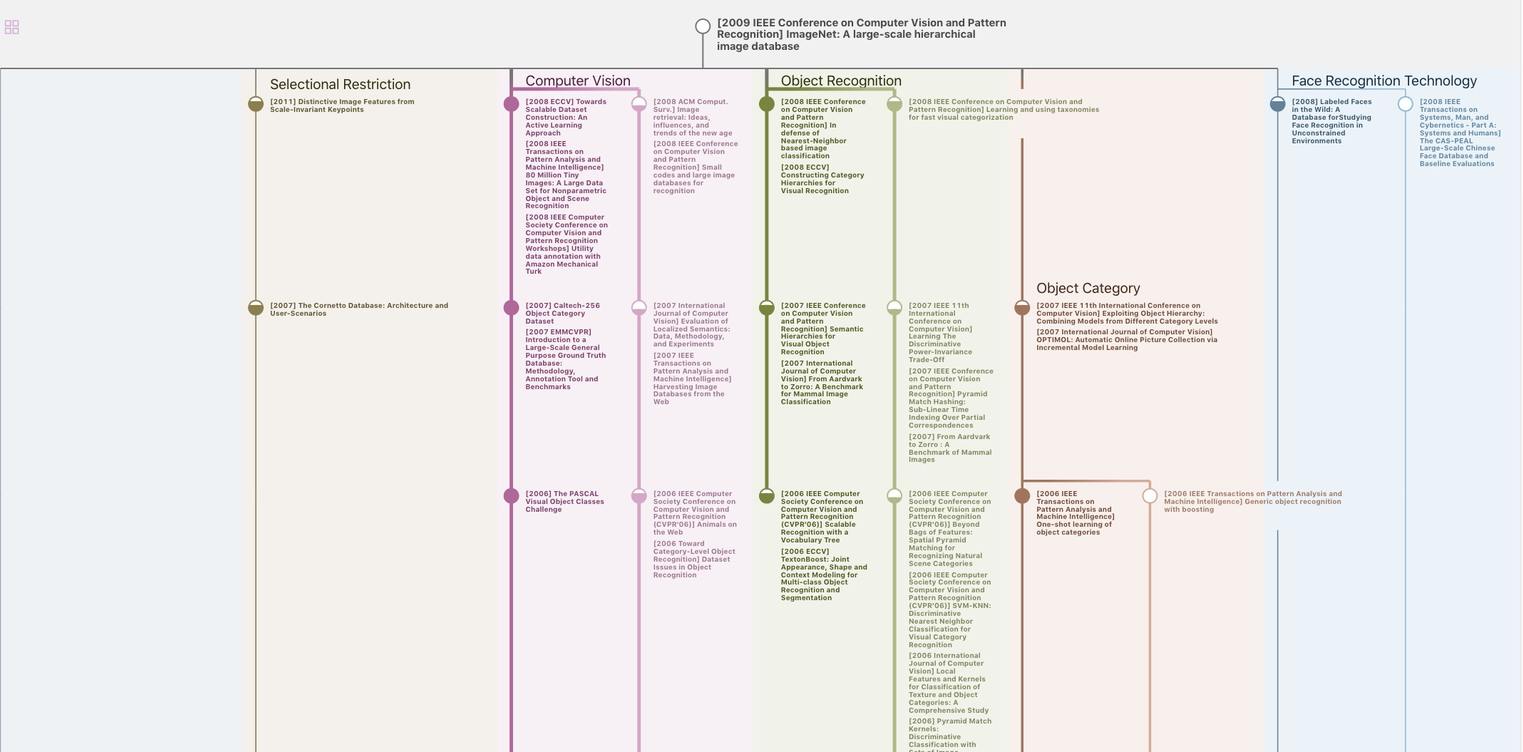
生成溯源树,研究论文发展脉络
Chat Paper
正在生成论文摘要