Performance Enhancement via Synaptic Plasticity in an Integrated Photonic Recurrent Neural Network with Phase-Change Materials
2023 Conference on Lasers and Electro-Optics Europe & European Quantum Electronics Conference (CLEO/Europe-EQEC)(2023)
摘要
Synaptic plasticity, i.e. the ability of synaptic connections to strengthen or weaken depending on their input, is a fundamental component of learning and memory in biological neural networks [1]. This property allows the network parameters to directly adapt to the input signal, thus without being externally tuned by a training algorithm. In contrast with this paradigm, the most popular and successful artificial neural network (ANN) models are nowadays based on backpropagation, which usually requires full observability of the network states and precise parameter tuning. In practice, these requirements strongly limit the scalability of neuromorphic hardware and backpropagation is not considered biologically plausible [2].
更多查看译文
关键词
ANN,artificial neural network,backpropagation,biological neural networks,integrated photonic recurrent neural network,network states,neuromorphic hardware,phase-change materials,precise parameter tuning,synaptic plasticity,training algorithm
AI 理解论文
溯源树
样例
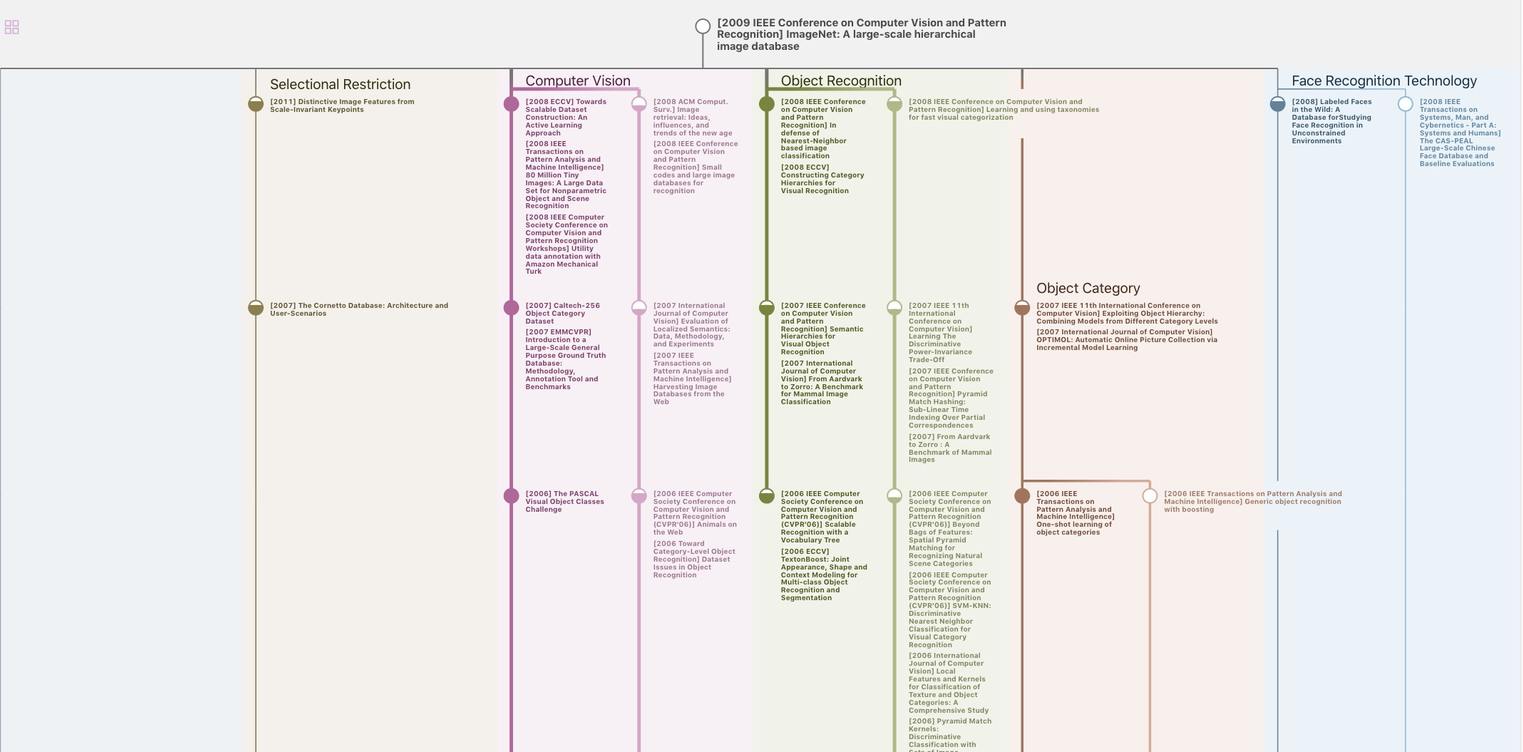
生成溯源树,研究论文发展脉络
Chat Paper
正在生成论文摘要