Shallow hierarchical CNN-LSTM for activity recognition to integrate postural transition states
2023 IEEE SENSORS(2023)
摘要
This paper presents an approach to enhance classification accuracy of Human Activity Recognition (HAR) datasets using a shallow hierarchical method, comprised of 2 Convolutional layers and 1 LSTM Layer, to identify transition tasks. The designed model was tested on the Human Activity and Postural Transition (HAPT) dataset from the University of California, Irvine, achieving an accuracy of 93%, demonstrating it's efficacy in correctly identifying a variety of human activities, including the postural transition states. An F1 score of 85% was attained, highlighting a reliable method of reducing false positives on an unbalanced dataset. The performance on these metrics showcases the model's reliability and proficiency in classifying activities within unbalanced datasets.
更多查看译文
关键词
Human Activity Recognition,CNN-LSTM,Hierarchical Networks,HAPT,Postural Transition
AI 理解论文
溯源树
样例
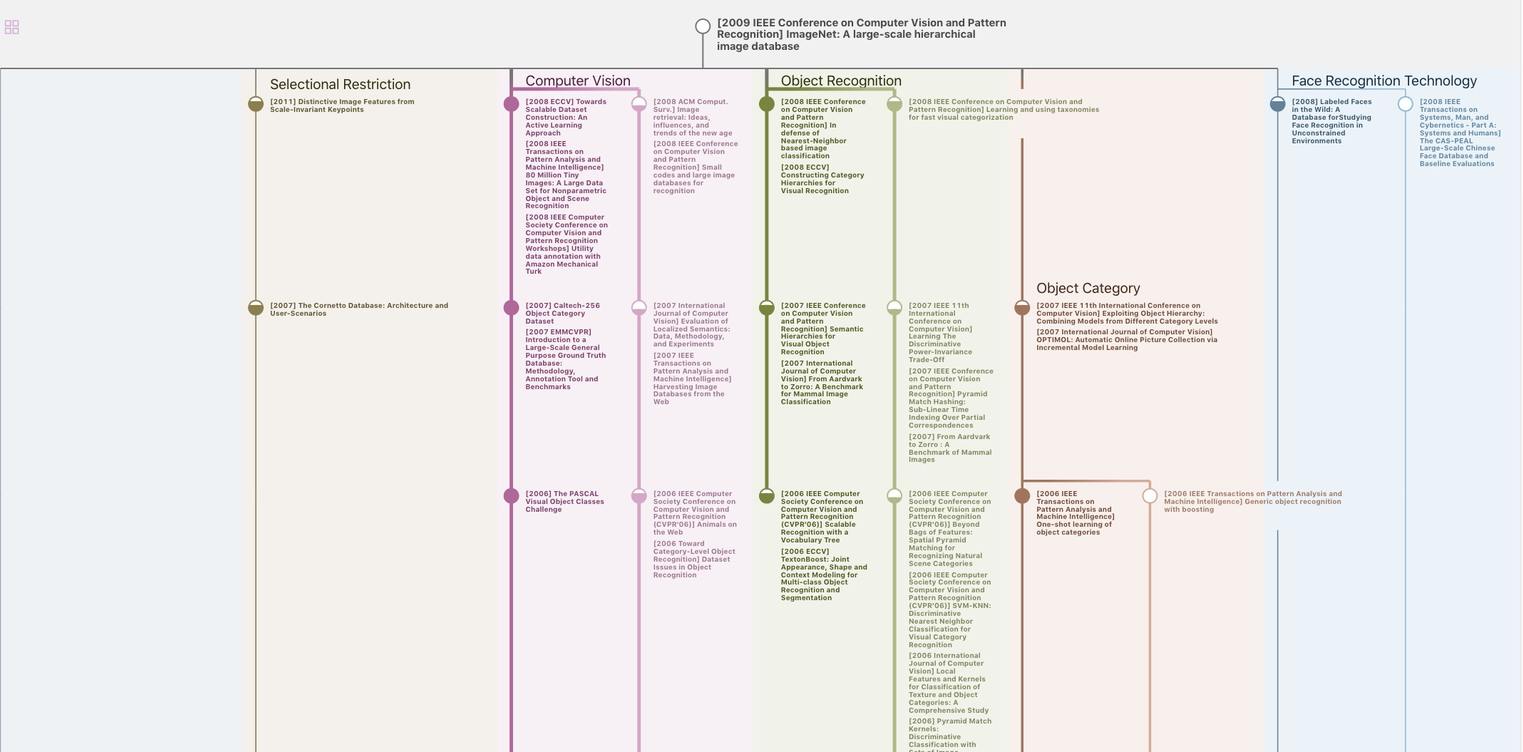
生成溯源树,研究论文发展脉络
Chat Paper
正在生成论文摘要