GAN-Based Interactive Reinforcement Learning from Demonstration and Human Evaluative Feedback
2023 IEEE INTERNATIONAL CONFERENCE ON ROBOTICS AND AUTOMATION, ICRA(2023)
摘要
Generative adversarial imitation learning (GAIL) - a general model-free imitation learning method, allows robots to directly learn policies from expert trajectories in large environments. However, GAIL shares the limitation of other imitation learning methods that they can seldom surpass the performance of demonstrations. In this paper, to address the limit of GAIL, we propose GAN-based interactive reinforcement learning (GAIRL) from demonstrations and human evaluative feedback, by combining the advantages of GAIL and interactive reinforcement learning. We test GAIRL in six physics-based control tasks, ranging from simple low-dimensional control tasks - Cart Pole, Mountain Car and Lunar Lander, to difficult high-dimensional tasks - Inverted Double Pendulum, Hopper and HalfCheetah. Our results suggest that, the GAIRL agent can generally surpass the performance of demonstrations in both low-dimensional and high-dimensional tasks and get an optimal or close to optimal policy.
更多查看译文
AI 理解论文
溯源树
样例
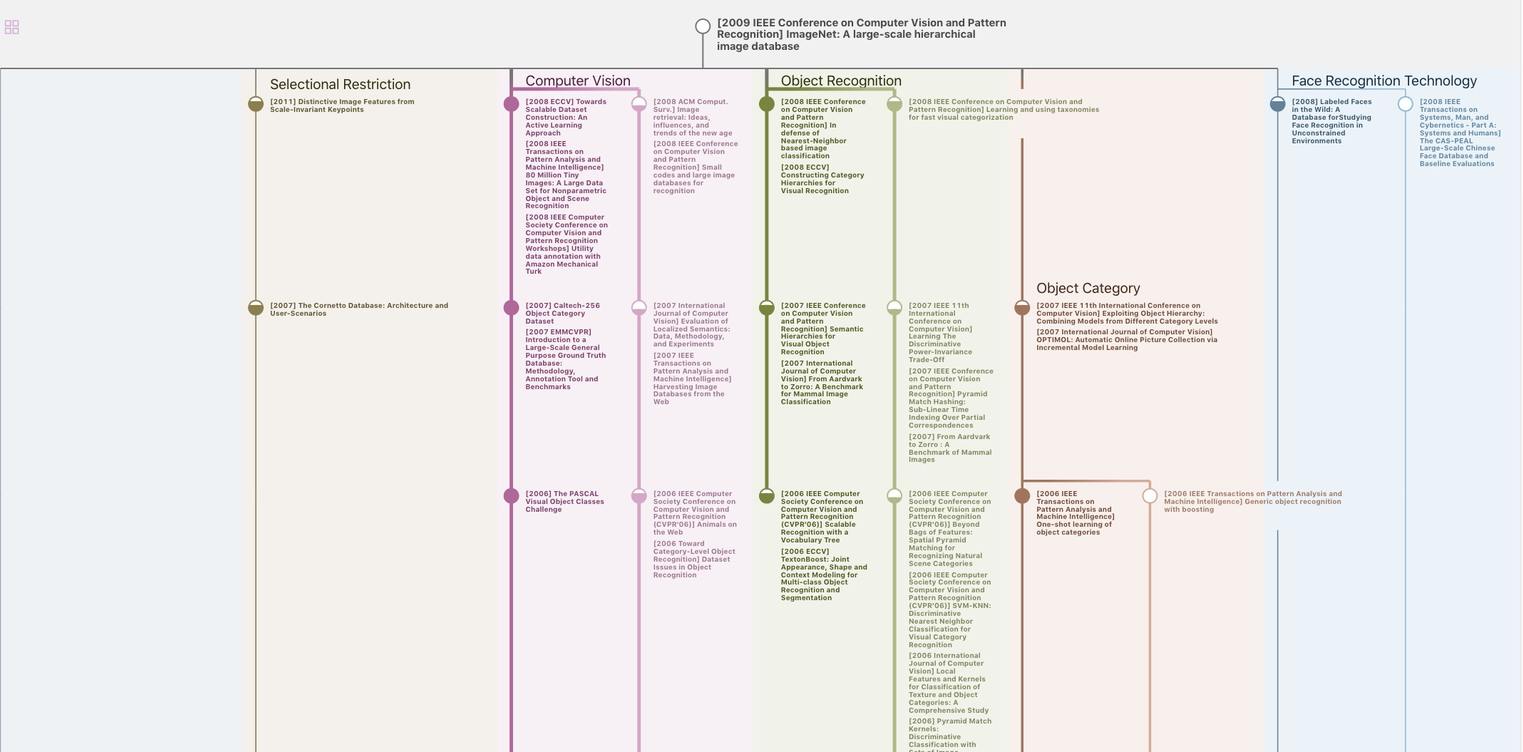
生成溯源树,研究论文发展脉络
Chat Paper
正在生成论文摘要