Toxicity Detection Methodology of Adverse Outcome Pathways using Physicochemical Properties and Machine Learning Approaches
2023 4th International Conference for Emerging Technology (INCET)(2023)
摘要
In order to quickly and effectively determine whether certain chemical compounds have the potential to interfere with bodily functions that could be harmful to a person's health. Authors have developed a prediction model for improved assessment of toxicity. Here, we have presented an in-silico computational method for the prediction of toxicity of small drug molecules using their various physicochemical characteristics (molecular descriptors) that can bind with various nuclear receptor (NR) and stress response (SR) signalling pathways. These signalling pathways are also known as adverse outcome pathways. The pharmaceutical data exploration laboratory (PaDEL) software is used for extracting the features of all drug molecules. Initially, the class imbalance is resolved using SMOTE algorithms for all the datasets and feature selection is performed by a correlation-based feature selection algorithm. It is found that the extended topochemical atom (ETA) descriptors, electro-topological state descriptors, Crippen's log P, and Molar refractivity (MR) are quite rich in chemical information to encode the structural features that contribute to the toxicities and these indices may be used in combination with other topological and physicochemical descriptors for the development of predictive QSAR model. We have proposed a methodology that can be applied on various datasets of adverse outcome pathways for the prediction of toxicity of small drug molecules. The accuracy that we have found by using our proposed methodology is outperformed in comparison to using the models in simple manners.
更多查看译文
关键词
machine learning,molecular descriptor,activity,toxicity,feature selection,nuclear receptor pathways,stress response pathways,class imbalance
AI 理解论文
溯源树
样例
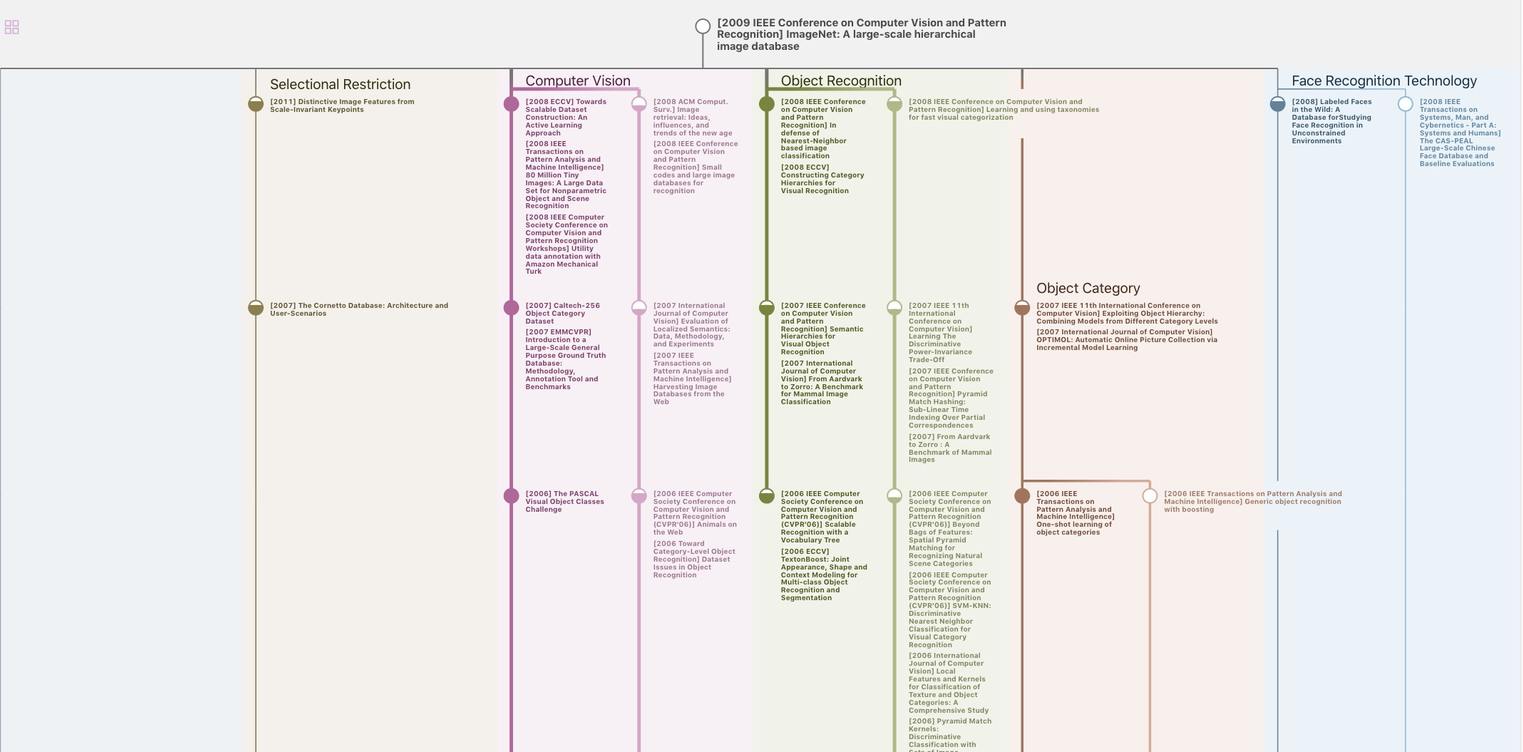
生成溯源树,研究论文发展脉络
Chat Paper
正在生成论文摘要