Deep Learning Based TEMPEST Attacks on a Quantum Key Distribution Sender
2023 Conference on Lasers and Electro-Optics Europe & European Quantum Electronics Conference (CLEO/Europe-EQEC)(2023)
摘要
While quantum key distribution (QKD) protocols are proven secure based on fundamental physical laws, side channels may allow an eavesdropper to obtain information unnoticeably. We present a profiled [1] side-channel attack using a deep convolutional neural network to analyze the classical, radio-frequency electromagnetic emissions from the electronics of a QKD sender. At a few centimeters from the device, we are able to recover virtually all information about the secret key. Furthermore, we can still observe traces of electromagnetic radiation from the device at distances of a few meters using a wideband antenna. Our methods are designed to be easily adaptable and may serve as a starting point for assessing the presence of this side channel for other devices.
更多查看译文
关键词
classical radio-frequency electromagnetic emissions,deep convolutional neural network,deep learning,electromagnetic radiation,fundamental physical laws,QKD sender,quantum key distribution protocols,quantum key distribution sender,secret key,side-channel attack,tEMPEST attacks
AI 理解论文
溯源树
样例
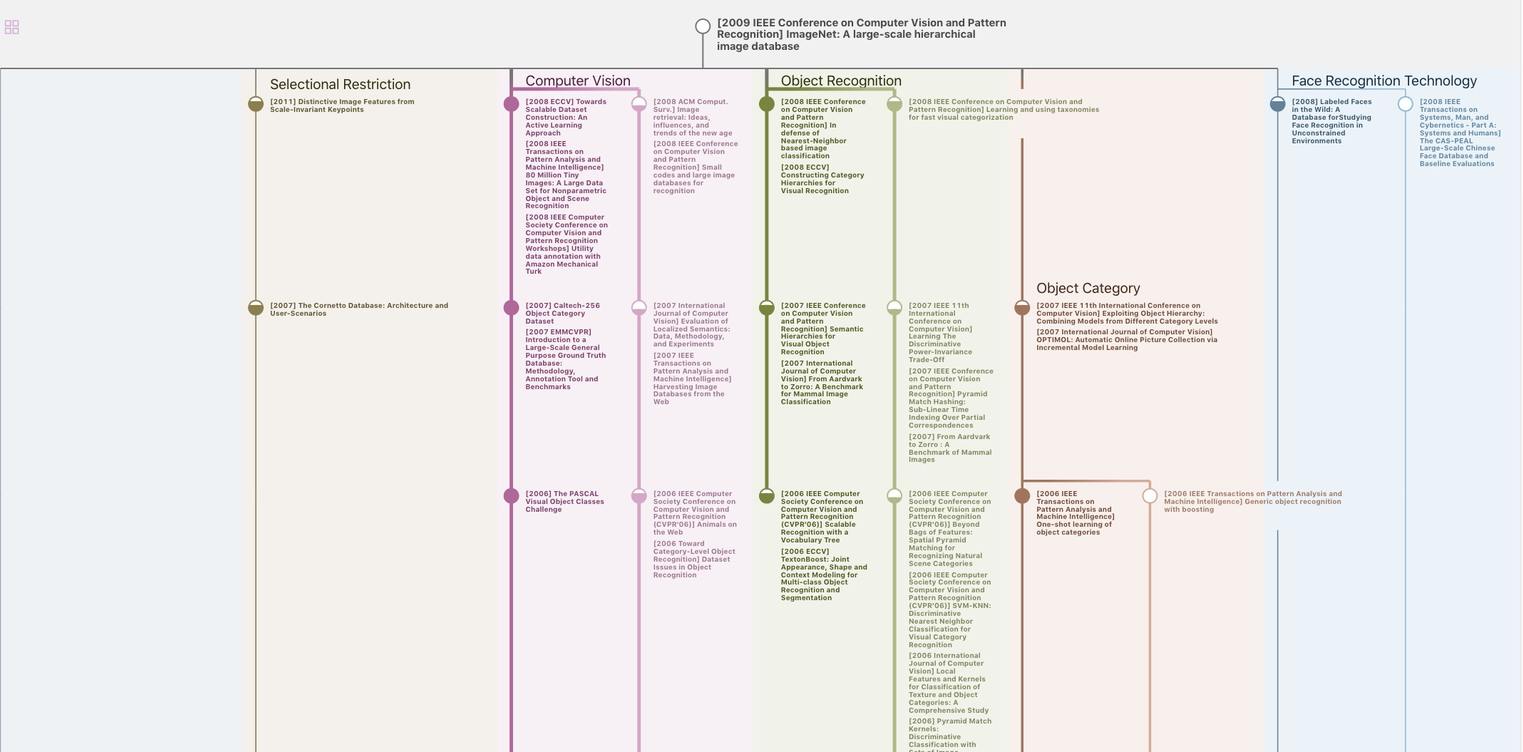
生成溯源树,研究论文发展脉络
Chat Paper
正在生成论文摘要