CARP-YOLO: A Detection Framework for Recognising and Counting Fish Species in a Cluttered Environment
2023 4th International Conference for Emerging Technology (INCET)(2023)
摘要
In the research area of object detection, the recognition of different fish species in a cluttered environment is a challenging task. In the live fish market, different fish species with variations in size, angle, and scale make it hard for the common people to recognize the species properly. Again, counting and sorting fish species is an important task in the fisheries industry. A dataset named JUDVLP-WBUAFS: Fishdb-Detection.v1 with 400 images is prepared by collecting images from the different live fish markets in West Bengal under unconstrained environments. Various augmentations like flip, rotation, blur, gaussian noise, hue saturation, and RGBshift have been applied to make the dataset more diversified and less prone to overfitting. A total of six fish species, Labeo catla, Labeo rohita, Cirrhinus mrigala, Labeo bata, Hypophthalmichthys molitrix, and Ctenopharyngodon idella, are considered in this study for the purposes of recognition and counting. Two popular object detection deep learning networks, YOLOv3 and YOLOv5, with different variants, have been applied to the original and augmented datasets individually. Using YOLOv5l and the YOLOv3-SPP network, the best mAP@0.5 of 0.764 is achieved on the original dataset. In the augmented dataset, the best mAP@0.5 of 0.84 is achieved using the YOLOv3-SPP network. The mAP@0.5 value on both datasets shows a promising result for the recognition of fish species in some extremely cluttered environments. This study is expected to help common people and the fishing industry in a variety of contexts.
更多查看译文
关键词
Fish recognition,counting fish species,Object detection
AI 理解论文
溯源树
样例
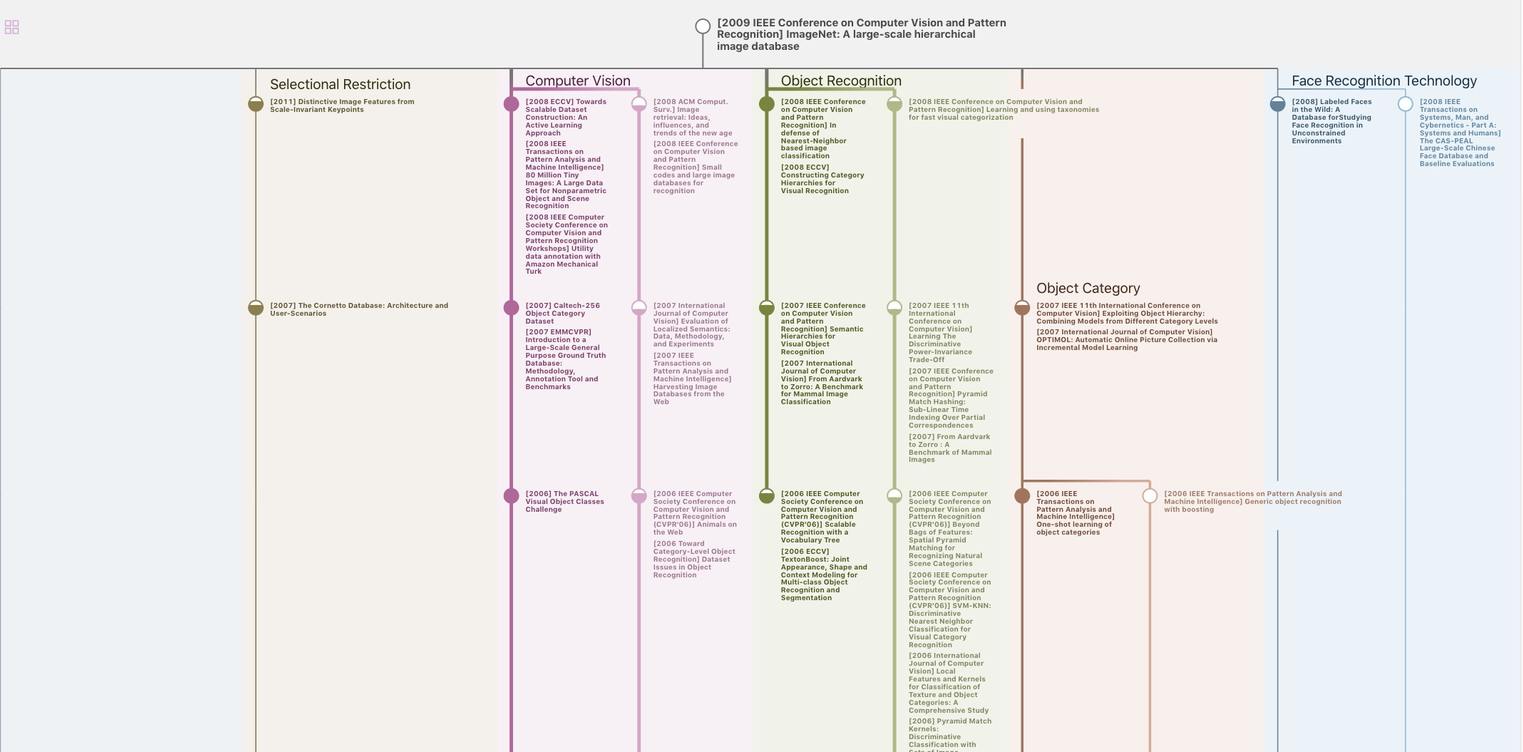
生成溯源树,研究论文发展脉络
Chat Paper
正在生成论文摘要